Cell-type-directed design of synthetic enhancers
Nature(2024)
摘要
Transcriptional enhancers act as docking stations for combinations of transcription factors and thereby regulate spatiotemporal activation of their target genes 1 . It has been a long-standing goal in the field to decode the regulatory logic of an enhancer and to understand the details of how spatiotemporal gene expression is encoded in an enhancer sequence. Here we show that deep learning models 2 – 6 , can be used to efficiently design synthetic, cell-type-specific enhancers, starting from random sequences, and that this optimization process allows detailed tracing of enhancer features at single-nucleotide resolution. We evaluate the function of fully synthetic enhancers to specifically target Kenyon cells or glial cells in the fruit fly brain using transgenic animals. We further exploit enhancer design to create ‘dual-code’ enhancers that target two cell types and minimal enhancers smaller than 50 base pairs that are fully functional. By examining the state space searches towards local optima, we characterize enhancer codes through the strength, combination and arrangement of transcription factor activator and transcription factor repressor motifs. Finally, we apply the same strategies to successfully design human enhancers, which adhere to enhancer rules similar to those of Drosophila enhancers. Enhancer design guided by deep learning leads to better understanding of how enhancers work and shows that their code can be exploited to manipulate cell states.
更多查看译文
关键词
cell type
AI 理解论文
溯源树
样例
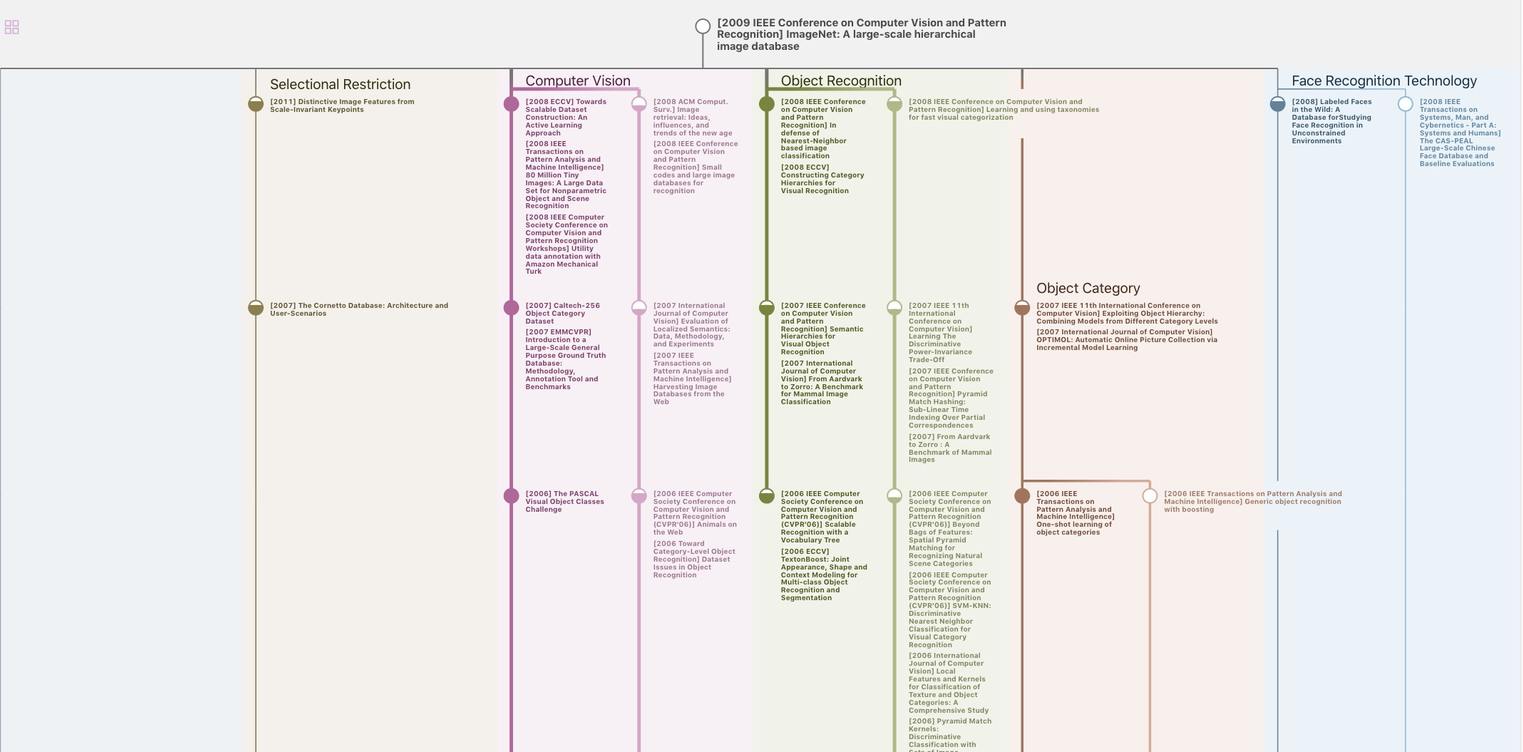
生成溯源树,研究论文发展脉络
Chat Paper
正在生成论文摘要