Resting-state aperiodic neural activity as a novel objective marker of daytime somnolence
bioRxiv (Cold Spring Harbor Laboratory)(2022)
摘要
Study Objectives Current assessment of excessive daytime somnolence (EDS) requires subjective measurements such as the Epworth Sleepiness Scale (ESS), and/or resource intensive sleep laboratory investigations. Recent work [1][1],[2][2] has called for more non-performance-based measures of EDS. One promising non-performance-based measure of EDS is the aperiodic component of electroencephalography (EEG). Aperiodic (non-oscillatory) activity reflects excitation/inhibition ratios of neural populations and is altered in various states of consciousness, and thus may be a potential biomarker of hypersomnolence.
Methods We retrospectively analysed EEG data from patients who underwent a Multiple Sleep Latency Test (MSLT) and determined whether aperiodic neural activity is predictive of EDS. Participants having undergone laboratory polysomnogram and next day MSLT were grouped into MSLT+ ( n = 26) and MSLT– ( n = 33) groups (mean sleep latency of < 8min and > 10min, respectively) and compared against a non-clinical (Control) group of participants ( n = 26).
Results While the MSLT+ and MSLT– groups did not differ in their aperiodic activity, the Control group had a significantly flatter slope and larger offset compared to both MSLT+ and MSLT– groups. Logistic regression machine learning predicted group status (i.e., symptomatic, non-symptomatic) with 90% accuracy based on the aperiodic slope while controlling for age. Slow oscillation-spindle coupling was also significantly stronger in the Control group relative to MSLT+ and MSLT– groups.
Conclusions Our results provide first evidence that aperiodic neural dynamics and sleep-based cross-frequency coupling is predictive of EDS, thereby providing a novel avenue for basic and applied research in the study of sleepiness.
### Competing Interest Statement
The authors have declared no competing interest.
[1]: #ref-1
[2]: #ref-2
更多查看译文
AI 理解论文
溯源树
样例
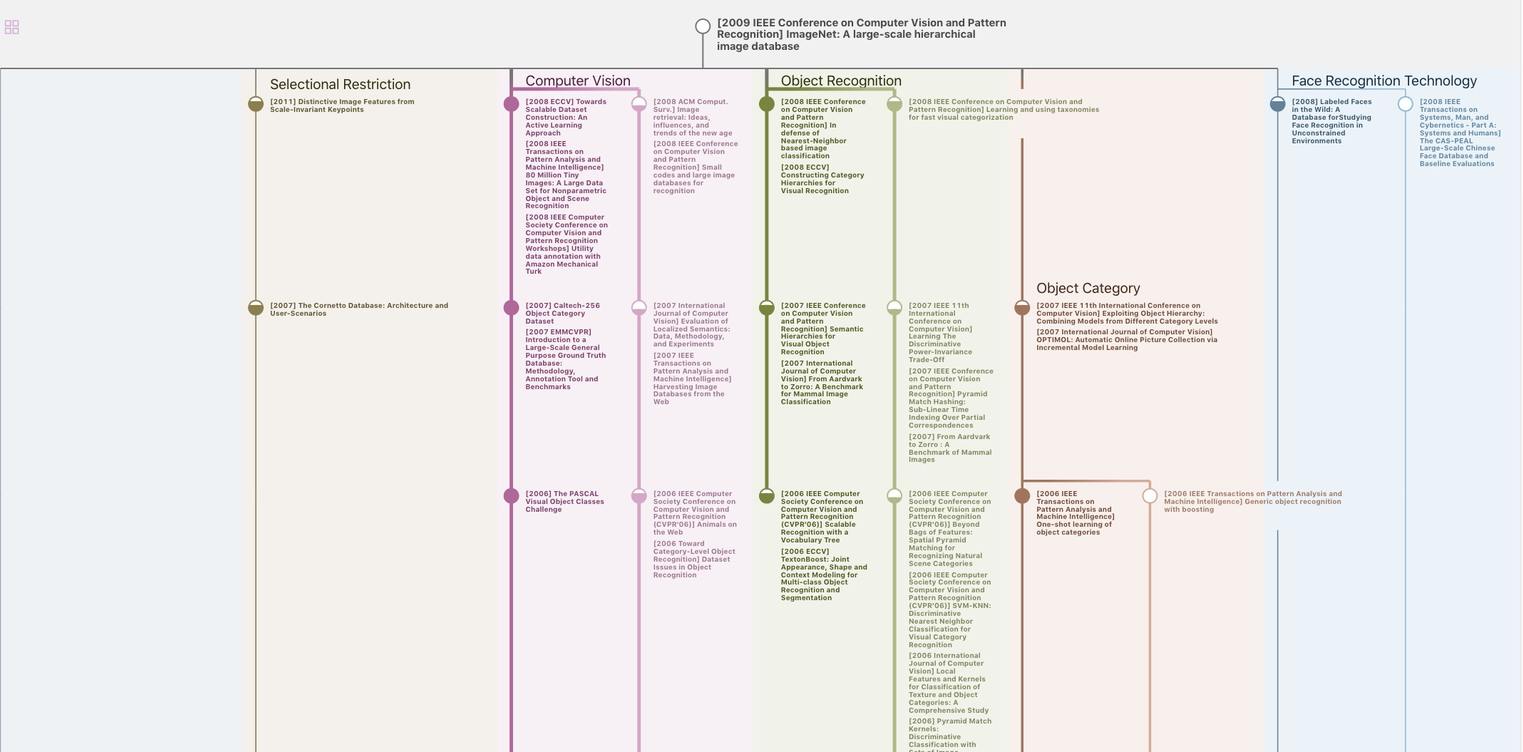
生成溯源树,研究论文发展脉络
Chat Paper
正在生成论文摘要