Simultaneous Depth Estimation and Surgical Tool Segmentation in Laparoscopic Images
IEEE Transactions on Medical Robotics and Bionics(2022)
Abstract
Surgical instrument segmentation and depth estimation are crucial steps to improve autonomy in robotic surgery. Most recent works treat these problems separately, making the deployment challenging. In this paper, we propose a unified framework for depth estimation and surgical tool segmentation in laparoscopic images. The network has an encoder-decoder architecture and comprises two branches for simultaneously performing depth estimation and segmentation. To train the network end to end, we propose a new multi-task loss function that effectively learns to estimate depth in an unsupervised manner, while requiring only semi-ground truth for surgical tool segmentation. We conducted extensive experiments on different datasets to validate these findings. The results showed that the end-to-end network successfully improved the state-of-the-art for both tasks while reducing the complexity during their deployment.
MoreTranslated text
Key words
Deep learning,self-supervised depth estimation,surgical instrument segmentation,multi-task learning
AI Read Science
Must-Reading Tree
Example
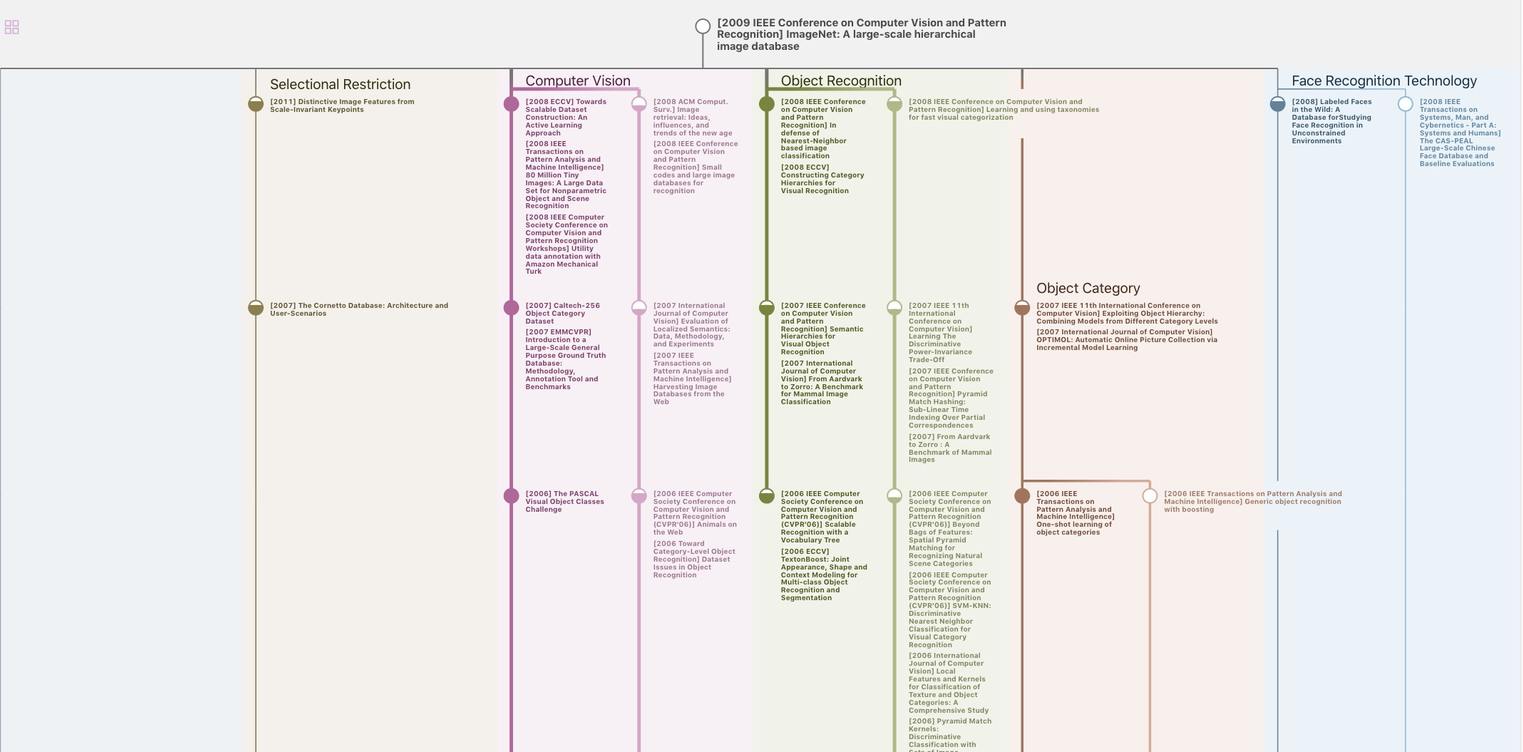
Generate MRT to find the research sequence of this paper
Chat Paper
Summary is being generated by the instructions you defined