DRVN (Deep Random Vortex Network): A new physics-informed machine learning method for simulating and inferring incompressible fluid flows
Physics of Fluids(2022)
摘要
We present the deep random vortex network (DRVN), a novel physics-informed framework for simulating and inferring the fluid dynamics governed by the incompressible Navier--Stokes equations. Unlike the existing physics-informed neural network (PINN), which embeds physical and geometry information through the residual of equations and boundary data, DRVN automatically embeds this information into neural networks through neural random vortex dynamics equivalent to the Navier--Stokes equation. Specifically, the neural random vortex dynamics motivates a Monte Carlo-based loss function for training neural networks, which avoids the calculation of derivatives through auto-differentiation. Therefore, DRVN can efficiently solve Navier--Stokes equations with non-differentiable initial conditions and fractional operators. Furthermore, DRVN naturally embeds the boundary conditions into the kernel function of the neural random vortex dynamics and thus, does not need additional data to obtain boundary information. We conduct experiments on forward and inverse problems with incompressible Navier--Stokes equations. The proposed method achieves accurate results when simulating and when inferring Navier--Stokes equations. For situations that include singular initial conditions and agnostic boundary data, DRVN significantly outperforms the existing PINN method. Furthermore, compared with the conventional adjoint method when solving inverse problems, DRVN achieves a 2 orders of magnitude improvement for the training time with significantly precise estimates.
更多查看译文
关键词
deep random vortex network,flows,drvn,learning,physics-informed
AI 理解论文
溯源树
样例
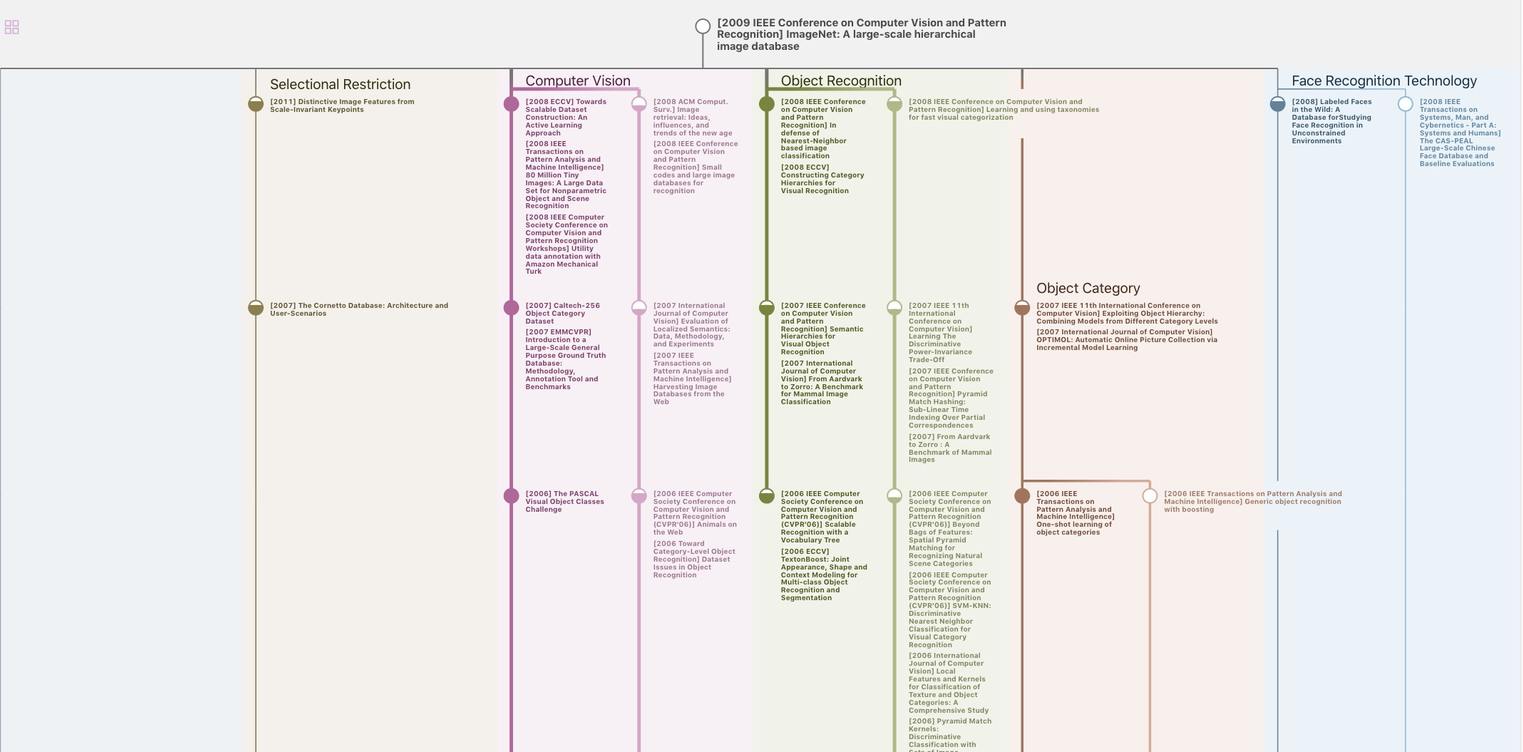
生成溯源树,研究论文发展脉络
Chat Paper
正在生成论文摘要