Multi-view Local Co-occurrence and Global Consistency Learning Improve Mammogram Classification Generalisation
arxiv(2022)
摘要
When analysing screening mammograms, radiologists can naturally process information across two ipsilateral views of each breast, namely the cranio-caudal (CC) and mediolateral-oblique (MLO) views. These multiple related images provide complementary diagnostic information and can improve the radiologist's classification accuracy. Unfortunately, most existing deep learning systems, trained with globally-labelled images, lack the ability to jointly analyse and integrate global and local information from these multiple views. By ignoring the potentially valuable information present in multiple images of a screening episode, one limits the potential accuracy of these systems. Here, we propose a new multi-view global-local analysis method that mimics the radiologist's reading procedure, based on a global consistency learning and local co-occurrence learning of ipsilateral views in mammograms. Extensive experiments show that our model outperforms competing methods, in terms of classification accuracy and generalisation, on a large-scale private dataset and two publicly available datasets, where models are exclusively trained and tested with global labels.
更多查看译文
关键词
Deep learning, Supervised learning, Mammogram classification, Multi-view
AI 理解论文
溯源树
样例
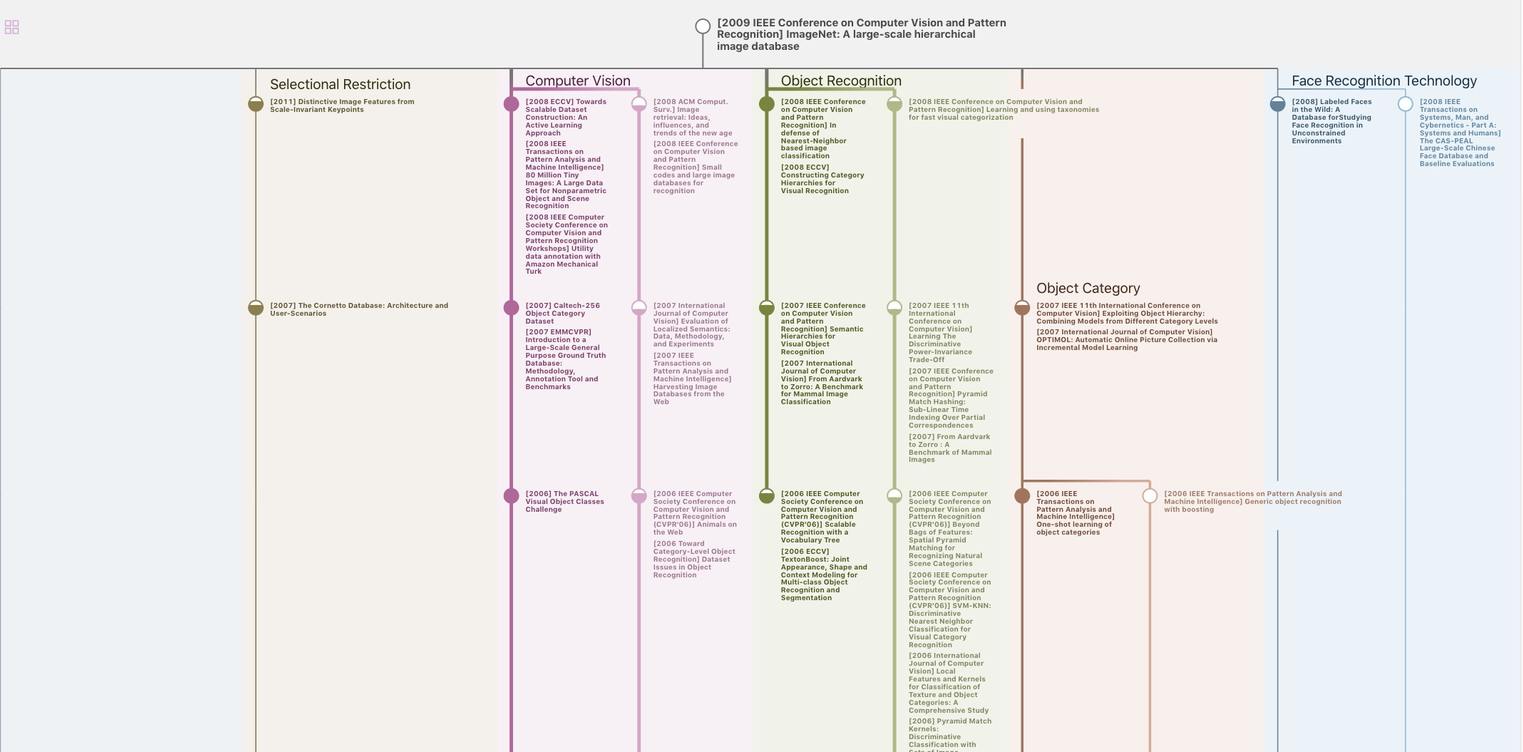
生成溯源树,研究论文发展脉络
Chat Paper
正在生成论文摘要