Dynamics-informed deconvolutional neural networks for super-resolution identification of regime changes in epidemiological time series
arxiv(2022)
摘要
Inferring the timing and amplitude of perturbations in epidemiological systems from their stochastically spread low-resolution outcomes is as relevant as challenging. It is a requirement for current approaches to overcome the need to know the details of the perturbations to proceed with the analyses. However, the general problem of connecting epidemiological curves with the underlying incidence lacks the highly effective methodology present in other inverse problems, such as super-resolution and dehazing from computer vision. Here, we develop an unsupervised physics-informed convolutional neural network approach in reverse to connect death records with incidence that allows the identification of regime changes at single-day resolution. Applied to COVID-19 data with proper regularization and model-selection criteria, the approach can identify the implementation and removal of lockdowns and other nonpharmaceutical interventions with 0.93-day accuracy over the time span of a year.
更多查看译文
关键词
epidemiological time series,deconvolutional neural networks,time series,neural networks,dynamics-informed,super-resolution
AI 理解论文
溯源树
样例
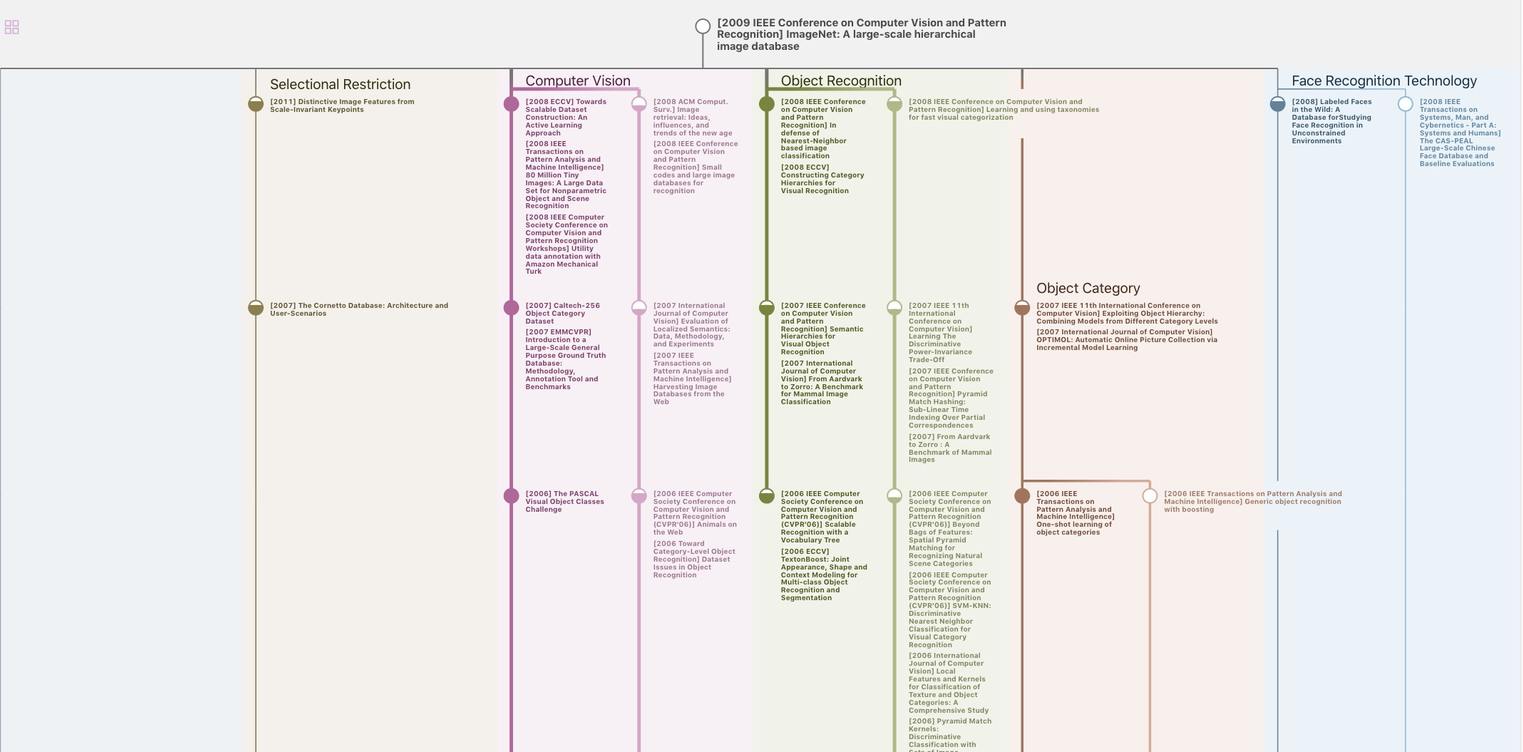
生成溯源树,研究论文发展脉络
Chat Paper
正在生成论文摘要