RNGDet++: Road Network Graph Detection by Transformer with Instance Segmentation and Multi-scale Features Enhancement
IEEE Robotics Autom Lett(2023)
Abstract
The road network graph is a critical component for downstream tasks in autonomous driving, such as global route planning and navigation. In the past years, road network graphs are usually annotated by human experts manually, which is time-consuming and labor-intensive. To annotate road network graphs effectively and efficiently, automatic algorithms for road network graph detection are demanded. Most existing methods either adopt a post-processing step on semantic segmentation maps to produce road network graphs, or propose graph-based algorithms to directly predict the graphs. However, these works suffer from hard-coded algorithms and inferior performance. To enhance the previous state-of-the-art (SOTA) method RNGDet, we add an instance segmentation head to better supervise the training, and enable the network to leverage multi-scale features of the backbone. Since the new proposed approach is improved from RNGDet, we name it RNGDet++. Experimental results show that our RNGDet++ outperforms baseline methods in terms of almost all evaluation metrics on two large-scale public datasets. Our code and supplementary materials are available at \url{https://tonyxuqaq.github.io/projects/RNGDetPlusPlus/}.
MoreTranslated text
Key words
Road network graph detection,imitation learning,autonomous driving,robotics
AI Read Science
Must-Reading Tree
Example
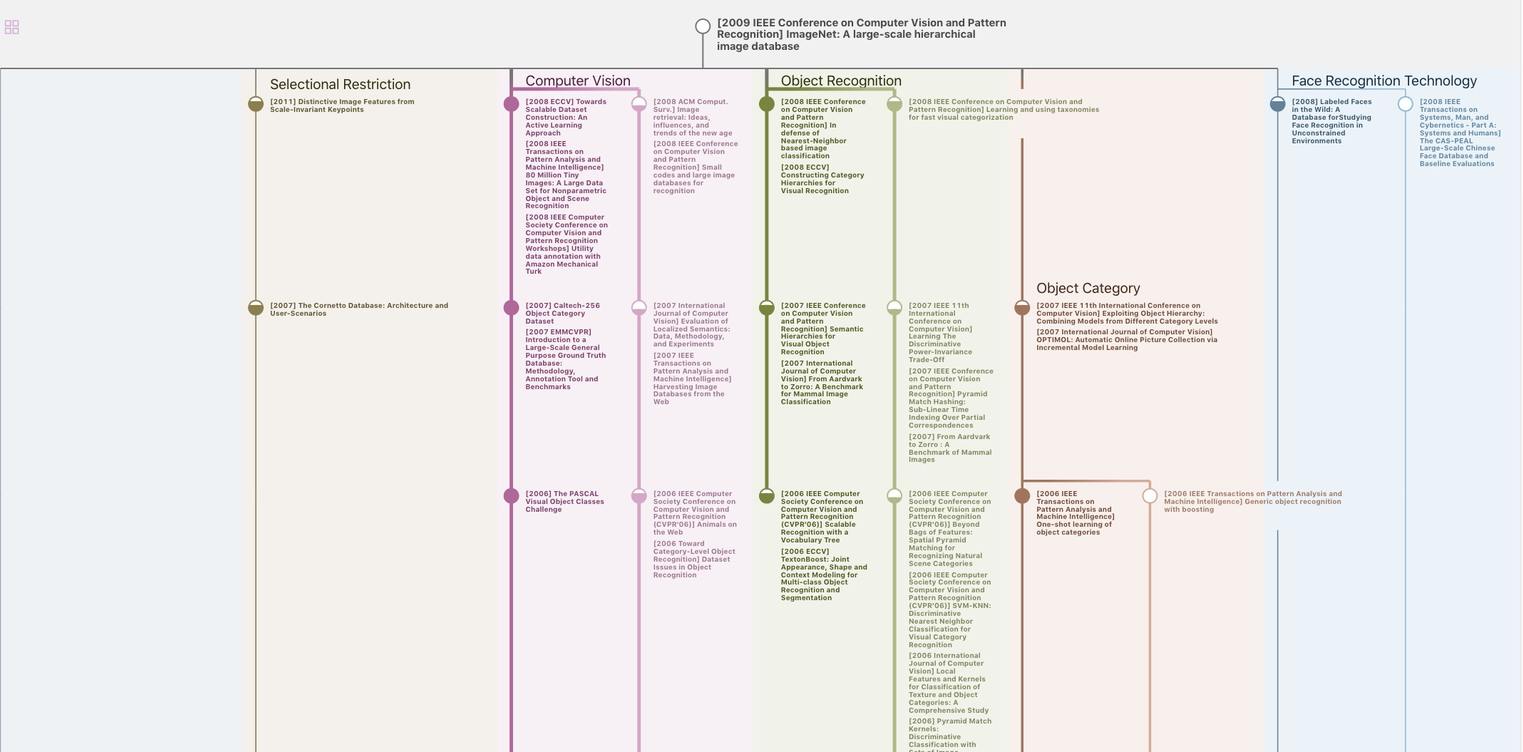
Generate MRT to find the research sequence of this paper
Chat Paper
Summary is being generated by the instructions you defined