Frame Size Optimization Using a Machine Learning Approach in WLAN Downlink MU-MIMO Channel
arxiv(2022)
摘要
The IEEE 802.11ac/n introduced frame aggregation technology to accommodate the growing traffic demand and increase the performance of transmission efficiency and channel utilization. This is achieved by allowing many packets to be aggregated per transmission which realized a significant enhancement in the throughput performance of WLAN. However, it is difficult to efficiently utilize the benefits of frame aggregation in the downlink MU-MIMO channels as stations have heterogeneous transmission demands and data transmission rates. As a result of this, wasted space channel time will occur which degrades transmission efficiency. In addressing these challenges, the existing studies have proposed different approaches. However, most of these approaches did not consider a machine-Learning based optimization solution. The main contribution of this paper is to propose a machine-learning-based frame size optimization solution to maximize the system throughput of WLAN in the downlink MU-MIMO channel. In this approach, the Access Point (AP) performs the maximum system throughput measurement and collected frame size-system throughput patterns which contain knowledge about the effects of traffic patterns, channel conditions, and number of stations(STAs). Based on these patterns,our approach uses a neural network to correctly model the system throughput as a function of the system frame size. After training the neural network, we obtain the gradient information to adjust the frame size. the performance of the proposed Machine learning(ML) approach is evaluated over the FIFO aggregation algorithm under the effects of heterogenous traffic patterns for VoIP and video applications, channel conditions, and number of stations.
更多查看译文
关键词
wlan
AI 理解论文
溯源树
样例
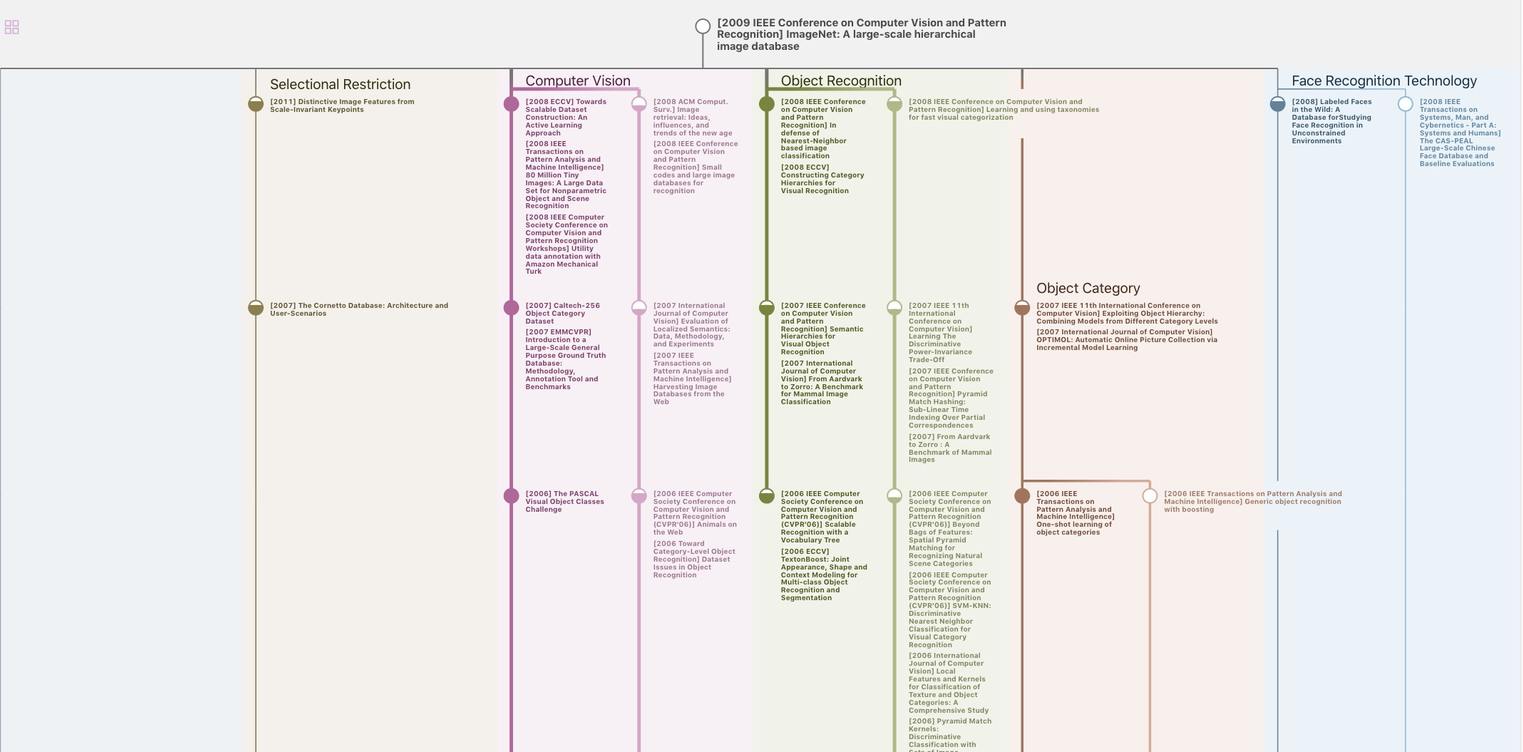
生成溯源树,研究论文发展脉络
Chat Paper
正在生成论文摘要