Deep Model Predictive Variable Impedance Control
CoRR(2022)
摘要
The capability to adapt compliance by varying muscle stiffness is crucial for dexterous manipulation skills in humans. Incorporating compliance in robot motor control is crucial to performing real-world force interaction tasks with human-level dexterity. This work presents a Deep Model Predictive Variable Impedance Controller for compliant robotic manipulation which combines Variable Impedance Control with Model Predictive Control (MPC). A generalized Cartesian impedance model of a robot manipulator is learned using an exploration strategy maximizing the information gain. This model is used within an MPC framework to adapt the impedance parameters of a low-level variable impedance controller to achieve the desired compliance behavior for different manipulation tasks without any retraining or finetuning. The deep Model Predictive Variable Impedance Control approach is evaluated using a Franka Emika Panda robotic manipulator operating on different manipulation tasks in simulations and real experiments. The proposed approach was compared with model-free and model-based reinforcement approaches in variable impedance control for transferability between tasks and performance.
更多查看译文
关键词
control,model
AI 理解论文
溯源树
样例
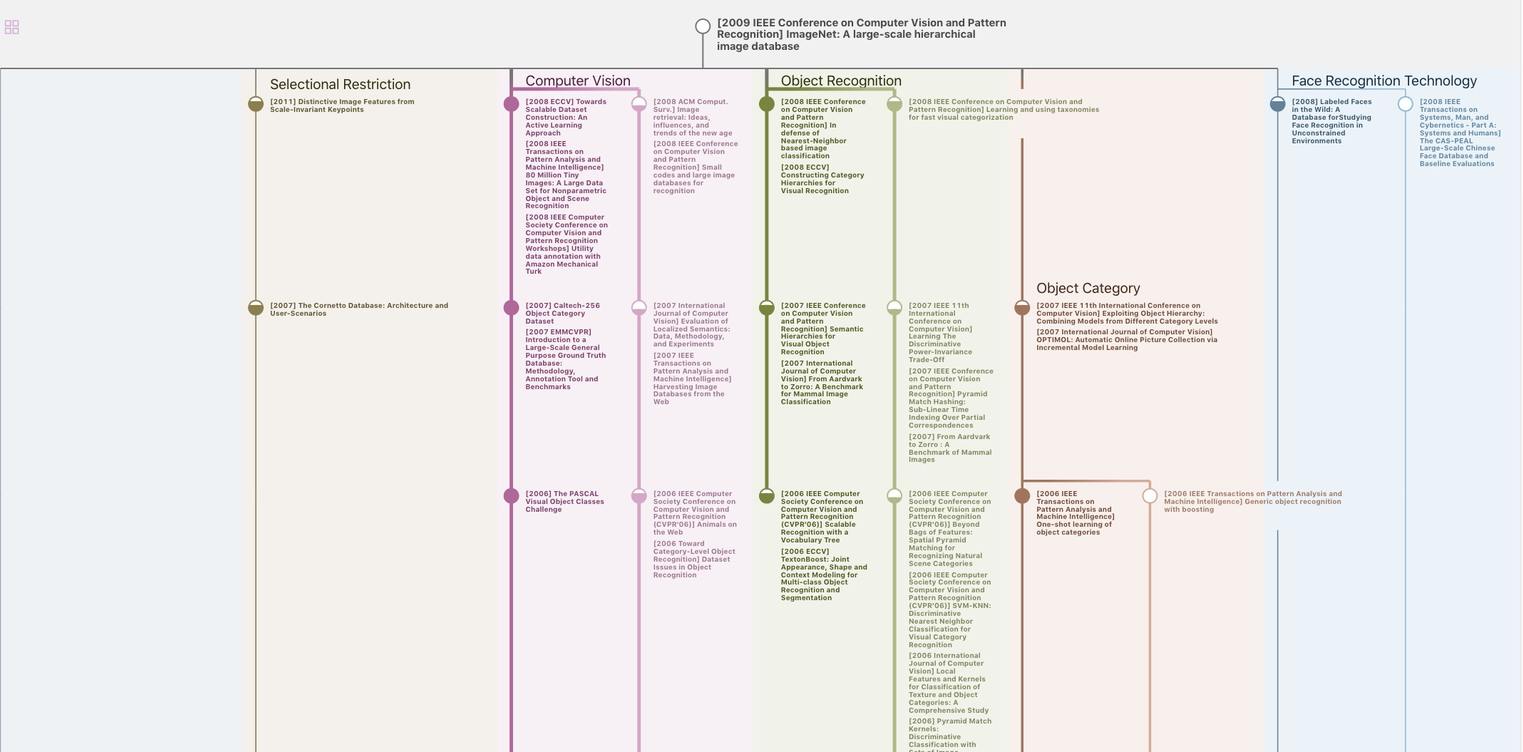
生成溯源树,研究论文发展脉络
Chat Paper
正在生成论文摘要