Confidence-Guided Data Augmentation for Improved Semi-Supervised Training
arXiv (Cornell University)(2023)
摘要
We propose a new strategy to improve the accuracy and robustness of image classification. First, we train a baseline CNN model. Then, we identify challenging regions in the feature space by identifying all misclassified samples, and correctly classified samples with low confidence values. These samples are then used to train a Variational AutoEncoder (VAE). Next, the VAE is used to generate synthetic images. Finally, the generated synthetic images are used in conjunction with the original labeled images to train a new model in a semi-supervised fashion. Empirical results on benchmark datasets such as STL10 and CIFAR-100 show that the synthetically generated samples can further diversify the training data, leading to improvement in image classification in comparison with the fully supervised baseline approaches using only the available data.
更多查看译文
关键词
training,data,confidence-guided,semi-supervised
AI 理解论文
溯源树
样例
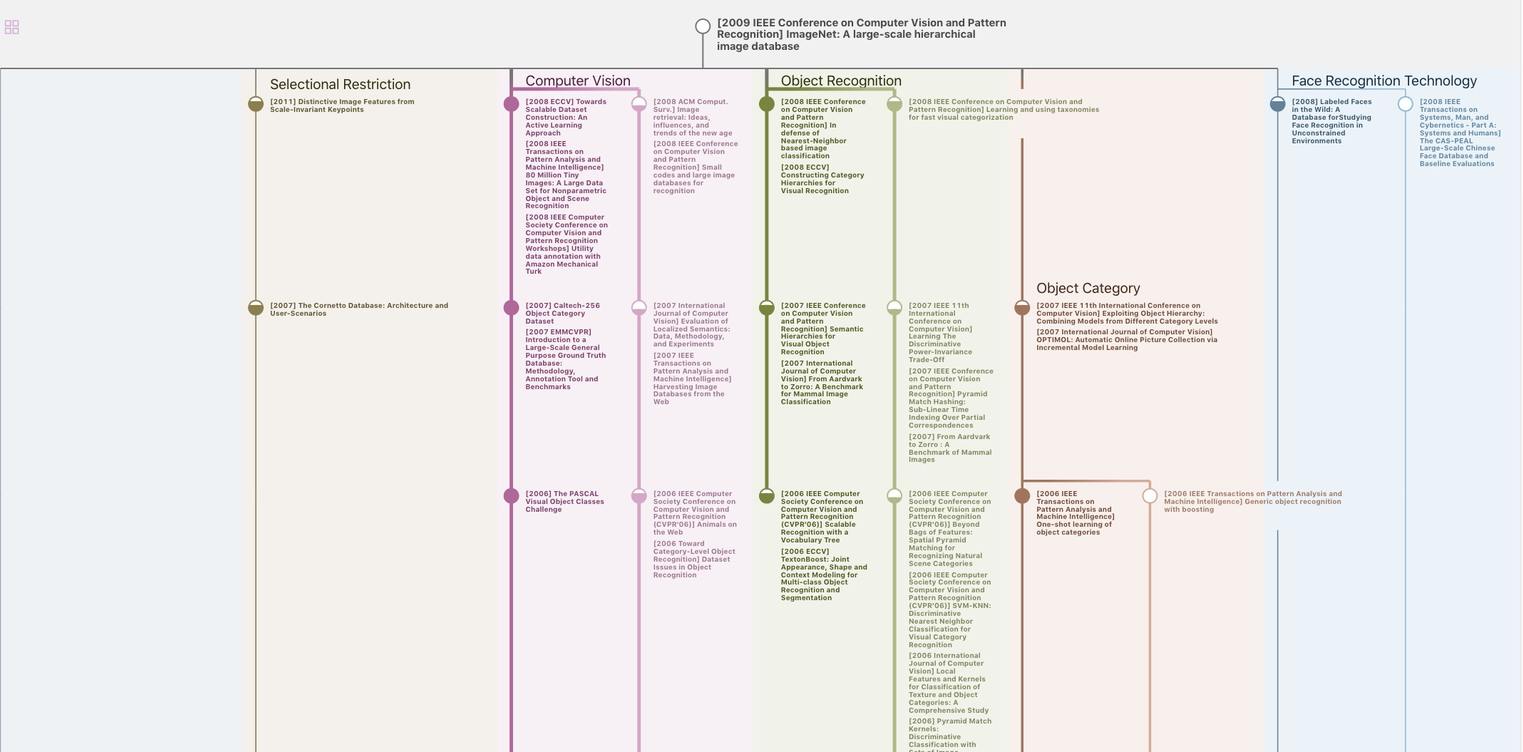
生成溯源树,研究论文发展脉络
Chat Paper
正在生成论文摘要