Optimization of fast-charging strategies for lithium-ion batteries with Kriging-Assisted evolution
International Journal of Energy Research(2022)
摘要
Reducing the charging period while maximizing battery cycle life is a major objective in the development of fast charging technologies. However, the optimization of fast-charging strategies and evaluation of lifetime necessitate substantial time-consuming experiments. To decrease both the time and number of tests needed, we propose an experimental learning optimization method to efficiently optimize the parameter space of 10-min, five-step fast-charging strategies. Two components are combined to minimize the optimization cost. On the one hand, the cycle life is evaluated by a deep learning method. It only requires 10 cycles of charging data to predict the cycle life with high accuracy, so can reduce lifetime experiments by over 99%. On the other hand, the optimization of the charging strategy is experimentally carried out based on the Kriging-assisted population evolution algorithm (KAPEA). It is employed for the first time in this field to select promising strategies for experiments and narrow down the experimental search space remarkably. And the procedure period can be significantly saved, 98.7% less than with conventional approaches. Furthermore, validated by experiments, the battery's cycle life under the strategy recommended by our method is the highest among all the strategies evaluated. Highlights A method of optimizing a 10-min fast-charging strategy is presented. A HPRCNN method is introduced to reduce the battery cycle life test time. The KAPEA approach is designed to reduce the test numbers of charging strategies. The test period is compressed greatly, 98.7% less than with conventional methods. A fast-charging strategy for batteries is obtained with the best cycle life.
更多查看译文
关键词
cycle life prediction, fast-charging strategy, kriging-assisted evolution algorithm, Lithium-ion battery
AI 理解论文
溯源树
样例
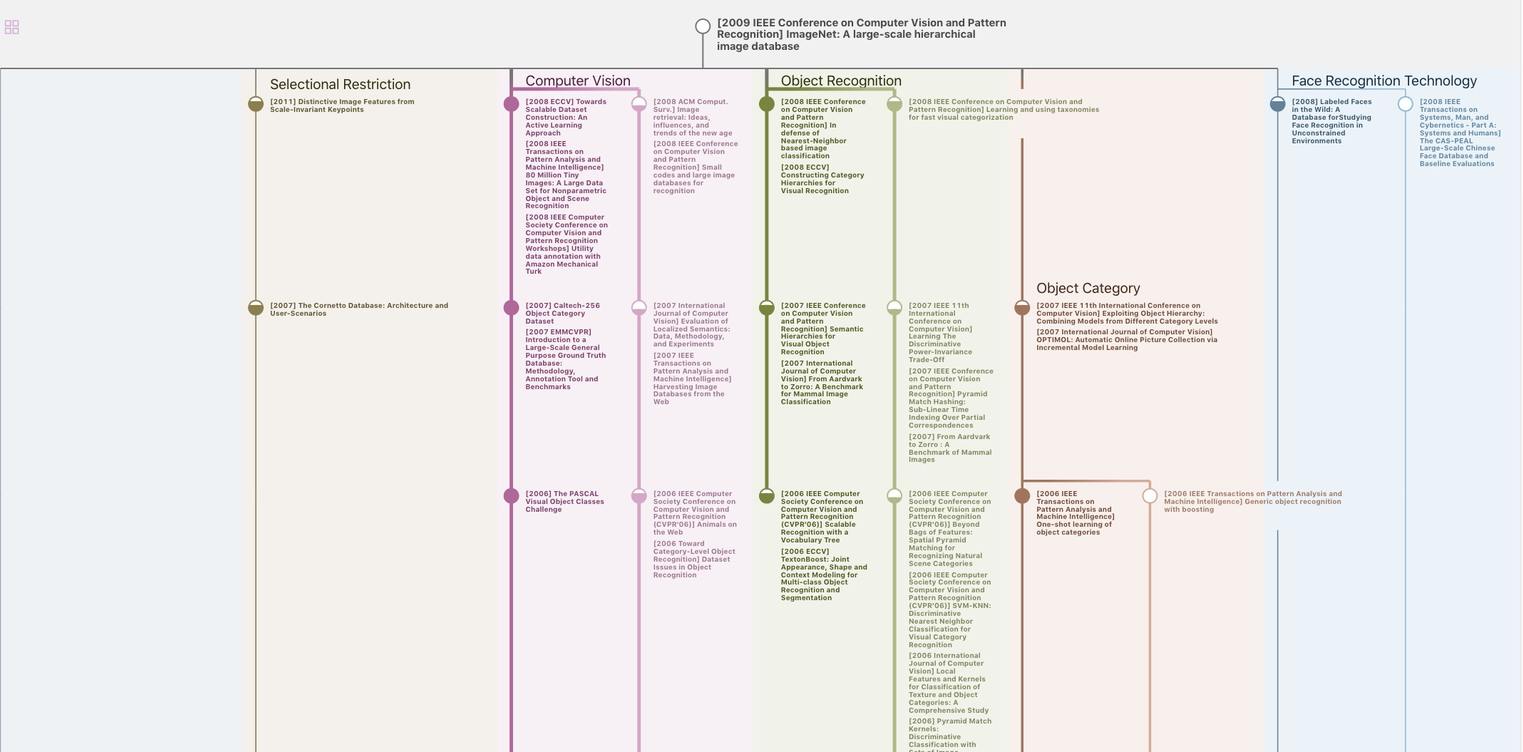
生成溯源树,研究论文发展脉络
Chat Paper
正在生成论文摘要