Investigating the human and non-obese diabetic mouse MHC class II immunopeptidome using protein language modelling
Bioinformatics(2022)
Abstract
Identifying peptides associated with the major histocompability complex class II (MHCII) is a central task in the evaluation of the immunoregulatory function of therapeutics and drug prototypes. MHCII-peptide presentation prediction has multiple biopharmaceutical applications, including the safety assessment of biologics and engineered derivatives in silico , or the fast progression of antigen-specific immunomodulatory drug discovery programs in immune disease and cancer. This has resulted in the collection of large–scale data sets on adaptive immune receptor antigenic responses and MHC-associated peptide proteomics. In parallel, recent deep learning algorithmic advances in natural language processing (NLP) and protein lan-guage modelling (PLM) have shown potential in leveraging large collections of sequence data and improve MHC presentation prediction. Here, we train a compact transformer model (AEGIS) on human and mouse MHCII immunopeptidome data, including a preclinical murine model, and evaluate its performance on the peptide presentation prediction task. We show that the transformer performs on par with existing deep learning algorithms and that combining datasets from multiple organisms increases model performance. We trained variants of the model with and without MHCII information. In both alternatives, the inclusion of peptides presented by the I-Ag7 MHC class II molecule expressed by the non-obese diabetic (NOD) mice enabled the in silico prediction of presented peptides in a preclinical type 1 diabetes model organism, which has promising therapeutic applications.
Availability and implementation The source code is available at .
### Competing Interest Statement
The authors have declared no competing interest.
MoreTranslated text
AI Read Science
Must-Reading Tree
Example
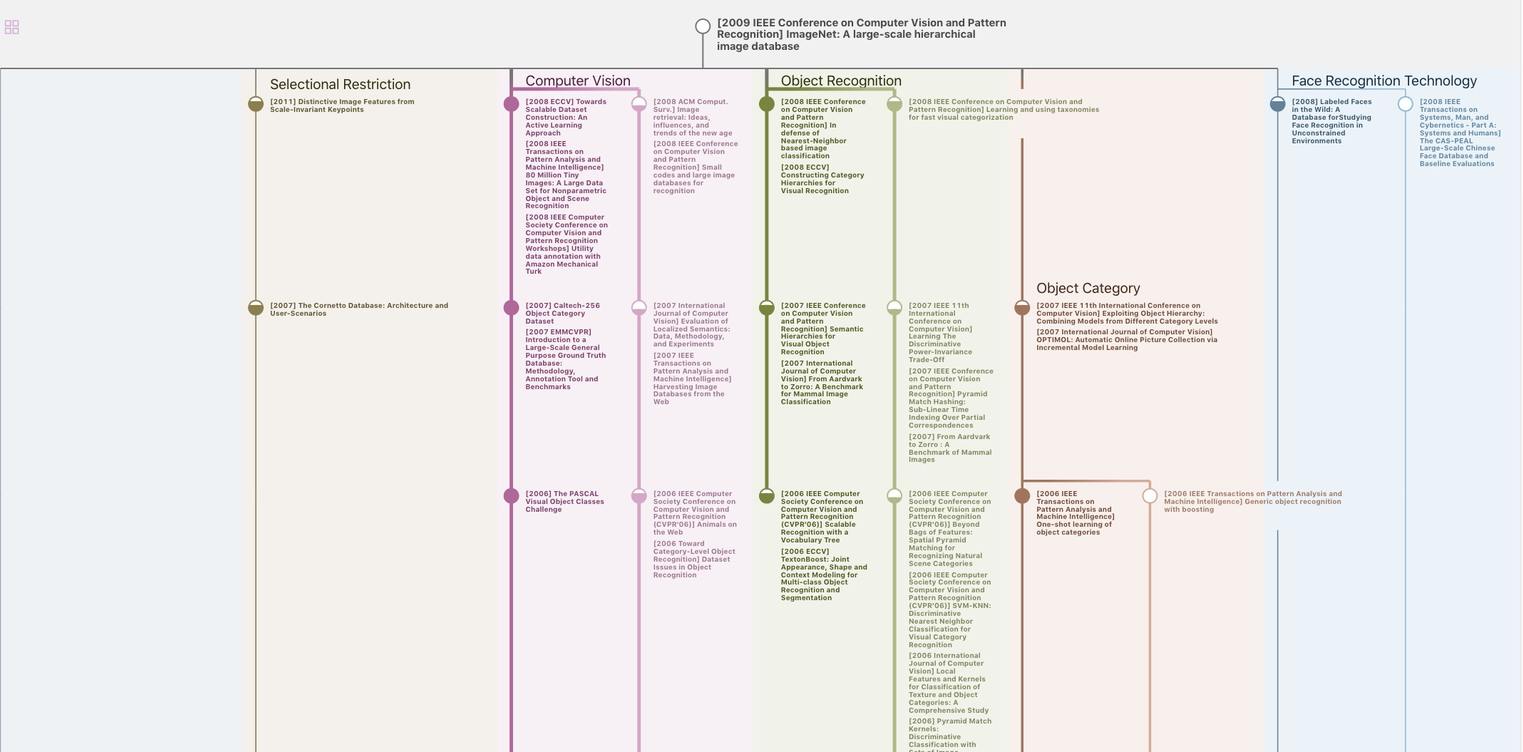
Generate MRT to find the research sequence of this paper
Chat Paper
Summary is being generated by the instructions you defined