Mitigating urinary incontinence condition using machine learning
BMC Medical Informatics and Decision Making(2022)
摘要
Background Urinary incontinence (UI) is the inability to completely control the process of releasing urine. UI presents a social, medical, and mental issue with financial consequences. Objective This paper proposes a framework based on machine learning for predicting urination time, which can benefit people with various degrees of UI. Method A total of 850 data points were self-recorded by 51 participants to investigate how different factors impact urination time. The participants were instructed to record input data (such as the time of consumption and the number of drinks) and output data (i.e., the time the individual urinated). Other factors, such as age and BMI, were also considered. The study was conducted in two phases: (1) data was prepared for modeling, including missing values, data encoding, and scaling; and (2) a classification model was designed with four output classes of the next urination time: < = 30 min, 31–60 min, 61–90 min, > 90 min. The model was built in two steps: (1) feature selection and (2) model training and testing. Feature selection methods such as lasso regression, decision tree, random forest, and chi-square were used to select the best features, which were then used to train an extreme gradient boosting (XGB) algorithm model to predict the class of the next urination time. Result The feature selection steps resulted in nine features considered the most important features affecting UI. The accuracy, precision, recall, and F1 score of the XGB predictive model are 0.70, 0.73, 0.70, and 0.71, respectively. Conclusion This research is the first step in developing a machine learning model to predict when a person will need to urinate. A precise predictive instrument can enable healthcare providers and caregivers to assist people with various forms of UI in reliable, prompted voiding. The insights from this predictive model can allow future apps to go beyond current UI-related apps by predicting the time of urination using the most relevant factors that impact voiding frequency.
更多查看译文
关键词
Machine learning, Urinary incontinence, Urination, Bladder voiding
AI 理解论文
溯源树
样例
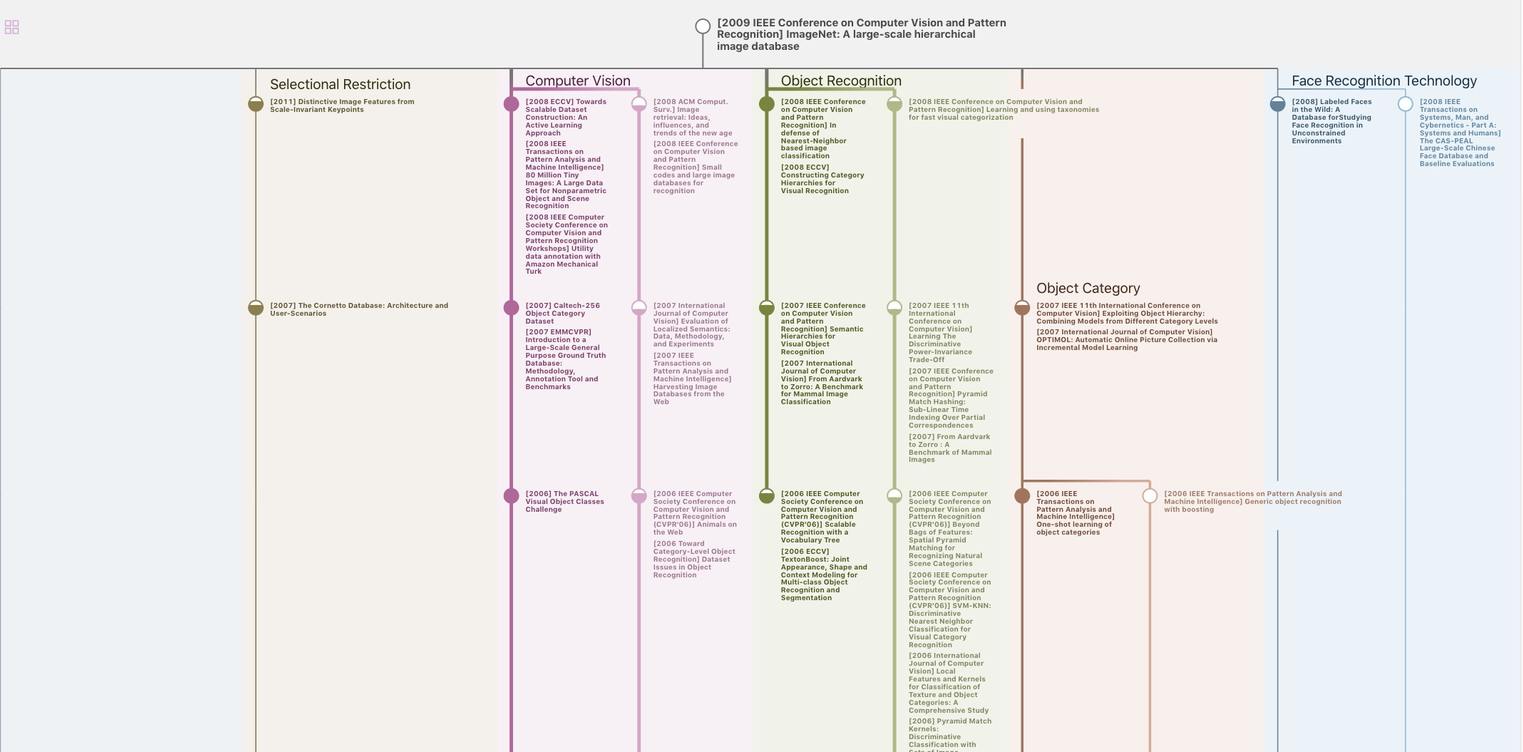
生成溯源树,研究论文发展脉络
Chat Paper
正在生成论文摘要