Area Under the ROC Curve Maximization for Metric Learning
IEEE Conference on Computer Vision and Pattern Recognition(2022)
摘要
Most popular metric learning losses have no direct relation with the evaluation metrics that are subsequently applied to evaluate their performance. We hypothesize that training a metric learning model by maximizing the area under the ROC curve (which is a typical performance measure of recognition systems) can induce an implicit ranking suitable for retrieval problems. This hypothesis is supported by previous work that proved that a curve dominates in ROC space if and only if it dominates in Precision-Recall space. To test this hypothesis, we design and maximize an approximated, derivable relaxation of the area under the ROC curve. The proposed AUC loss achieves state-of-the-art results on two large scale retrieval benchmark datasets (Stanford Online Products and DeepFashion In-Shop). Moreover, the AUC loss achieves comparable performance to more complex, domain specific, state-of-the-art methods for vehicle re-identification.
更多查看译文
关键词
roc curve maximization,roc curve,metric,learning
AI 理解论文
溯源树
样例
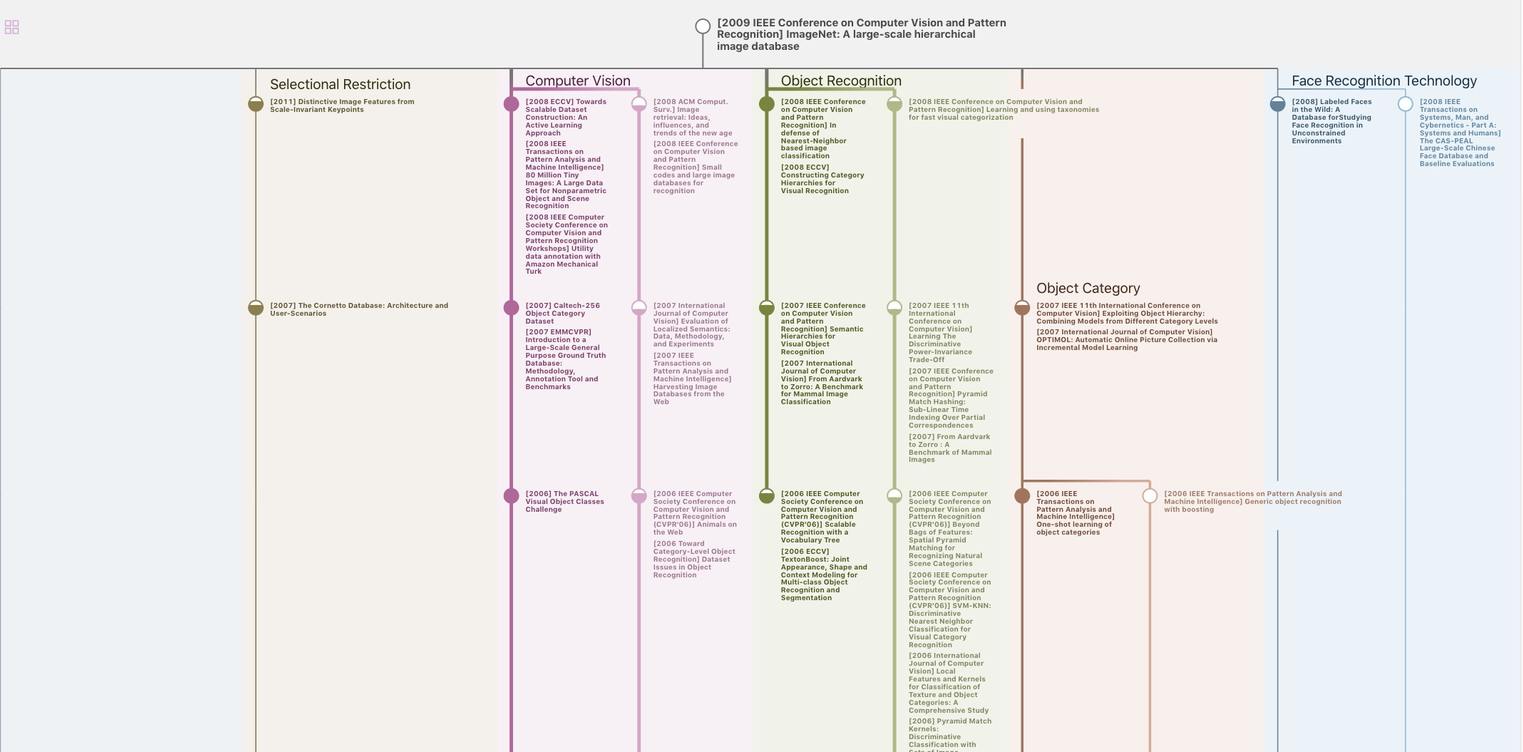
生成溯源树,研究论文发展脉络
Chat Paper
正在生成论文摘要