Uncertainty Quantification of Kinetic Models Using Adjoint-Driven Active Subspace Algorithms
PROCEEDINGS OF THE COMBUSTION INSTITUTE(2023)
Key words
Kinetic models,Uncertainty quantification,Active subspaces,Adjoint-based methods
AI Read Science
Must-Reading Tree
Example
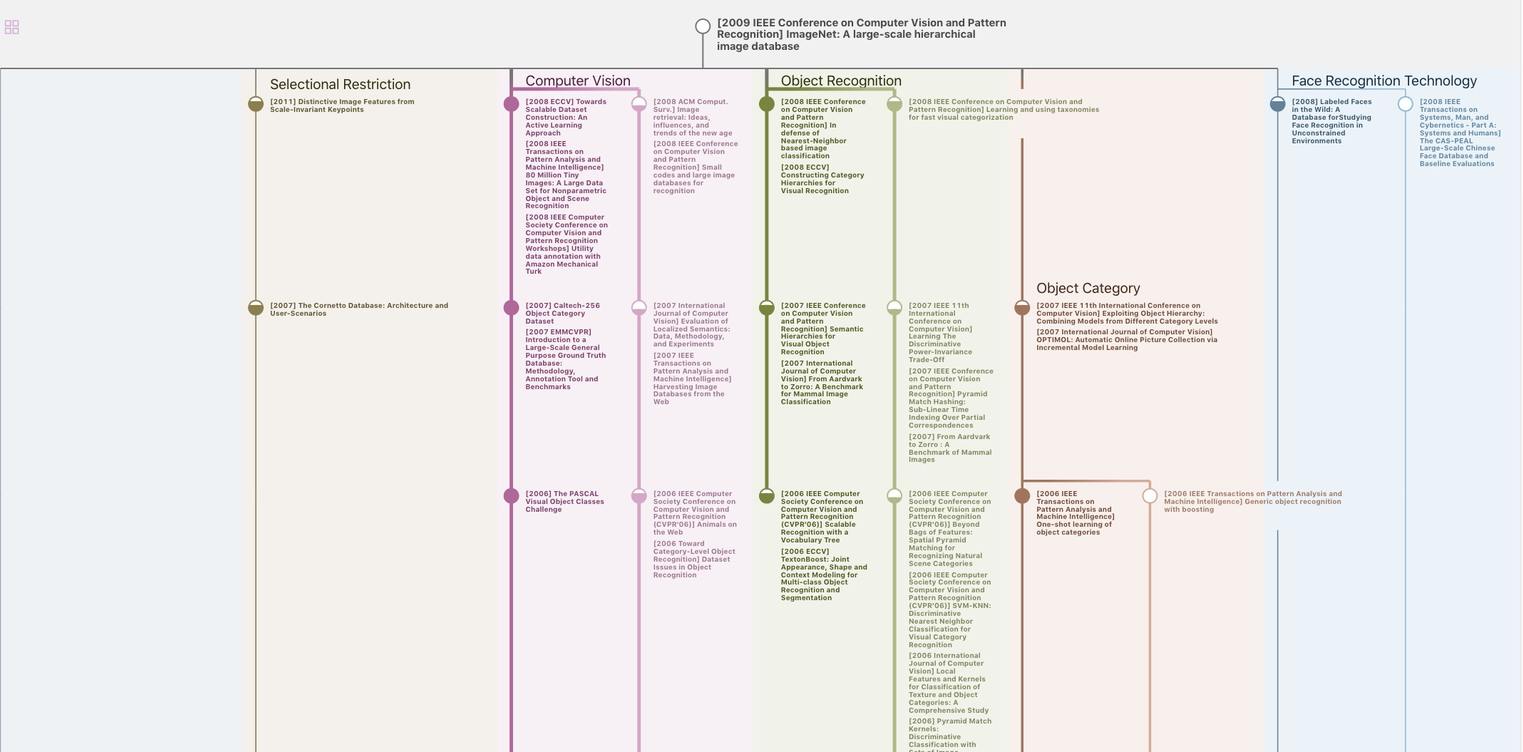
Generate MRT to find the research sequence of this paper
Chat Paper
Summary is being generated by the instructions you defined