Improving 3D edge detection for visual inspection of MRI coregistration and alignment.
Journal of neuroscience methods(2024)
摘要
BACKGROUND:Visualizing edges is critical for neuroimaging. For example, edge maps enable quality assurance for the automatic alignment of an image from one modality (or individual) to another.
NEW METHOD:We suggest that using the second derivative (difference of Gaussian, or DoG) provides robust edge detection. This method is tuned by size (which is typically known in neuroimaging) rather than intensity (which is relative).
RESULTS:We demonstrate that this method performs well across a broad range of imaging modalities. The edge contours produced consistently form closed surfaces, whereas alternative methods may generate disconnected lines, introducing potential ambiguity in contiguity.
COMPARISON WITH EXISTING METHODS:Current methods for computing edges are based on either the first derivative of the image (FSL), or a variation of the Canny Edge detection method (AFNI). These methods suffer from two primary limitations. First, the crucial tuning parameter for each of these methods relates to the image intensity. Unfortunately, image intensity is relative for most neuroimaging modalities making the performance of these methods unreliable. Second, these existing approaches do not necessarily generate a closed edge/surface, which can reduce the ability to determine the correspondence between a represented edge and another image.
CONCLUSION:The second derivative is well suited for neuroimaging edge detection. We include this method as part of both the AFNI and FSL software packages, standalone code and online.
更多查看译文
AI 理解论文
溯源树
样例
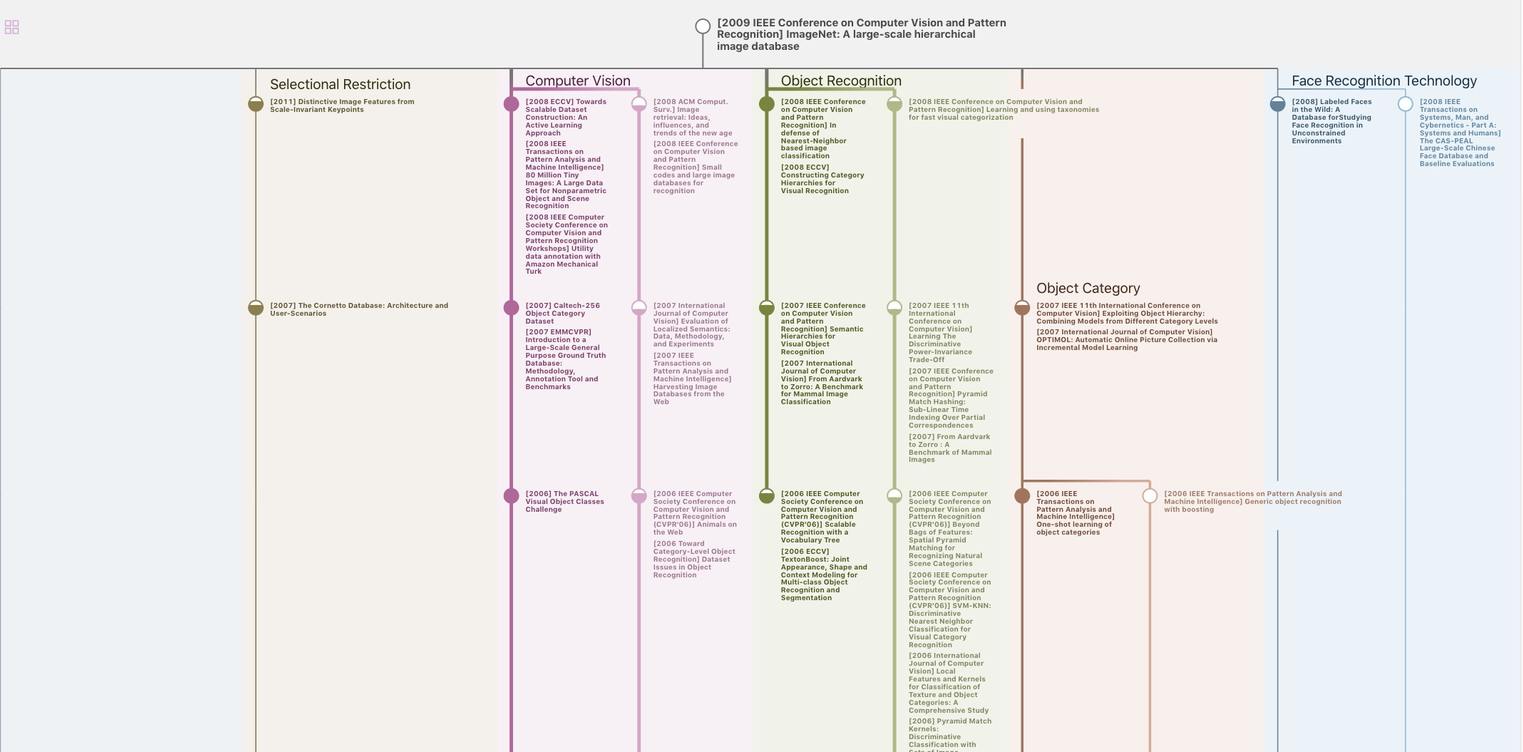
生成溯源树,研究论文发展脉络
Chat Paper
正在生成论文摘要