An inexact regularized proximal Newton method for nonconvex and nonsmooth optimization
Computational Optimization and Applications(2024)
摘要
This paper focuses on the minimization of a sum of a twice continuously differentiable function f and a nonsmooth convex function. An inexact regularized proximal Newton method is proposed by an approximation to the Hessian of f involving the ϱ th power of the KKT residual. For ϱ =0 , we justify the global convergence of the iterate sequence for the KL objective function and its R-linear convergence rate for the KL objective function of exponent 1/2. For ϱ∈ (0,1) , by assuming that cluster points satisfy a locally Hölderian error bound of order q on a second-order stationary point set and a local error bound of order q>1+ϱ on the common stationary point set, respectively, we establish the global convergence of the iterate sequence and its superlinear convergence rate with order depending on q and ϱ . A dual semismooth Newton augmented Lagrangian method is also developed for seeking an inexact minimizer of subproblems. Numerical comparisons with two state-of-the-art methods on ℓ _1 -regularized Student’s t-regressions, group penalized Student’s t-regressions, and nonconvex image restoration confirm the efficiency of the proposed method.
更多查看译文
关键词
Nonconvex and nonsmooth optimization,Regularized proximal Newton method,Global convergence,Convergence rate,KL function,Metric q-subregularity,90C26,49M15,90C55
AI 理解论文
溯源树
样例
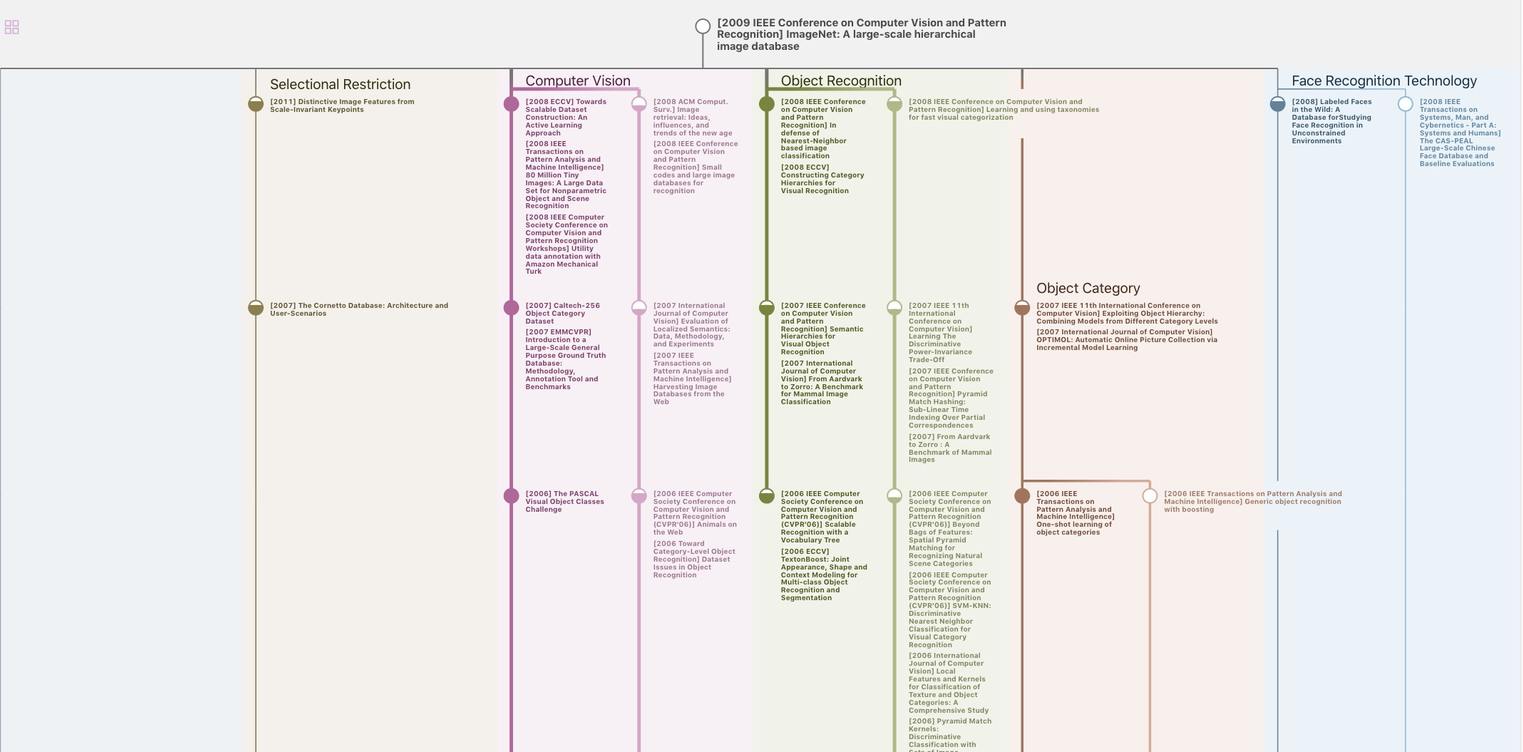
生成溯源树,研究论文发展脉络
Chat Paper
正在生成论文摘要