Scalable computation of energy functions for nonlinear balanced truncation
CURRENT OPINION IN CHEMICAL ENGINEERING(2024)
摘要
Nonlinear balanced truncation is a model order reduction technique that reduces the dimension of nonlinear systems in a manner that accounts for either open- or closed -loop observability and controllability aspects of the system. A computational challenges that has so far prevented its deployment on large-scale systems is that the energy functions required for characterization of controllability and observability are solutions of various high -dimensional Hamilton-Jacobi- (Bellman) equations, which are computationally intractable in high dimensions. This work proposes a unifying and scalable approach to this challenge by considering a Taylor -series -based approximation to solve a class of parametrized Hamilton-Jacobi-Bellman equations that are at the core of nonlinear balancing. The value of a formulation parameter provides either open -loop balancing or a variety of closed -loop balancing options. To solve for the coefficients of Taylorseries approximations to the energy functions, the presented method derives a linear tensor system and heavily utilizes it to numerically solve structured linear systems with billions of unknowns. The strength and scalability of the algorithm is demonstrated on two semi-discretized partial differential equations, namely the Burgers and the Kuramoto-Sivashinsky equations.
更多查看译文
关键词
Reduced-order modeling,Balanced truncation,Nonlinear manifolds,Hamilton-Jacobi-Bellman equation,Nonlinear systems
AI 理解论文
溯源树
样例
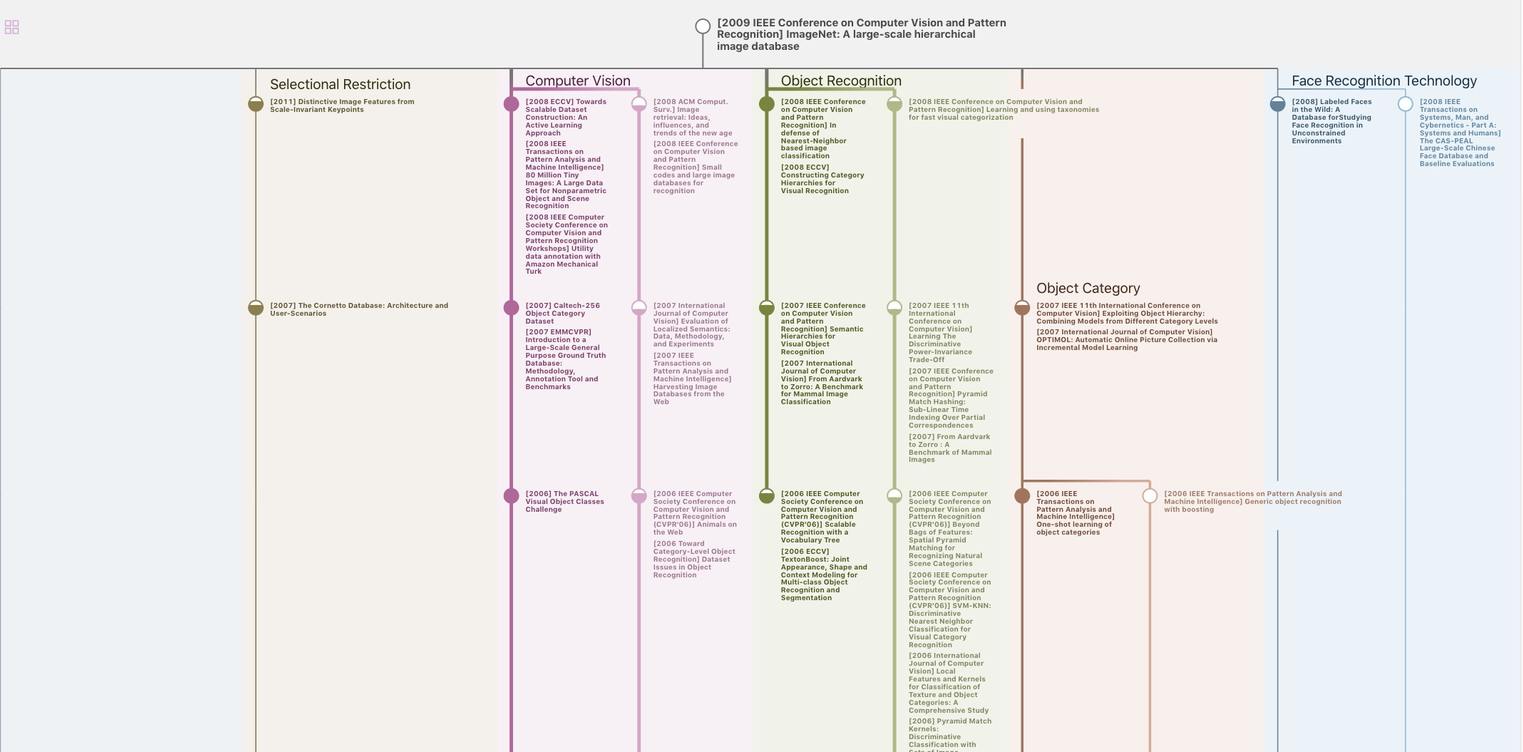
生成溯源树,研究论文发展脉络
Chat Paper
正在生成论文摘要