Tracked-Vehicle Retrieval by Natural Language Descriptions With Domain Adaptive Knowledge
IEEE Conference on Computer Vision and Pattern Recognition(2022)
摘要
This paper introduces our solution for Track 2 in AI City Challenge 2022. The task is Tracked-Vehicle Retrieval by Natural Language Descriptions with a real-world dataset of various scenarios and cameras. We mainly focus on developing a robust natural language-based vehicle retrieval system to address the domain bias problem due to unseen scenarios and multi-view multi-camera vehicle tracks. Specifically, we apply CLIP [16] to effectively extract both visual and textual representations for contrastive representation learning. Furthermore, for new scenarios in the test set, we pro-pose a novel Domain Adaptive Training method that utilizes information from labeled data and transfers it to the unseen domain by generating pseudo labels. By using this simple and effective strategy, we not only bridge the domain gap between the training set and test set, but also require less computational cost and data compared to previous top performance methods. Finally, we employ a context-sensitive post-processing method to address model’s uncertainty and eliminate the wrong retrieved vehicle track. Taking one step further, we also investigate the impact of different text formats and the number of pseudo labels data for the fine-tuning process. Our proposed method has achieved 3rd place in the AI City Challenge 2022, yielding a competitive performance of 47.73% MRR accuracy on the private test set, which verified the effectiveness of the proposed solution.
更多查看译文
关键词
natural language descriptions,knowledge,tracked-vehicle
AI 理解论文
溯源树
样例
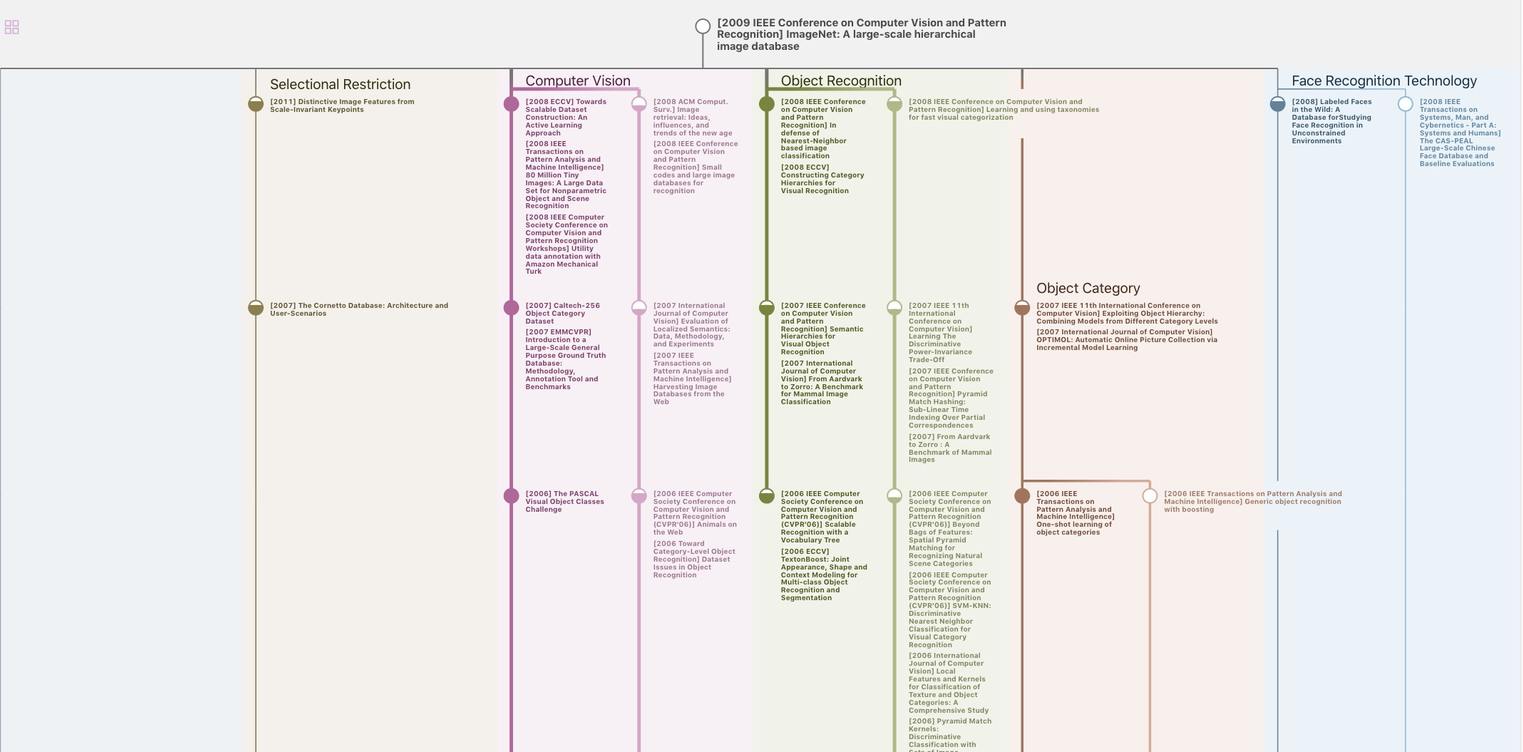
生成溯源树,研究论文发展脉络
Chat Paper
正在生成论文摘要