Sharing Time-to-Event Data with Privacy Protection.
IEEE International Conference on Healthcare Informatics. IEEE International Conference on Healthcare Informatics(2022)
摘要
Sharing time-to-event data is beneficial for enabling collaborative research efforts (e.g., survival studies), facilitating the design of effective interventions, and advancing patient care (e.g., early diagnosis). Despite numerous privacy solutions for sharing time-to-event data, recent research studies have shown that external information may become available (e.g., self-disclosure of study participation on social media) to an adversary, posing new privacy concerns. In this work, we formulate a cohort inference attack for time-to-event data sharing, in which an informed adversary aims at inferring the membership of a target individual in a specific cohort. Our study investigates the privacy risks associated with time-to-event data and evaluates the empirical privacy protection offered by popular privacy-protecting solutions (e.g., binning, differential privacy). Furthermore, we propose a novel approach to privately release individual level time-to-event data with high utility, while providing indistinguishability guarantees for the input value. Our method TE-Sanitizer is shown to provide effective mitigation against the inference attacks and high usefulness in survival analysis. The results and discussion provide domain experts with insights on the privacy and the usefulness of the studied methods.
更多查看译文
关键词
Provable Privacy,Survival Analysis,Time-to-event Data
AI 理解论文
溯源树
样例
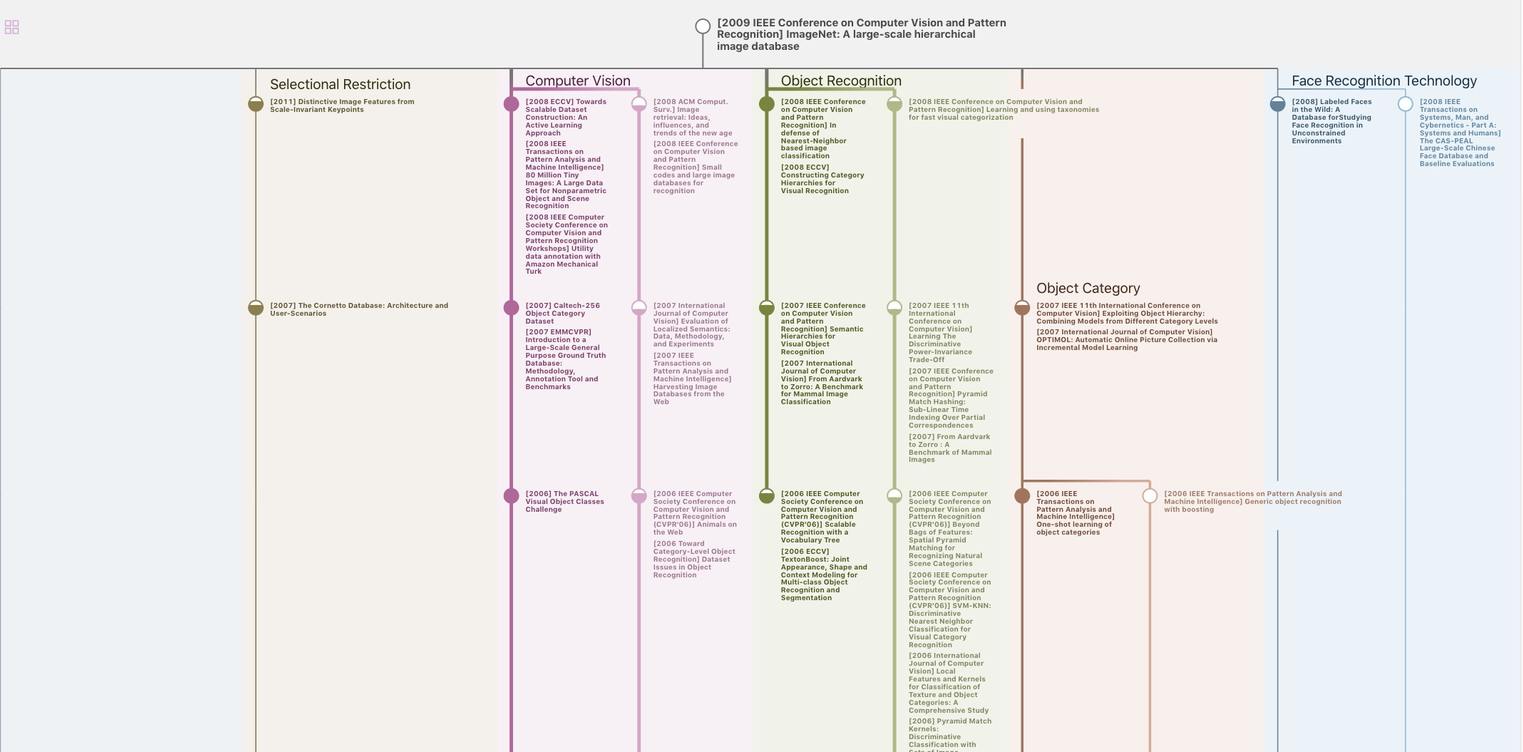
生成溯源树,研究论文发展脉络
Chat Paper
正在生成论文摘要