Privacy-Preserving Online Content Moderation with Federated Learning
COMPANION OF THE WORLD WIDE WEB CONFERENCE, WWW 2023(2023)
摘要
Users are exposed to a large volume of harmful content that appears daily on various social network platforms. One solution to users' protection is developing online moderation tools using Machine Learning (ML) techniques for automatic detection or content filtering. On the other hand, the processing of user data requires compliance with privacy policies. This paper proposes a privacy-preserving Federated Learning (FL) framework for online content moderation that incorporates Central Differential Privacy (CDP). We simulate the FL training of a classifier for detecting tweets with harmful content, and we show that the performance of the FL framework can be close to the centralized approach. Moreover, it has a high performance even if a small number of clients (each with a small number of tweets) are available for the FL training. When reducing the number of clients (from fifty to ten) or the tweets per client (from 1K to 100), the classifier can still achieve AUC. Furthermore, we extend the evaluation to four other Twitter datasets that capture different types of user misbehavior and still obtain a promising performance (61% - 80% AUC).
更多查看译文
关键词
content moderation,federated learning,privacy
AI 理解论文
溯源树
样例
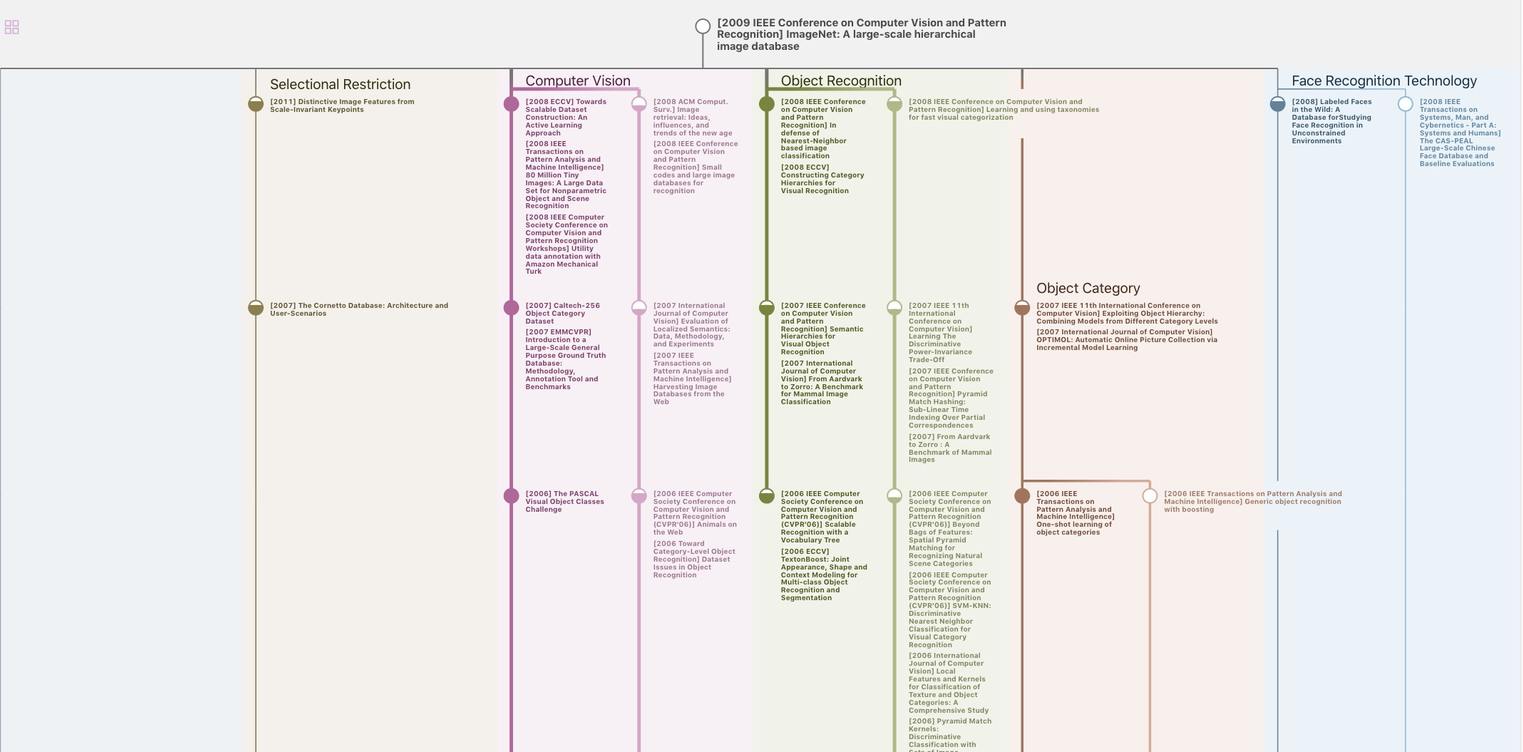
生成溯源树,研究论文发展脉络
Chat Paper
正在生成论文摘要