Rethinking Personalized Ranking at Pinterest: An End-to-End Approach
ACM Conference On Recommender Systems(2022)
摘要
BSTRACT In this work, we present our journey to revolutionize the personalized recommendation engine through end-to-end learning from raw user actions. We encode user’s long-term interest in PinnerFormer, a user embedding optimized for long-term future actions via a new dense all-action loss, and capture user’s short-term intention by directly learning from the real-time action sequences. We conducted both offline and online experiments to validate the performance of the new model architecture, and also address the challenge of serving such a complex model using mixed CPU/GPU setup in production. The proposed system has been deployed in production at Pinterest and has delivered significant online gains across organic and Ads applications.
更多查看译文
AI 理解论文
溯源树
样例
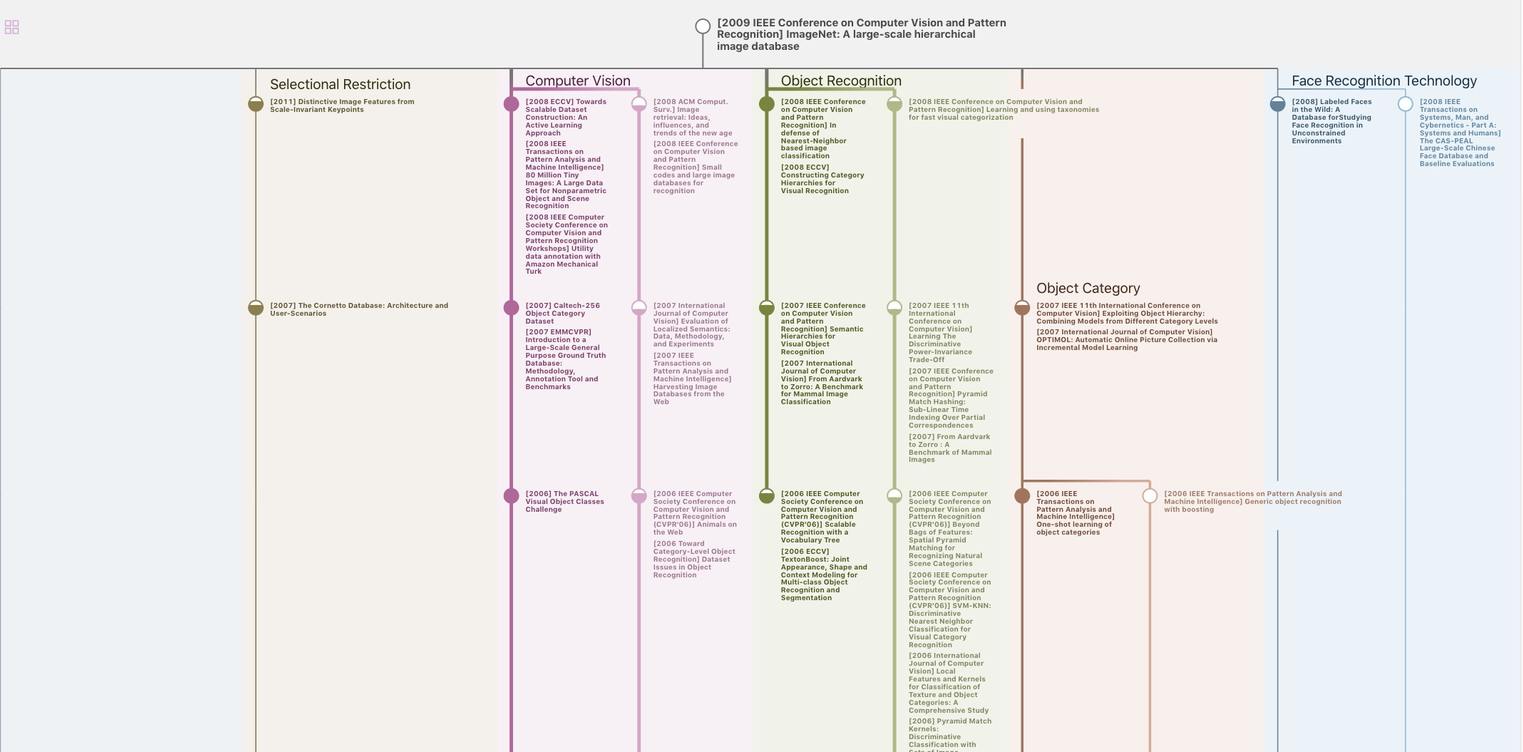
生成溯源树,研究论文发展脉络
Chat Paper
正在生成论文摘要