A comprehensive benchmark for COVID-19 predictive modeling using electronic health records in intensive care
Patterns(2024)
摘要
The COVID-19 pandemic highlighted the need for predictive deep-learning models in health care. However, practical prediction task design, fair comparison, and model selection for clinical applications remain a challenge. To address this, we introduce and evaluate two new prediction tasks—outcome-specific length-of-stay and early-mortality prediction for COVID-19 patients in intensive care—which better reflect clinical realities. We developed evaluation metrics, model adaptation designs, and open-source data preprocessing pipelines for these tasks while also evaluating 18 predictive models, including clinical scoring methods and traditional machine-learning, basic deep-learning, and advanced deep-learning models, tailored for electronic health record (EHR) data. Benchmarking results from two real-world COVID-19 EHR datasets are provided, and all results and trained models have been released on an online platform for use by clinicians and researchers. Our efforts contribute to the advancement of deep-learning and machine-learning research in pandemic predictive modeling.
更多查看译文
关键词
electronic health record,EHR,COVID-19,benchmark,deep learning,intensive care unit,ICU,mortality prediction,length-of-stay prediction
AI 理解论文
溯源树
样例
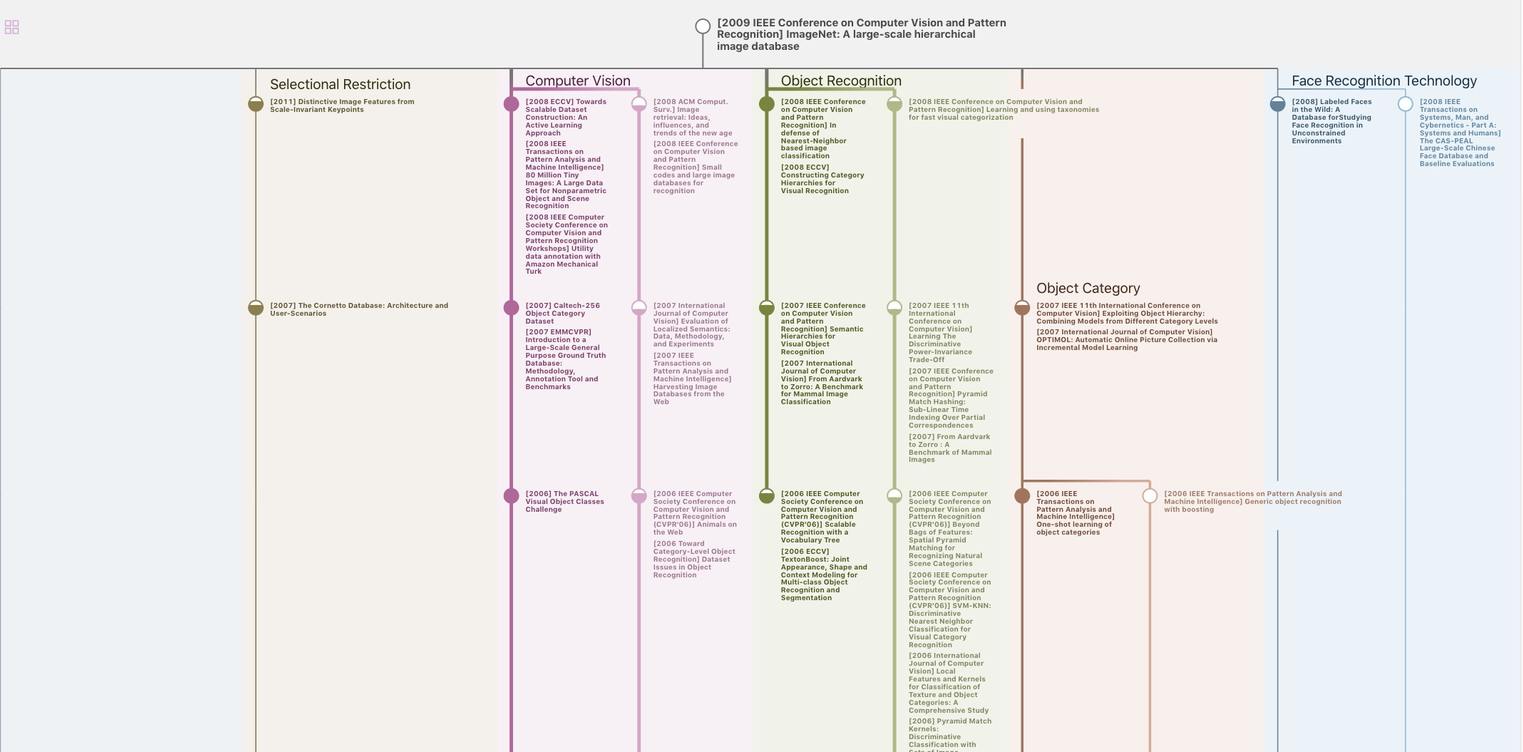
生成溯源树,研究论文发展脉络
Chat Paper
正在生成论文摘要