Turning Normalizing Flows into Monge Maps with Geodesic Gaussian Preserving Flows
arXiv (Cornell University)(2023)
摘要
Normalizing Flows (NF) are powerful likelihood-based generative models that are able to trade off between expressivity and tractability to model complex densities. A now well established research avenue leverages optimal transport (OT) and looks for Monge maps, i.e. models with minimal effort between the source and target distributions. This paper introduces a method based on Brenier's polar factorization theorem to transform any trained NF into a more OT-efficient version without changing the final density. We do so by learning a rearrangement of the source (Gaussian) distribution that minimizes the OT cost between the source and the final density. We further constrain the path leading to the estimated Monge map to lie on a geodesic in the space of volume-preserving diffeomorphisms thanks to Euler's equations. The proposed method leads to smooth flows with reduced OT cost for several existing models without affecting the model performance.
更多查看译文
关键词
geodesic gaussian preserving flows,normalizing flows,monge maps
AI 理解论文
溯源树
样例
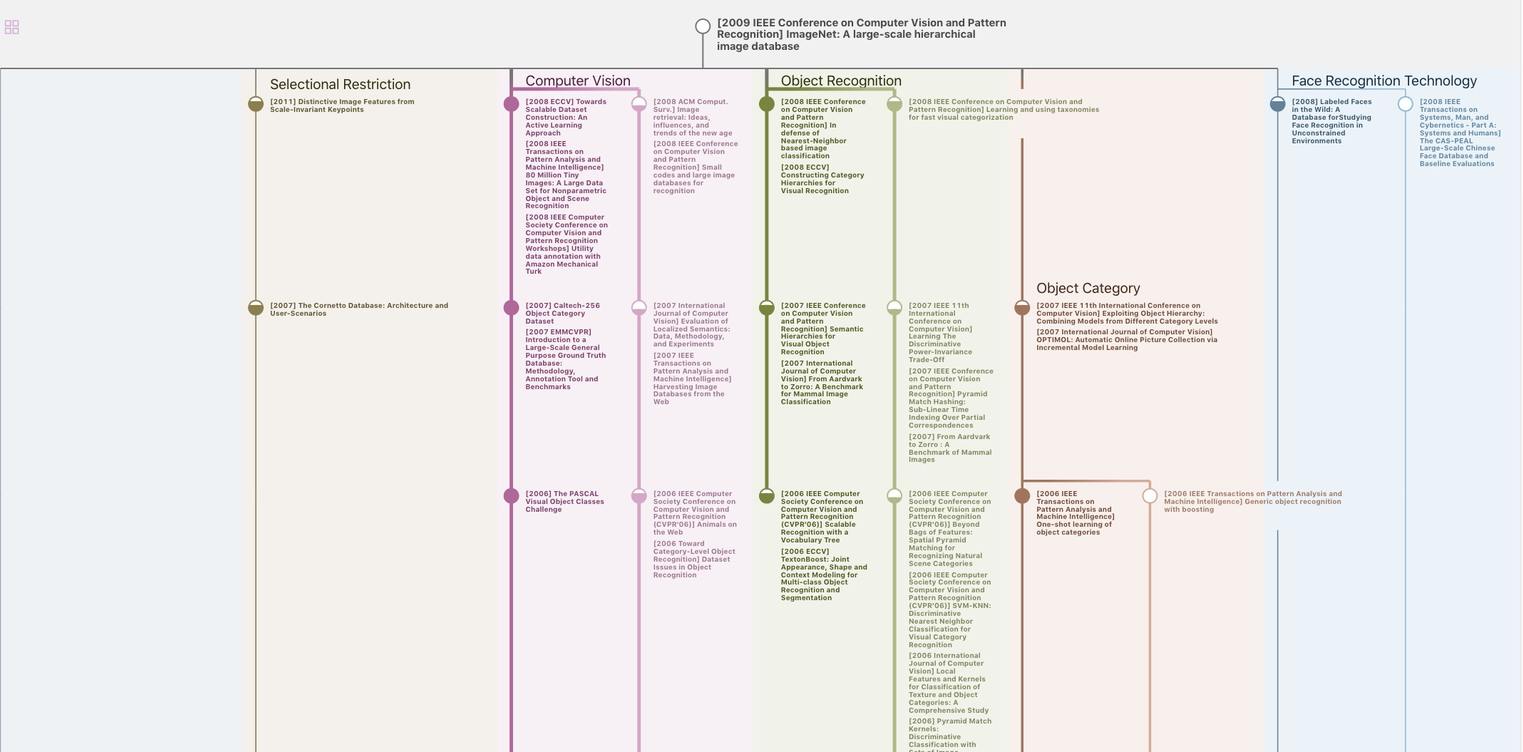
生成溯源树,研究论文发展脉络
Chat Paper
正在生成论文摘要