MCGRAM: Linking Multi-Scale CNN With a Graph-Based Recurrent Attention Model for Subject-Independent ERP Detection.
IEEE Trans. Circuits Syst. II Express Briefs(2022)
摘要
Electroencephalogram (EEG)-based event-related potential (ERP) detection is expected to play an important role in many applications. However, limited by the low signal-to-noise ratio and large inter-subject variability of EEG signals, it is still challenging to design a high-precision ERP detection method in subject-independent scenarios. In this brief, a novel graph-based multi-scale convolutional recurrent attention model (MCGRAM) is proposed to extract the underlying invariant features of EEG signals for subject-independent ERP detection. Specifically, considering the frequency factors of ERP signals, the multi-scale convolution module is designed for learning the frequency representations. Then, the graph convolution module is developed by constructing the links between each EEG node and designing trainable weights of the links to extract the discriminative spatial features. Besides, two-layer LSTM combined with a self-attention model is utilized to extract temporal representations of different EEG slices. Compared to the existing state-of-the-art methods, MCGRAM achieves the best performance in terms of AUC and F1-Score on a rapid serial visual presentation benchmark dataset. Lastly, the ablation studies demonstrate the superiority of each component in MCGRAM.
更多查看译文
关键词
EEG,subject-independent ERP detection,multi-scale convolutional neural network,graph convolution network,deep learning
AI 理解论文
溯源树
样例
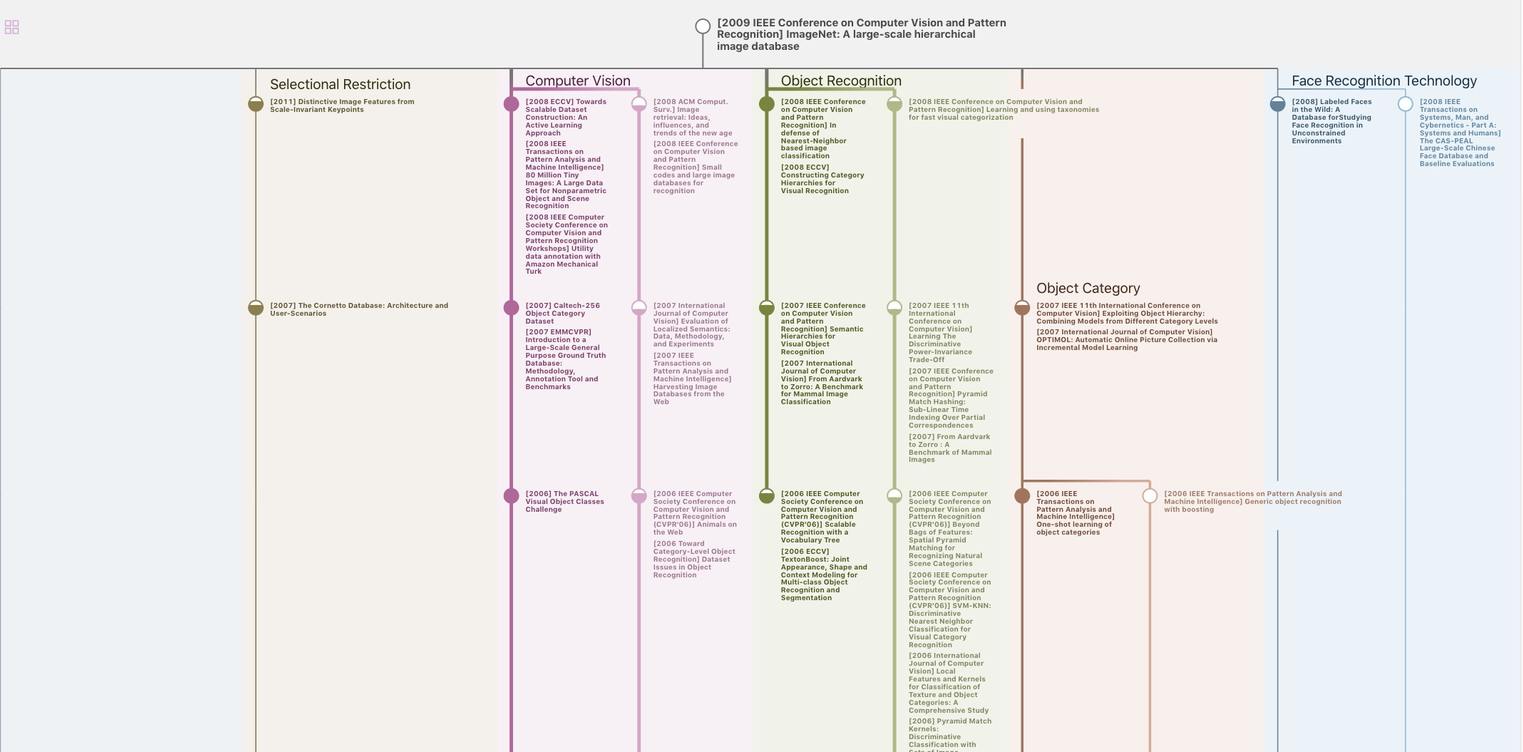
生成溯源树,研究论文发展脉络
Chat Paper
正在生成论文摘要