Performance‐guaranteed containment control for pure‐feedback multi agent systems via reinforcement learning algorithm
International Journal of Robust and Nonlinear Control(2022)
摘要
AbstractIn this article, a performance‐guaranteed containment control scheme based on reinforcement learning (RL) algorithm is proposed for a class of pure‐feedback multi agent systems (MASs) with unmeasurable states. The unknown nonlinear functions are approximated by the neural networks (NNs) and an adaptive NN state observer is designed for the states estimation. Based on estimated states, the algebraic loop problem can be removed by introducing filtered signals, and the actor‐critic architecture of RL algorithm is employed to acquire the optimal controller in the framework of backstepping. Different from many optimal strategies, this article proposes a simpler mechanism based on the uniqueness of the optimal solution to obtain the actor and critic updating laws instead of gradient descent algorithm with complicated calculation. In addition, predefined performance function and an improved error transformation technique are utilized to guarantee the containment error within a prescribed boundary. By using Lyapunov stability theory and graph theory, the stability of the closed‐loop system can be demonstrated. Finally, the effectiveness of the method proposed in this article is verified by a simulation example.
更多查看译文
AI 理解论文
溯源树
样例
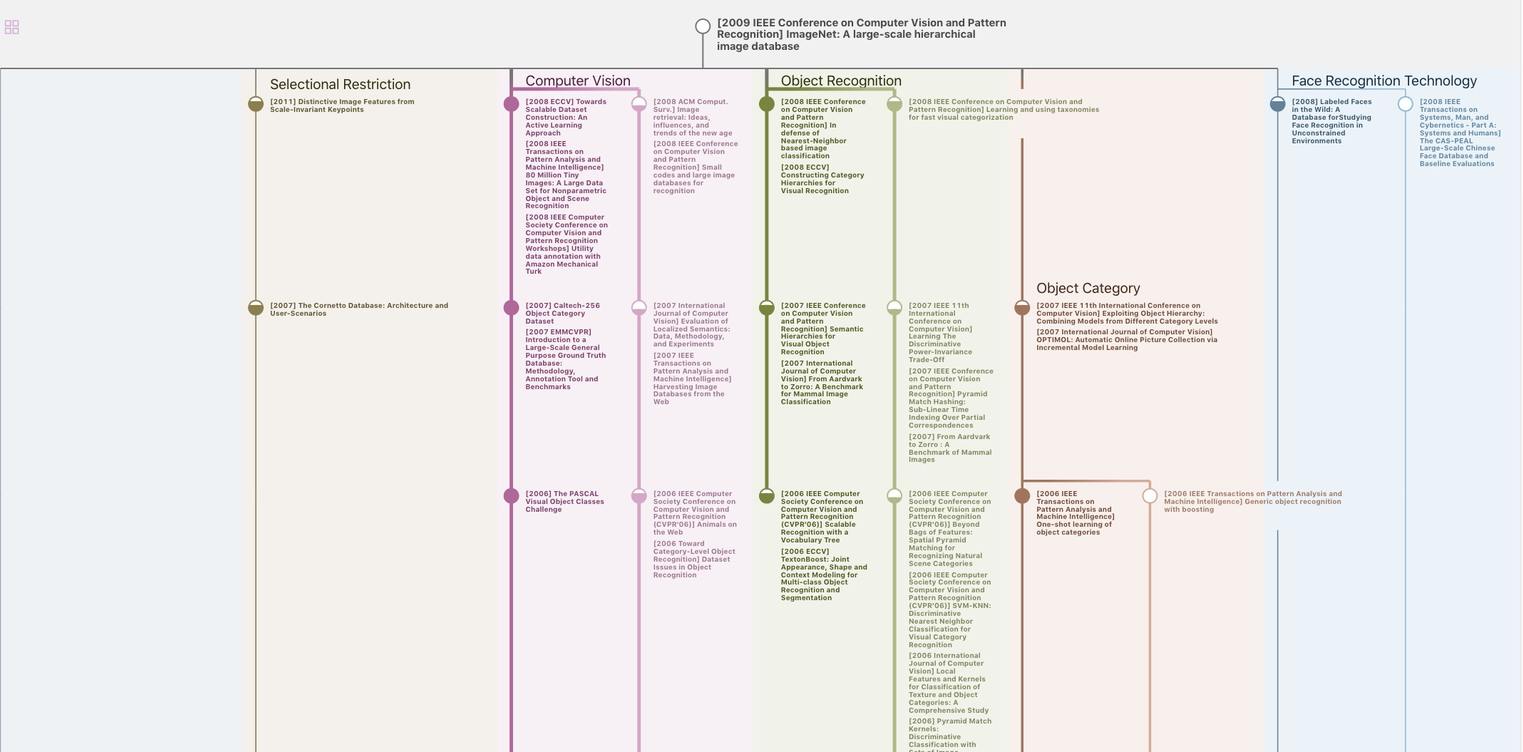
生成溯源树,研究论文发展脉络
Chat Paper
正在生成论文摘要