Label-free imaging of 3D pluripotent stem cell differentiation dynamics on chip
biorxiv(2022)
摘要
The dynamic chemical and architectural microenvironments of 3D stem cell cultures can be controlled by integration into a microfluidic chip. Massive parallelized 3D stem cell cultures for engineering in vitro human cell types require new imaging methods with high time and spatial resolution to fully exploit technological advances in cell culture. Here, we introduce a label-free deep learning method called Bright2Nuc to predict in silico nuclear staining in 3D from bright-field images obtained using traditional confocal microscopy. Bright2Nuc was trained and applied to several hundred 3D human induced pluripotent stem cell cultures differentiating towards definitive endoderm on a microfluidic platform. Combined with existing image analysis tools, Bright2Nuc segmented individual nuclei from bright-field images, quantified their morphological properties, predicted stem cell differentiation state, and tracked the cells over time. Our methods are available in an open-source pipeline that enables researchers to upscale 3D cell phenotyping in stem cell culture.
### Competing Interest Statement
The authors have declared no competing interest.
更多查看译文
关键词
stem,cell,label-free
AI 理解论文
溯源树
样例
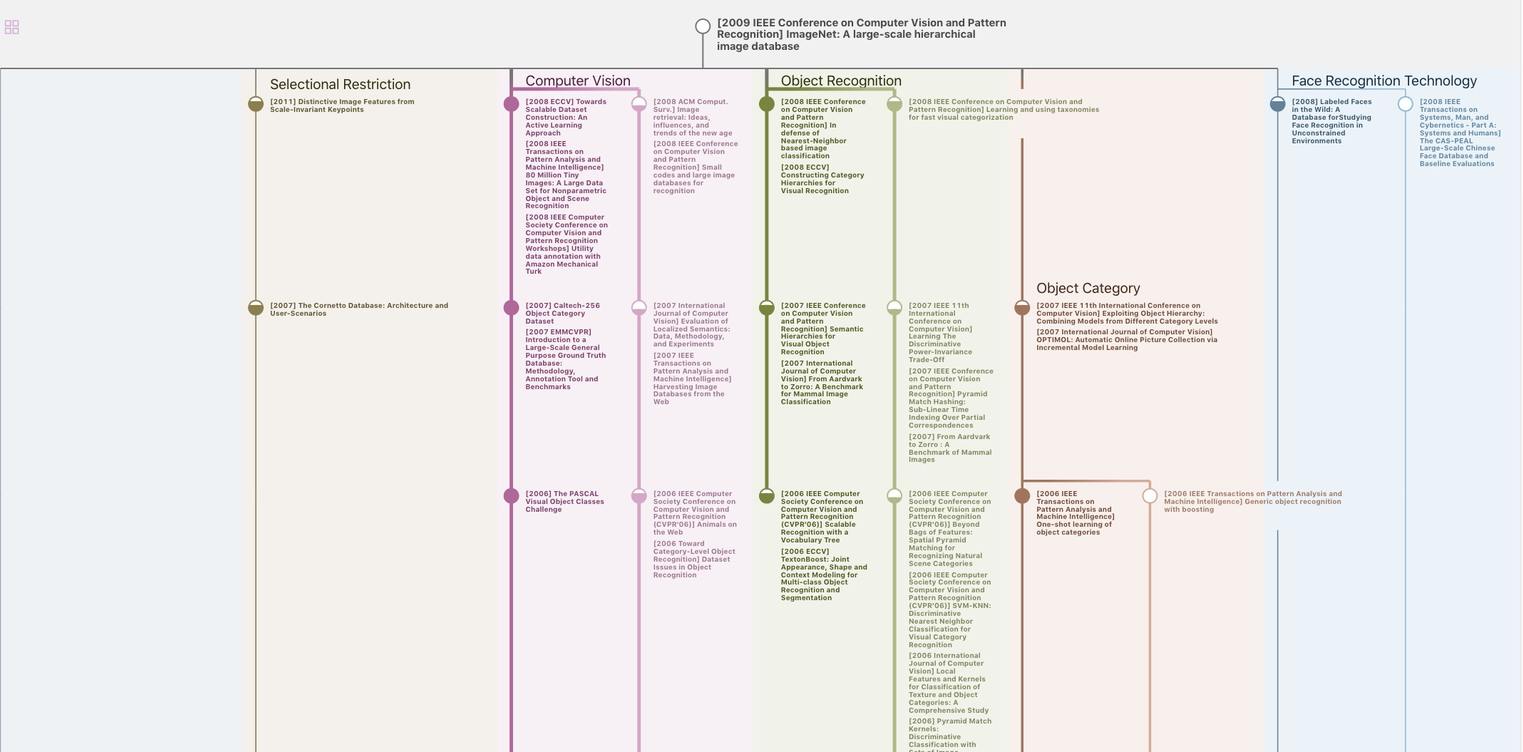
生成溯源树,研究论文发展脉络
Chat Paper
正在生成论文摘要