ActiveNeRF: Learning Where to See with Uncertainty Estimation.
European Conference on Computer Vision(2022)
摘要
Recently, Neural Radiance Fields (NeRF) has shown promising performances on reconstructing 3D scenes and synthesizing novel views from a sparse set of 2D images. Albeit effective, the performance of NeRF is highly influenced by the quality of training samples. With limited posed images from the scene, NeRF fails to generalize well to novel views and may collapse to trivial solutions in unobserved regions. This makes NeRF impractical under resource-constrained scenarios. In this paper, we present a novel learning framework, ActiveNeRF, aiming to model a 3D scene with a constrained input budget. Specifically, we first incorporate uncertainty estimation into a NeRF model, which ensures robustness under few observations and provides an interpretation of how NeRF understands the scene. On this basis, we propose to supplement the existing training set with newly captured samples based on an active learning scheme. By evaluating the reduction of uncertainty given new inputs, we select the samples that bring the most information gain. In this way, the quality of novel view synthesis can be improved with minimal additional resources. Extensive experiments validate the performance of our model on both realistic and synthetic scenes, especially with scarcer training data.
更多查看译文
关键词
Active learning,Neural radiance fields,Uncertainty estimation
AI 理解论文
溯源树
样例
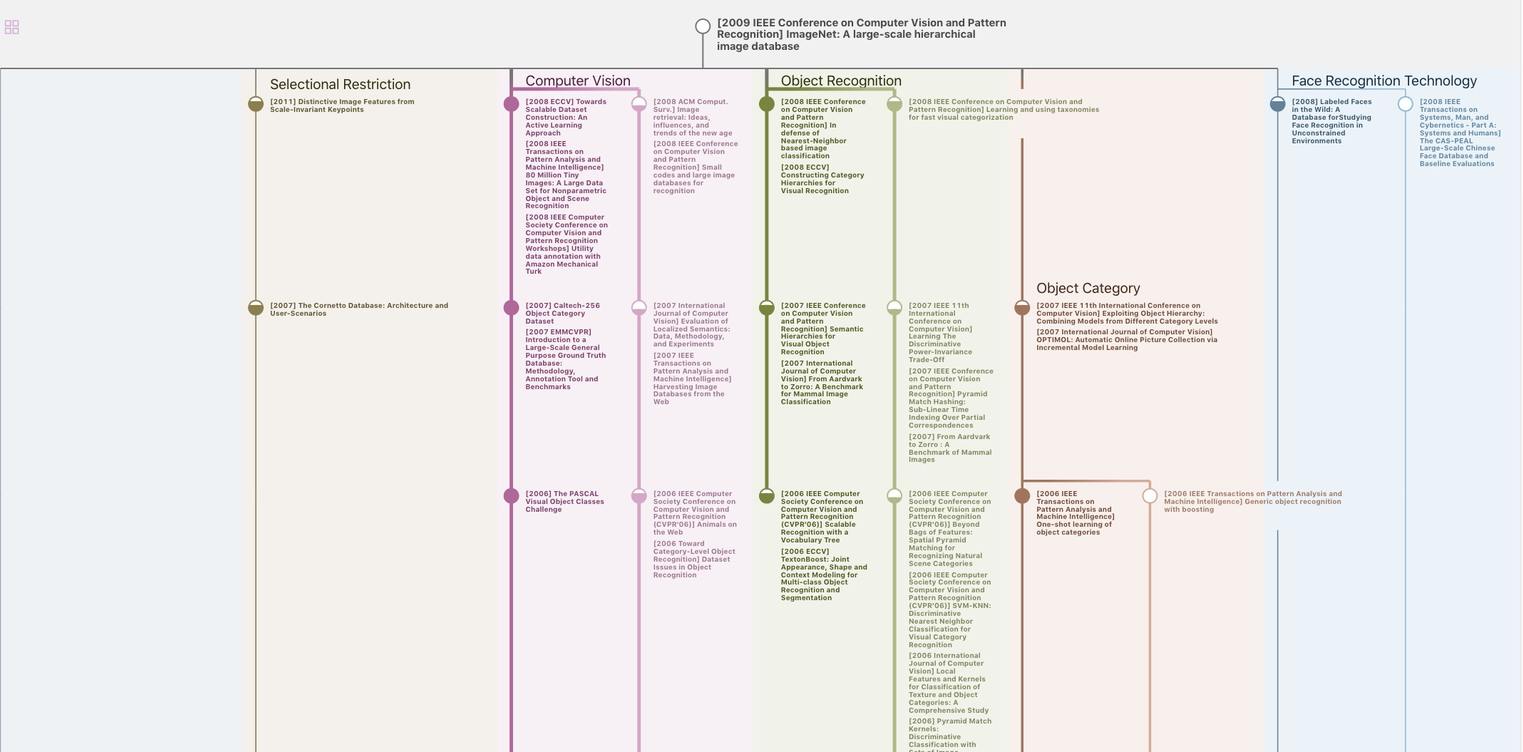
生成溯源树,研究论文发展脉络
Chat Paper
正在生成论文摘要