Selective Layer Tuning and Performance Study of Pre-Trained Models Using Genetic Algorithm
ELECTRONICS(2022)
摘要
Utilizing pre-trained models involves fully or partially using pre-trained parameters as initialization. In general, configuring a pre-trained model demands practitioners' knowledge about problems or an exhaustive trial-error experiment according to a given task. In this paper, we propose tuning trainable layers using a genetic algorithm on a pre-trained model that is fine-tuned on single-channel image datasets for a classification task. The single-channel dataset comprises images from grayscale and preprocessed audio signals transformed into a log-Mel spectrogram. Four deep-learning models used in the experimental evaluation employed the pre-trained model with the ImageNet dataset. The proposed genetic algorithm was applied to find the highest fitness for every generation to determine the selective layer tuning of the pre-trained models. Compared to the conventional fine-tuning method and random layer search, our proposed selective layer search with a genetic algorithm achieves higher accuracy, on average, by 9.7% and 1.88% (MNIST-Fashion), 1.31% and 1.14% (UrbanSound8k), and 2.2% and 0.29% (HospitalAlarmSound), respectively. In addition, our searching method can naturally be applied to various datasets of the same task without prior knowledge about the dataset of interest.
更多查看译文
关键词
deep learning,selective layer tuning,genetic algorithm,pre-trained model
AI 理解论文
溯源树
样例
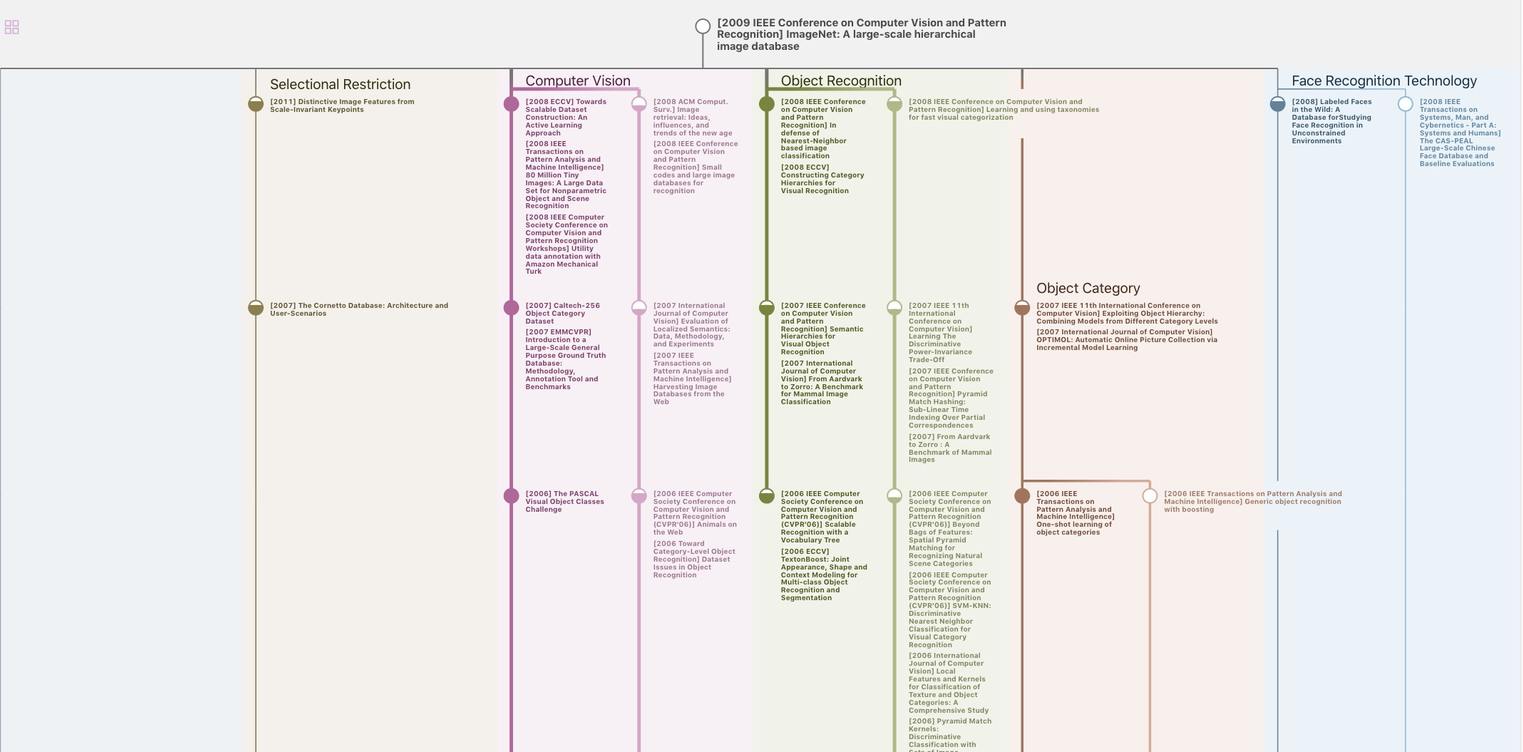
生成溯源树,研究论文发展脉络
Chat Paper
正在生成论文摘要