Towards Robots that Influence Humans over Long-Term Interaction
arxiv(2022)
摘要
When humans interact with robots influence is inevitable. Consider an autonomous car driving near a human: the speed and steering of the autonomous car will affect how the human drives. Prior works have developed frameworks that enable robots to influence humans towards desired behaviors. But while these approaches are effective in the short-term (i.e., the first few human-robot interactions), here we explore long-term influence (i.e., repeated interactions between the same human and robot). Our central insight is that humans are dynamic: people adapt to robots, and behaviors which are influential now may fall short once the human learns to anticipate the robot's actions. With this insight, we experimentally demonstrate that a prevalent game-theoretic formalism for generating influential robot behaviors becomes less effective over repeated interactions. Next, we propose three modifications to Stackelberg games that make the robot's policy both influential and unpredictable. We finally test these modifications across simulations and user studies: our results suggest that robots which purposely make their actions harder to anticipate are better able to maintain influence over long-term interaction. See videos here: https://youtu.be/ydO83cgjZ2Q
更多查看译文
关键词
autonomous car,desired behaviors,human drives,human learns,human-robot interactions,humans interact,influence humans,influential robot,long-term influence,long-term interaction,repeated interactions,robots influence,towards robots
AI 理解论文
溯源树
样例
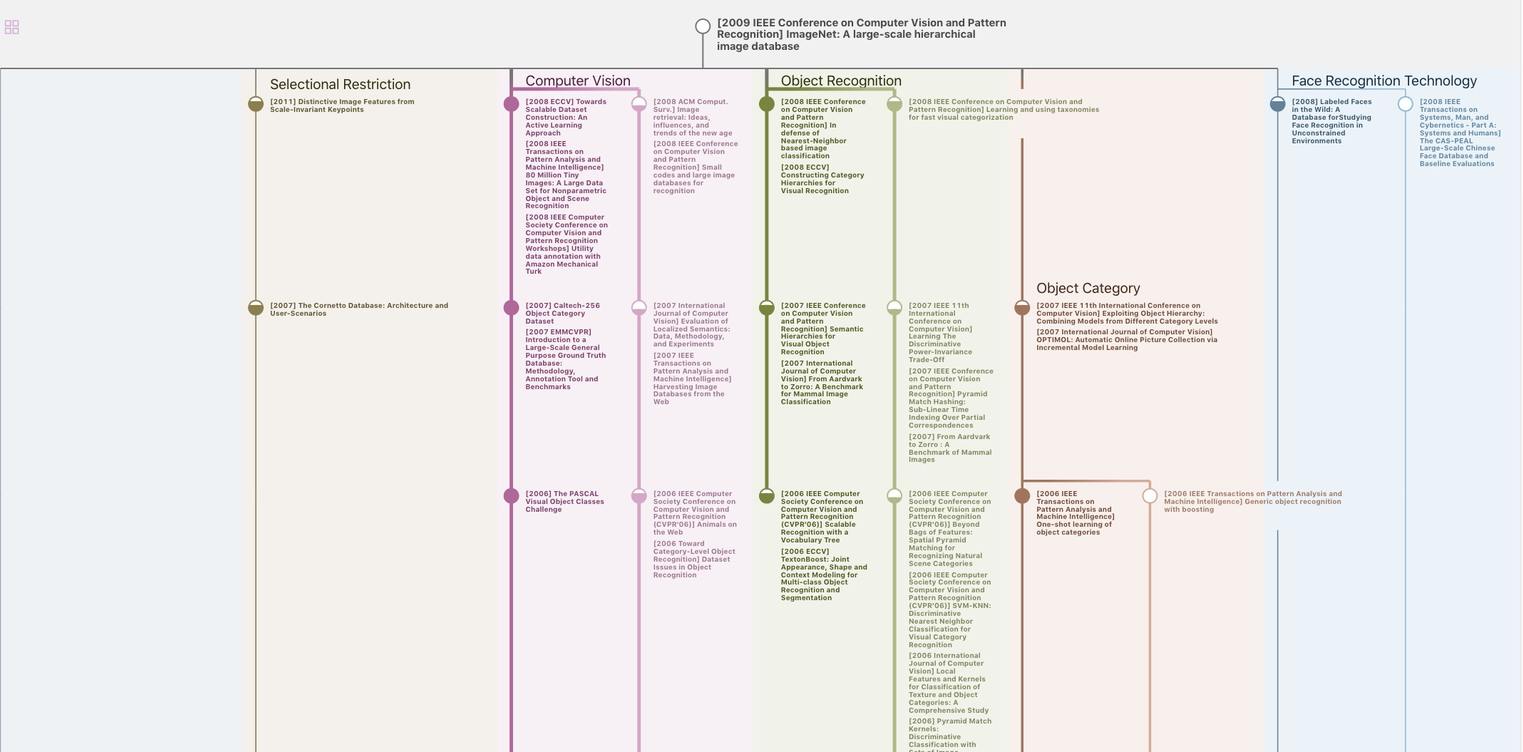
生成溯源树,研究论文发展脉络
Chat Paper
正在生成论文摘要