Selection of an Ideal Machine Learning Framework for Predicting Perturbation Effects on Network Topology of Bacterial KEGG Pathways
biorxiv(2022)
摘要
Biological networks for bacterial species are used to assign functional information to newly sequenced organisms but network quality can be largely affected by poor gene annotations. Current methods of gene annotation use homologous alignment to determine orthology, and have been shown to degrade network accuracy in non-model bacterial species. To address these issues in the KEGG pathway database, we investigated the ability for machine learning (ML) algorithms to re-annotate bacterial genes based on motif or homology information. The majority of the ensemble, clustering, and deep learning algorithms that we explored showed higher prediction accuracy than CD-hit in predicting EC ID, Map ID, and partial Map ID. Motif-based, machine-learning methods of annotation in new species were more accurate, faster, and had higher precisionrecall than methods of homologous alignment or orthologous gene clustering. Gradient boosted ensemble methods and neural networks also predicted higher connectivity of networks, finding twice as many new pathway interactions than blast alignment. The use of motif-based, machine-learning algorithms in annotation software will allow researchers to develop powerful network tools to interact with bacterial microbiomes in ways previously unachievable through homologous sequence alignment.
CCS CONCEPTS • Applied computing → Computational biology ; Life and medical sciences ; Bioinformatics ; • Computing methodologies → Machine learning algorithms ; Machine learning approaches .
ACM Reference Format Michael Robben, Mohammad Sadegh Nasr, Avishek Das, Manfred Huber, Justyn Jaworski, Jon Weidanz, and Jacob Luber. 2022. Selection of an Ideal Machine Learning Framework for Predicting Perturbation Effects on Network Topology of Bacterial KEGG Pathways. In The 13th ACM Conference on Bioinformatics, Computational Biology, and Health Informatics, August 07–10, 2022, Chicago, IL . ACM, New York, NY, USA, 11 pages.
### Competing Interest Statement
The authors have declared no competing interest.
更多查看译文
关键词
pathways,network topology,ideal machine learning framework,machine learning
AI 理解论文
溯源树
样例
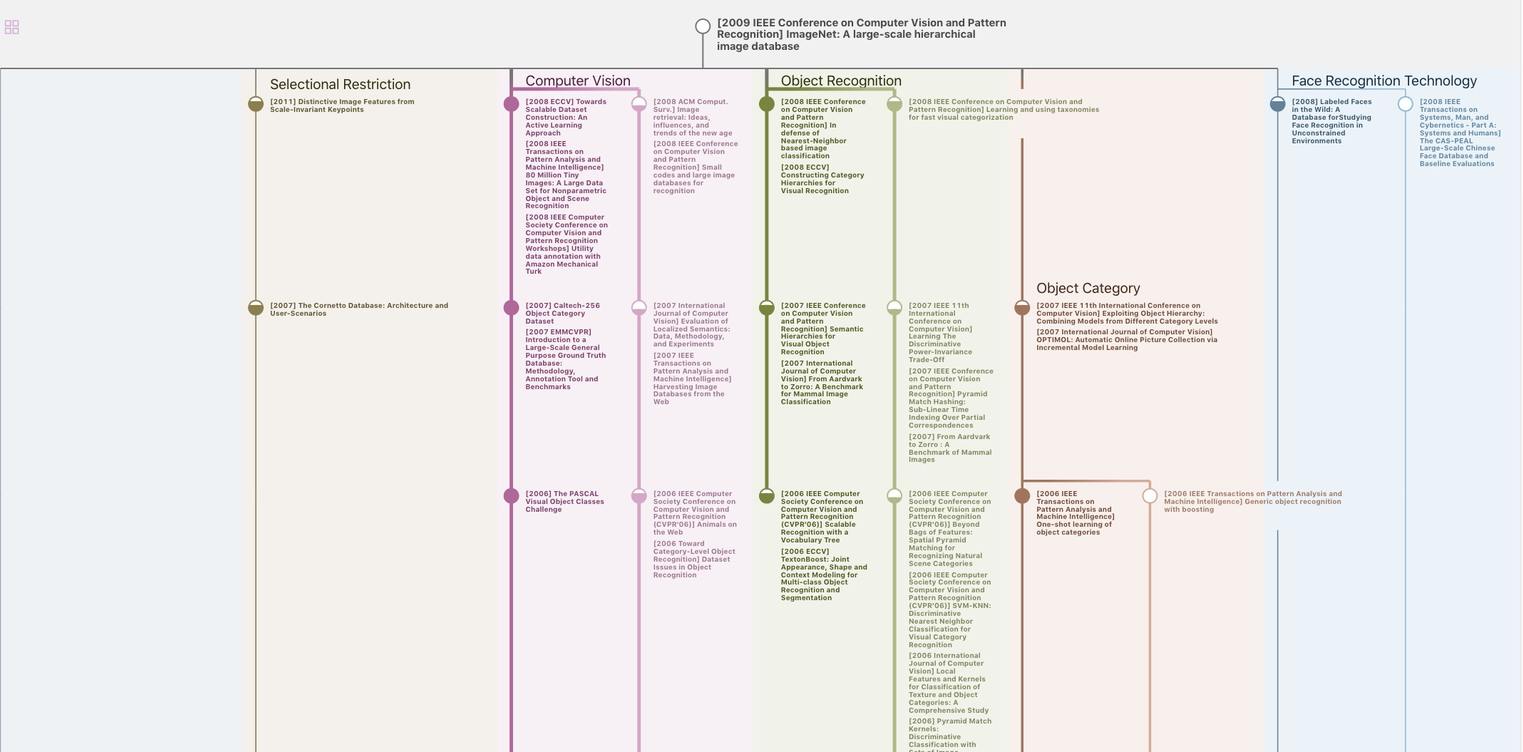
生成溯源树,研究论文发展脉络
Chat Paper
正在生成论文摘要