A Spatial-channel-temporal-fused Attention for Spiking Neural Networks
IEEE transactions on neural networks and learning systems(2024)
关键词
Neurons,Visualization,Biological system modeling,Training,Computational modeling,Membrane potentials,Biological neural networks,Event streams,predictive attentional remapping,spatial-channel-temporal-fused attention (SCTFA),spiking neural networks (SNNs),visual attention
AI 理解论文
溯源树
样例
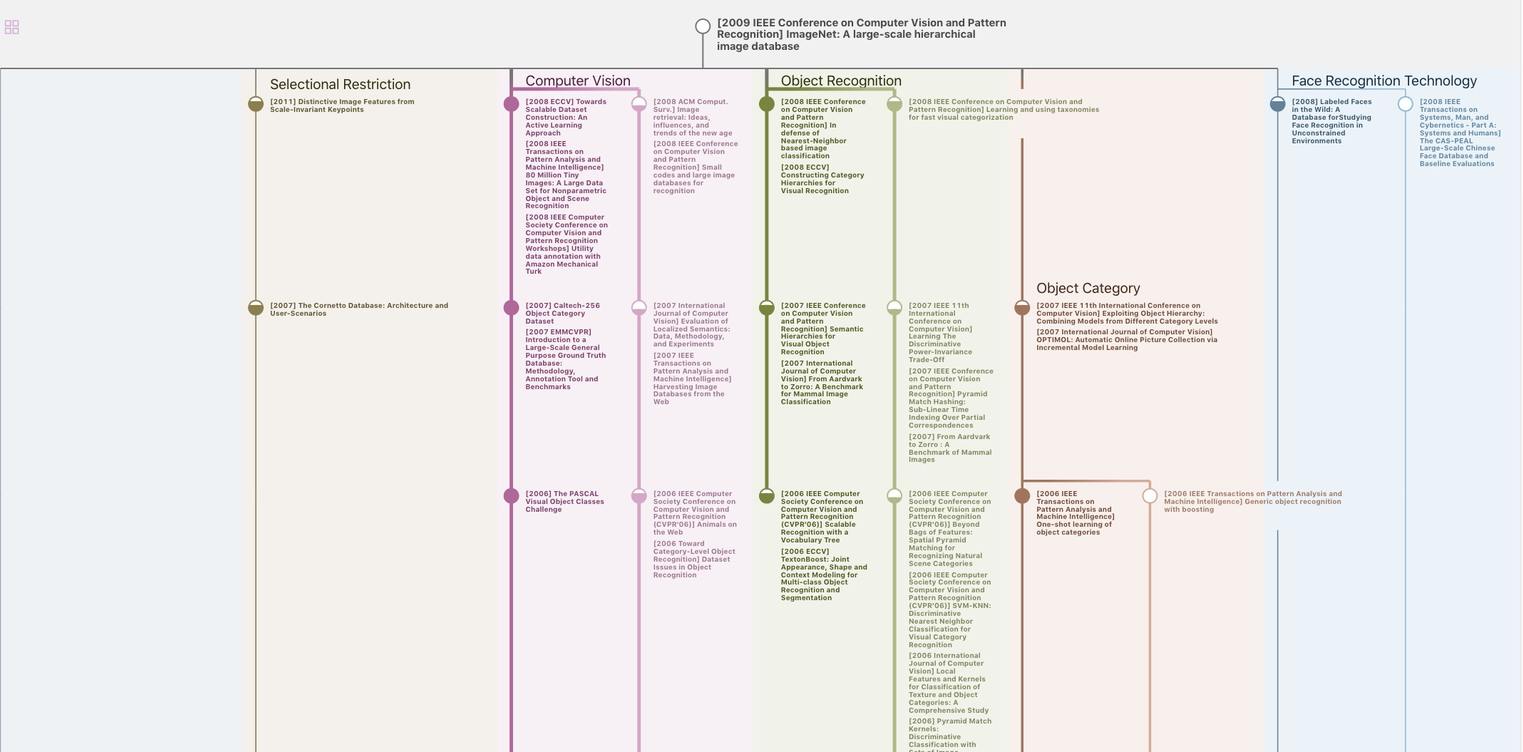
生成溯源树,研究论文发展脉络
Chat Paper
正在生成论文摘要