Hybrid Window Attention Based Transformer Architecture for Brain Tumor Segmentation
International Workshop on Brainlesion Glioma, Multiple Sclerosis, Stroke and Traumatic Brain Injuries(2022)
摘要
As intensities of MRI volumes are inconsistent across institutes, it is essential to extract universal features of multi-modal MRIs to precisely segment brain tumors. In this concept, we propose a volumetric vision transformer that follows two windowing strategies in attention for extracting fine features and local distributional smoothness (LDS) during model training inspired by virtual adversarial training (VAT) to make the model robust. We trained and evaluated network architecture on the FeTS Challenge 2022 dataset. Our performance on the online validation dataset is as follows: Dice Similarity Score of 81.71%, 91.38% and 85.40%; Hausdorff Distance (95%) of 14.81 mm, 3.93 mm, 11.18 mm for the enhancing tumor, whole tumor, and tumor core, respectively. Overall, the experimental results verify our method's effectiveness by yielding better performance in segmentation accuracy for each tumor sub-region. Our code implementation is publicly available : https://github.com/himashi92/vizviva_fets_2022
更多查看译文
关键词
brain tumor
AI 理解论文
溯源树
样例
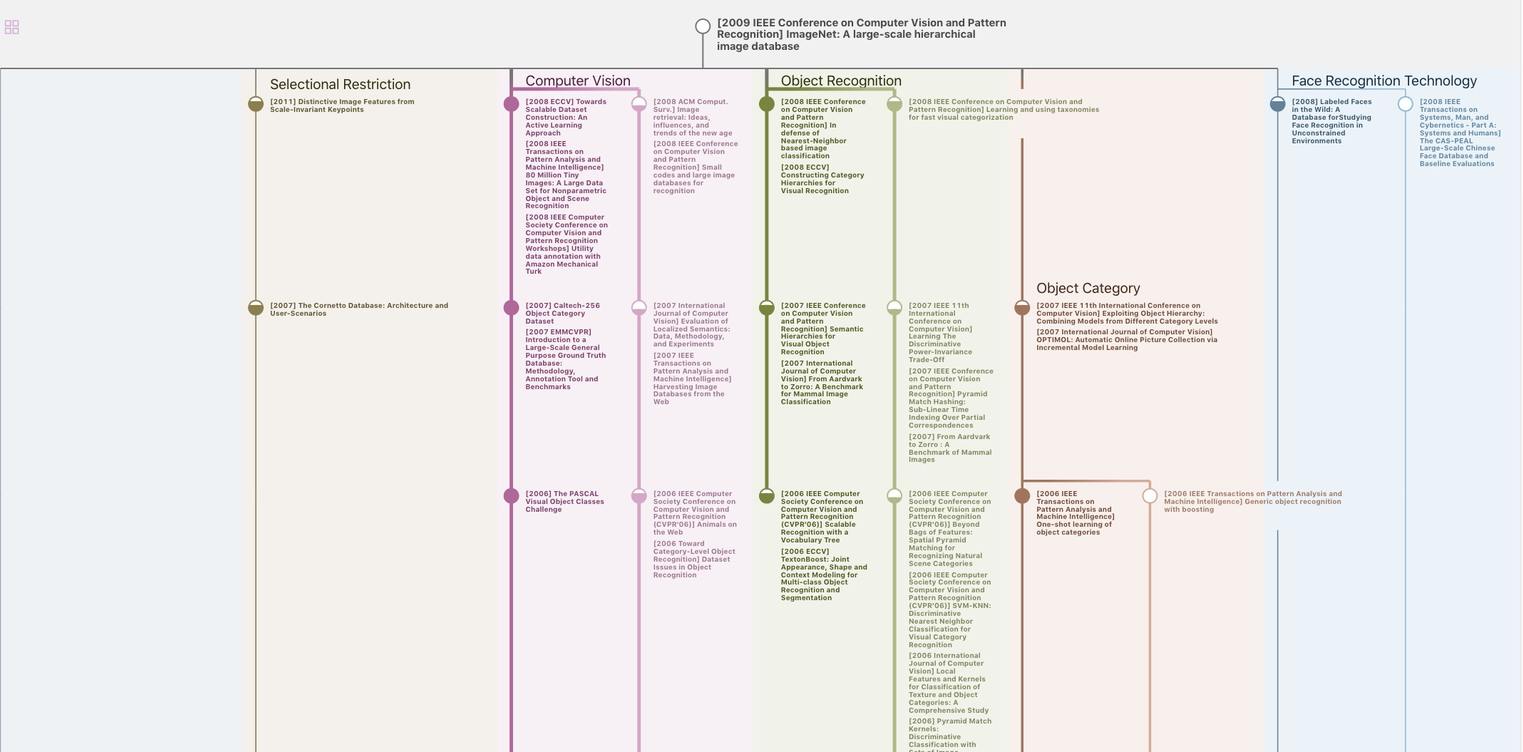
生成溯源树,研究论文发展脉络
Chat Paper
正在生成论文摘要