Multi-Source Remote Sensing Pretraining Based on Contrastive Self-Supervised Learning
REMOTE SENSING(2022)
Abstract
SAR-optical images from different sensors can provide consistent information for scene classification. However, the utilization of unlabeled SAR-optical images in deep learning-based remote sensing image interpretation remains an open issue. In recent years, contrastive self-supervised learning (CSSL) methods have shown great potential for obtaining meaningful feature representations from massive amounts of unlabeled data. This paper investigates the effectiveness of CSSL-based pretraining models for SAR-optical remote-sensing classification. Firstly, we analyze the contrastive strategies of single-source and multi-source SAR-optical data augmentation under different CSSL architectures. We find that the CSSL framework without explicit negative sample selection naturally fits the multi-source learning problem. Secondly, we find that the registered SAR-optical images can guide the Siamese self-supervised network without negative samples to learn shared features, which is also the reason why the CSSL framework outperforms the CSSL framework with negative samples. Finally, we apply the CSSL pretrained network without negative samples that can learn the shared features of SAR-optical images to the downstream domain adaptation task of optical transfer to SAR images. We find that the choice of a pretrained network is important for downstream tasks.
MoreTranslated text
Key words
multi-source,contrastive self-supervised learning,pretraining,SAR-optical
AI Read Science
Must-Reading Tree
Example
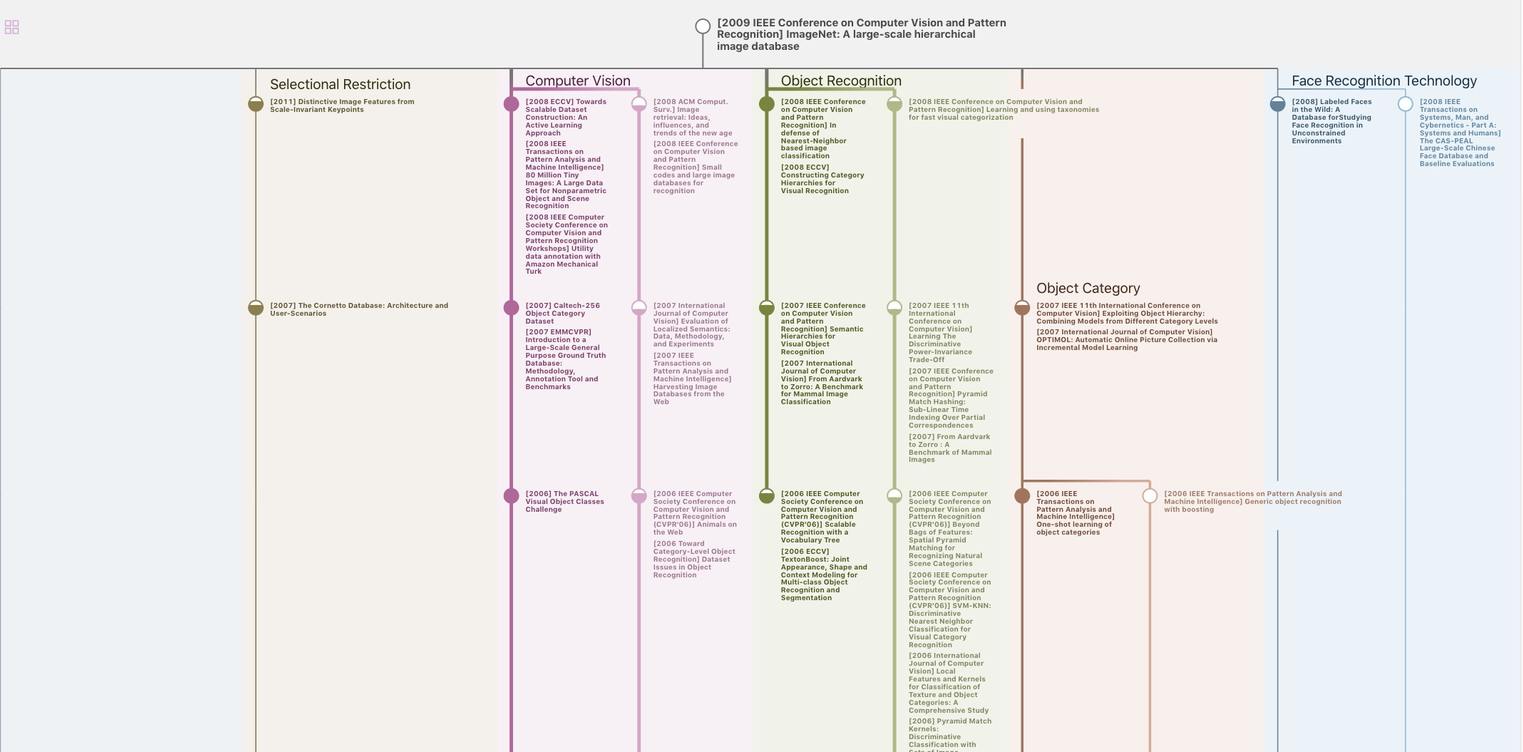
Generate MRT to find the research sequence of this paper
Chat Paper
Summary is being generated by the instructions you defined