Focused Feature Differentiation Network for Image Quality Assessment
IEEE Conference on Computer Vision and Pattern Recognition(2022)
摘要
Image quality assessment (IQA) intended to assess the perceptual quality of images has been an essential problem in both human and machine vision. Recently, with the help of deep neural network (DNN), IQA algorithms can extract more valuable differences between the distorted and reference images than the traditional algorithms, and thus the performance of DNN-based algorithms is more satisfactory than that of previous algorithms. However, the accuracy for different distorted images preference rating of the existing DNN-based quality assessment methods will be decreased when multiple distorted images are quite similar to each other or to the reference image. To tackle this problem, we propose a focused feature differentiation network (FFDN) to highlight the feature maps with greater distorted and reference differentiation. Furthermore, we use the multi-scale feature fusion module to fuse the focused differentiation features at different scale receptive fields. To further improve the accuracy of our method, we predict the mean opinion score and differentiation score by stages and combine them with different self-learning weights. Finally, we convert the weighted score into different image preference degrees. Experimental results on the validation dataset of CLIC2022 and test dataset of CLIC2021 show that the accuracy of our model FFDN is higher than other excellent quality assessment methods.
更多查看译文
关键词
multiscale feature fusion module,focused differentiation features,different scale receptive fields,mean opinion score,differentiation score,focused feature differentiation network,image quality assessment,human machine vision,deep neural network,IQA algorithms,valuable differences,distorted reference images,DNN-based quality assessment methods,multiple distorted images,reference image,feature maps,distorted reference differentiation,distorted image preference rating,image preference degrees,self-learning weights
AI 理解论文
溯源树
样例
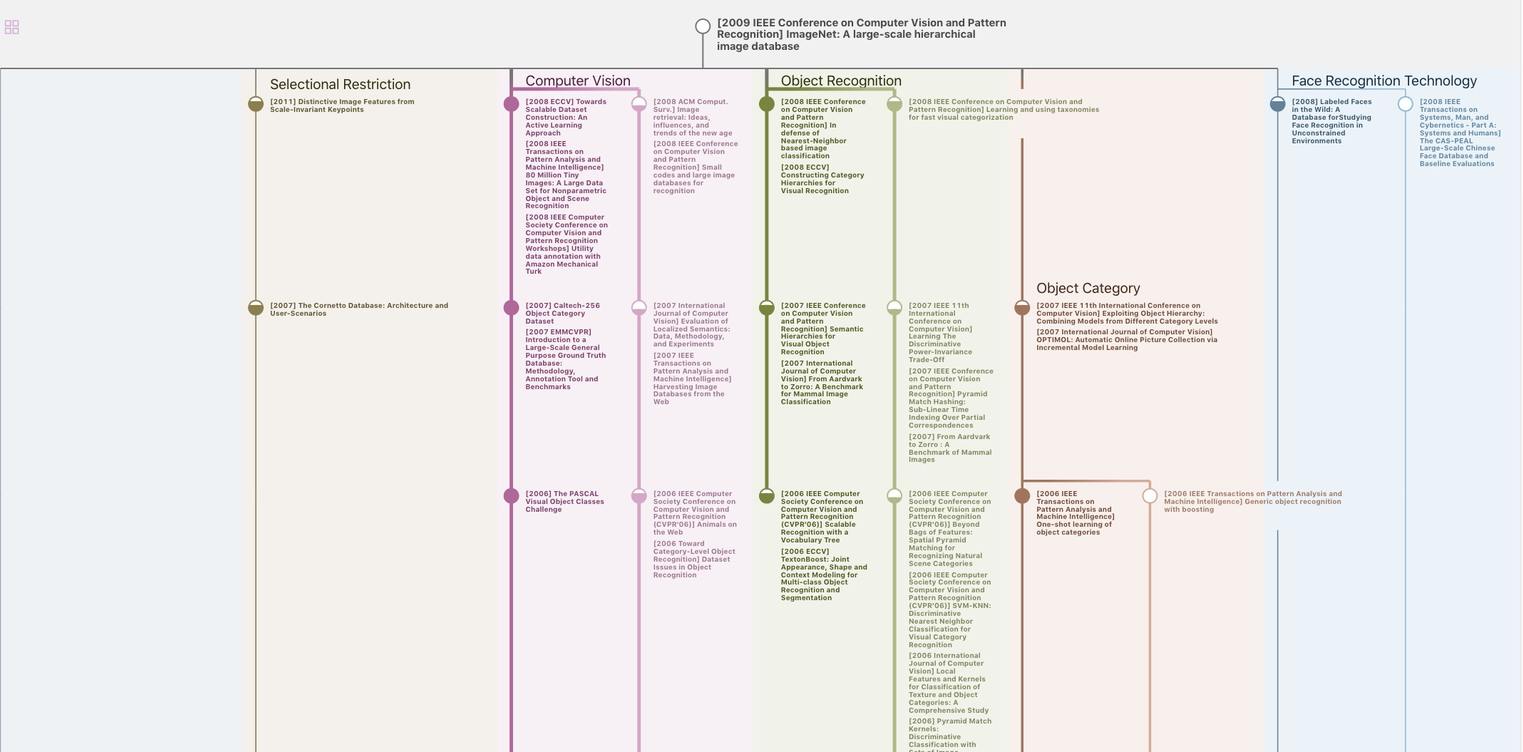
生成溯源树,研究论文发展脉络
Chat Paper
正在生成论文摘要