Comprehensively identifying Long Covid articles with human-in-the-loop machine learning.
Patterns (New York, N.Y.)(2023)
摘要
A significant percentage of COVID-19 survivors experience ongoing multisystemic symptoms that often affect daily living, a condition known as Long Covid or post-acute-sequelae of SARS-CoV-2 infection. However, identifying scientific articles relevant to Long Covid is challenging since there is no standardized or consensus terminology. We developed an iterative human-in-the-loop machine learning framework combining data programming with active learning into a robust ensemble model, demonstrating higher specificity and considerably higher sensitivity than other methods. Analysis of the Long Covid Collection shows that (1) most Long Covid articles do not refer to Long Covid by any name, (2) when the condition is named, the name used most frequently in the literature is Long Covid, and (3) Long Covid is associated with disorders in a wide variety of body systems. The Long Covid Collection is updated weekly and is searchable online at the LitCovid portal: https://www.ncbi.nlm.nih.gov/research/coronavirus/docsum?filters=e_condition.LongCovid.
更多查看译文
关键词
COVID-19,Long Covid,active learning,data programming,machine learning,natural language processing,post-acute sequelae of SARS-CoV-2 infection,text classification,weak supervision
AI 理解论文
溯源树
样例
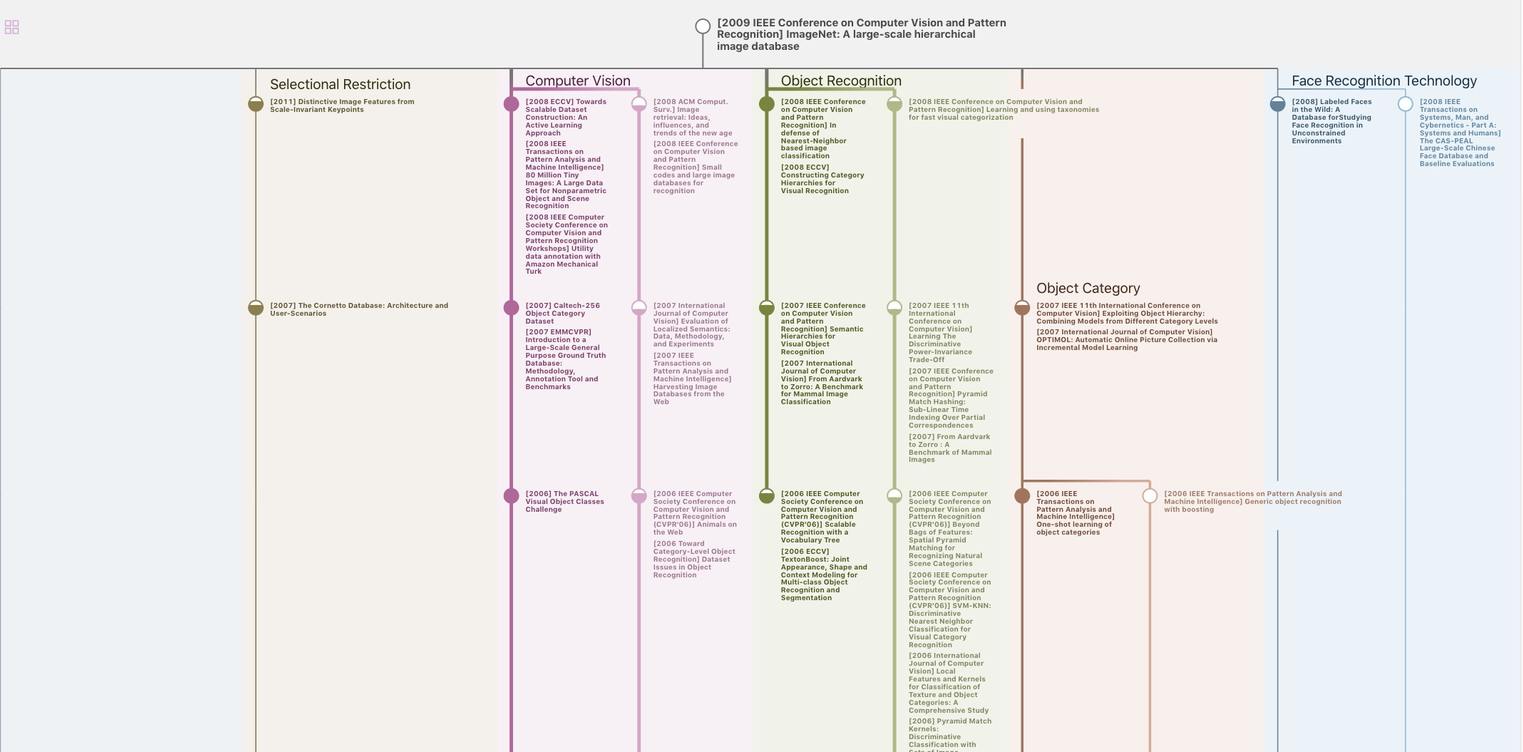
生成溯源树,研究论文发展脉络
Chat Paper
正在生成论文摘要