IMC-Denoise: a Content Aware Denoising Pipeline to Enhance Imaging Mass Cytometry
Nature Communications(2023)
关键词
Diagnostic markers,Histology,Imaging the immune system,Science,Humanities and Social Sciences,multidisciplinary
AI 理解论文
溯源树
样例
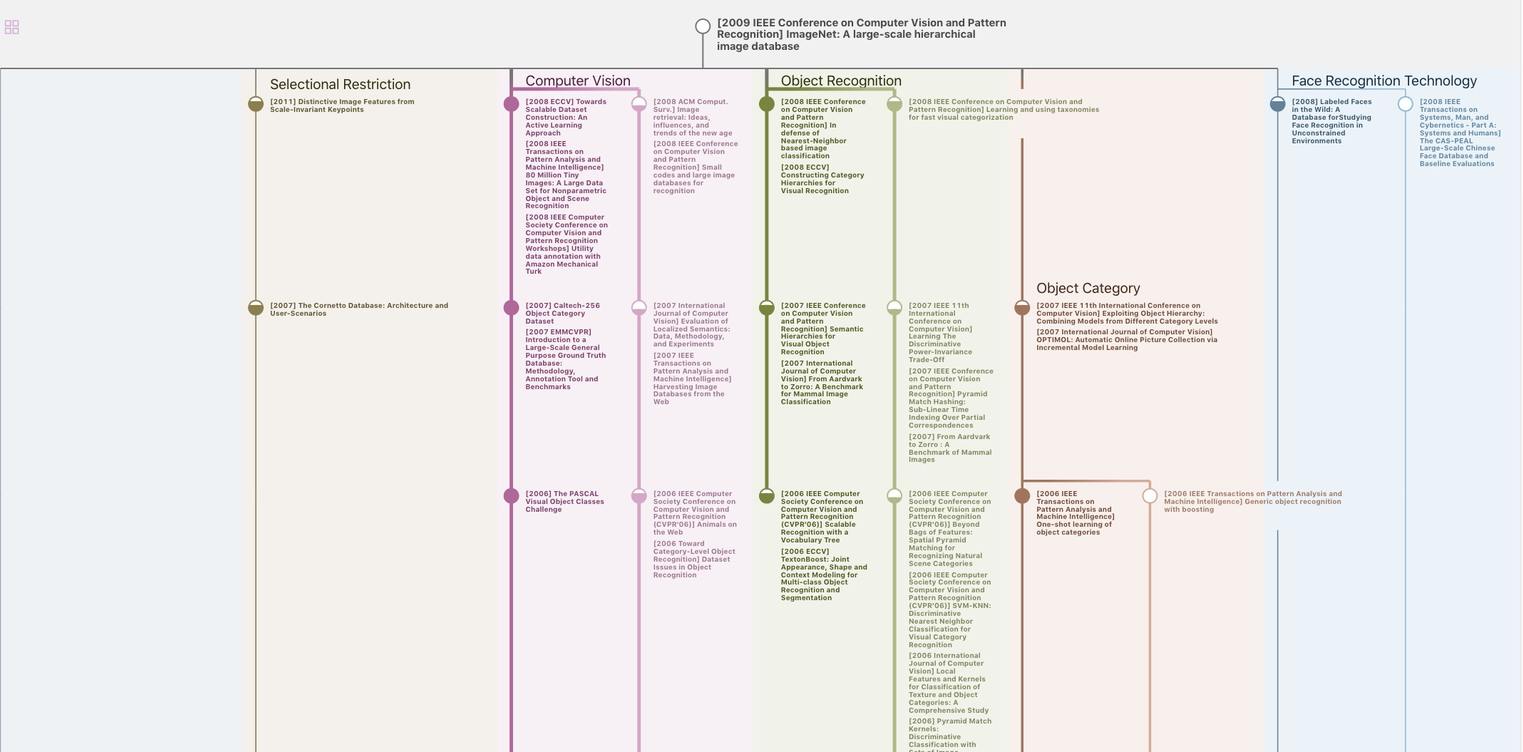
生成溯源树,研究论文发展脉络
Chat Paper
正在生成论文摘要