Harnessing deep reinforcement learning to construct time-dependent optimal fields for quantum control dynamics
PHYSICAL CHEMISTRY CHEMICAL PHYSICS(2022)
Abstract
We present an efficient deep reinforcement learning (DRL) approach to automatically construct time-dependent optimal control fields that enable desired transitions in dynamical chemical systems. Our DRL approach gives impressive performance in constructing optimal control fields, even for cases that are difficult to converge with existing gradient-based approaches. We provide a detailed description of the algorithms and hyperparameters as well as performance metrics for our DRL-based approach. Our results demonstrate that DRL can be employed as an effective artificial intelligence approach to efficiently and autonomously design control fields in quantum dynamical chemical systems.
MoreTranslated text
Key words
deep reinforcement learning,reinforcement learning,quantum,control,time-dependent
AI Read Science
Must-Reading Tree
Example
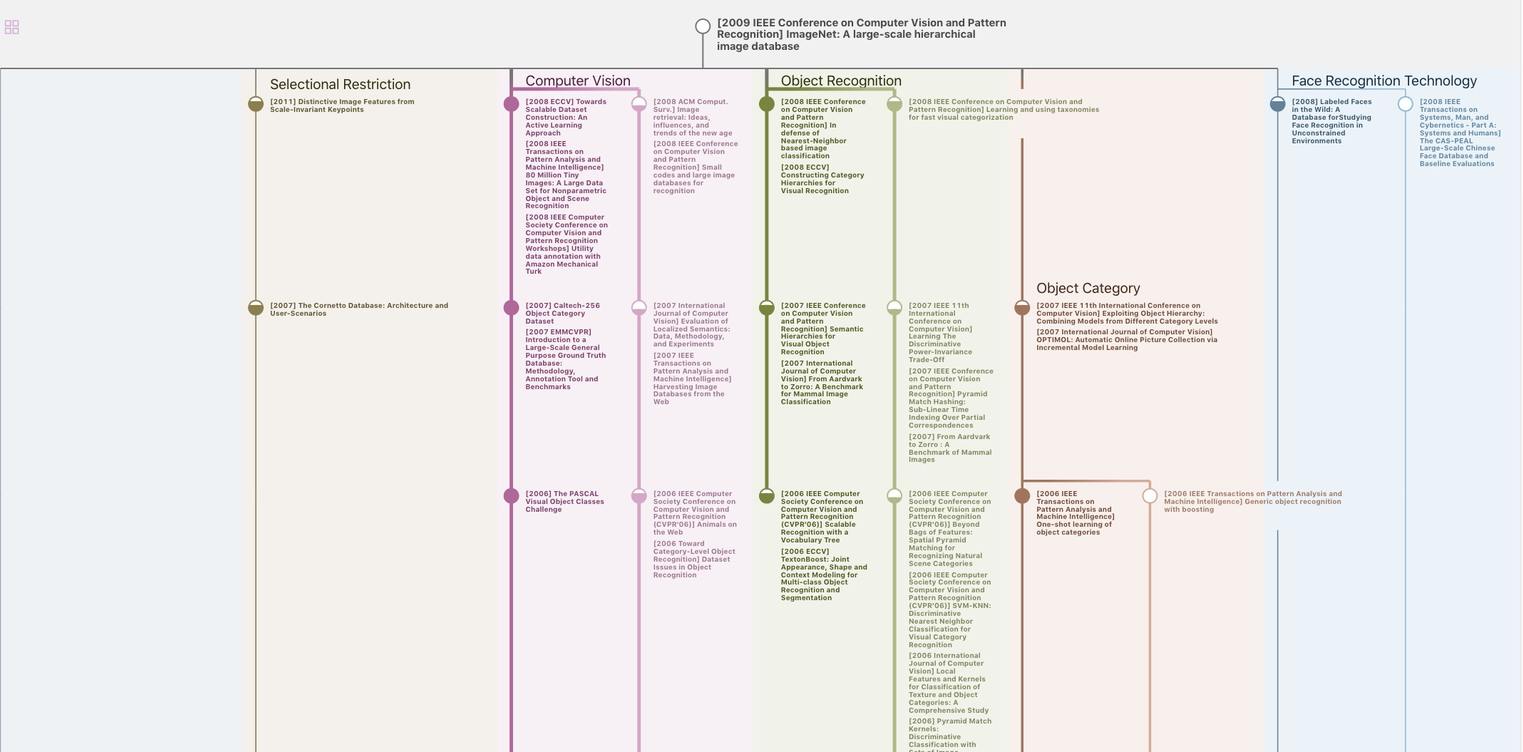
Generate MRT to find the research sequence of this paper
Chat Paper
Summary is being generated by the instructions you defined