Cross-Modality and Self-Supervised Protein Embedding for Compound–Protein Affinity and Contact Prediction
Bioinformatics(2022)
摘要
Motivation Computational methods for compound–protein affinity and contact (CPAC) prediction aim at facilitating rational drug discovery by simultaneous prediction of the strength and the pattern of compound–protein interactions. Although the desired outputs are highly structure-dependent, the lack of protein structures often makes structure-free methods rely on protein sequence inputs alone. The scarcity of compound–protein pairs with affinity and contact labels further limits the accuracy and the generalizability of CPAC models.
Results To overcome the aforementioned challenges of structure naivety and labelled-data scarcity, we introduce cross-modality and self-supervised learning, respectively, for structure-aware and task-relevant protein embedding. Specifically, protein data are available in both modalities of 1D amino-acid sequences and predicted 2D contact maps, that are separately embedded with recurrent and graph neural networks, respectively, as well as jointly embedded with two cross-modality schemes. Furthermore, both protein modalities are pretrained under various self-supervised learning strategies, by leveraging massive amount of unlabelled protein data. Our results indicate that individual protein modalities differ in their strengths of predicting affinities or contacts. Proper cross-modality protein embedding combined with self-supervised learning improves model generalizability when predicting both affinities and contacts for unseen proteins.
Availability Data and source codes are available at .
Contact yshen{at}tamu.edu
Supplementary information Supplementary data are included.
### Competing Interest Statement
The authors have declared no competing interest.
更多查看译文
AI 理解论文
溯源树
样例
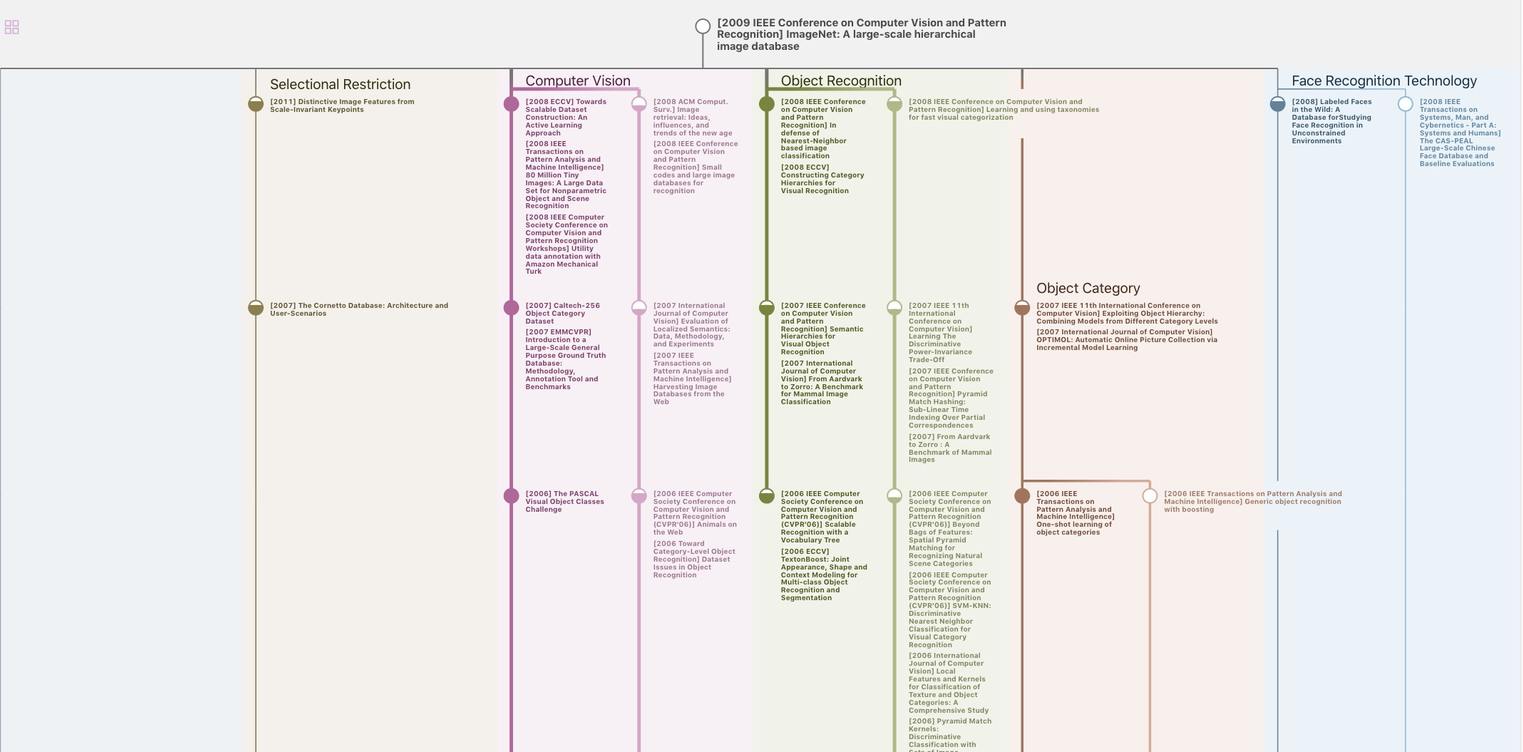
生成溯源树,研究论文发展脉络
Chat Paper
正在生成论文摘要