System identification reveals multiple interacting states in visuomotor adaptation
biorxiv(2022)
摘要
Many characteristics of sensorimotor adaptation are well predicted by the Kalman filter, a relatively simple learning algorithm for the optimal estimation of dynamic variables given noisy measurements. Yet not all Kalman filter predictions are confirmed empirically, suggesting that the model might not be sufficient to describe the observed behavior. In our study, we propose that sensorimotor adaptation can be modeled with multiple interacting states, each one described as a Kalman filter, to better reflect the architecture plant of the physical system implementing the behavior (i.e., the different motor and sensory components involved in adaptation). To test our hypothesis, we measured motor error in a series of rapid reaching tasks in which we introduced different conditions of feedback uncertainty and systematic perturbations. We then applied system identification procedures to the resulting adaptation response to test which system architecture would best fit the data. We considered three possible architectures: one with a single Kalman filter, with two filters in series, or two filters in parallel. We found that this latter structure consistently provided a significantly better fit than the others. When evaluating the identified system parameters, we found that the learning rates of both states decreased under higher uncertainty, while the weight assigned to the slower state increased. Moreover, the dual-state parallel system accounted for the presence of a constant residual bias in the adaptation to a step offset, which has been repeatedly reported but is in disaccord with the predictions of the single Kalman filter. We propose that the identified system architecture reflects how the error is assigned to different components of the physical plant responsible for adaptation: namely, as uncertainty increases, the controller assigns a larger contribution in error reduction to the slower state, which is more likely to have been responsible for the measured error.
### Competing Interest Statement
The authors have declared no competing interest.
更多查看译文
关键词
system,identification
AI 理解论文
溯源树
样例
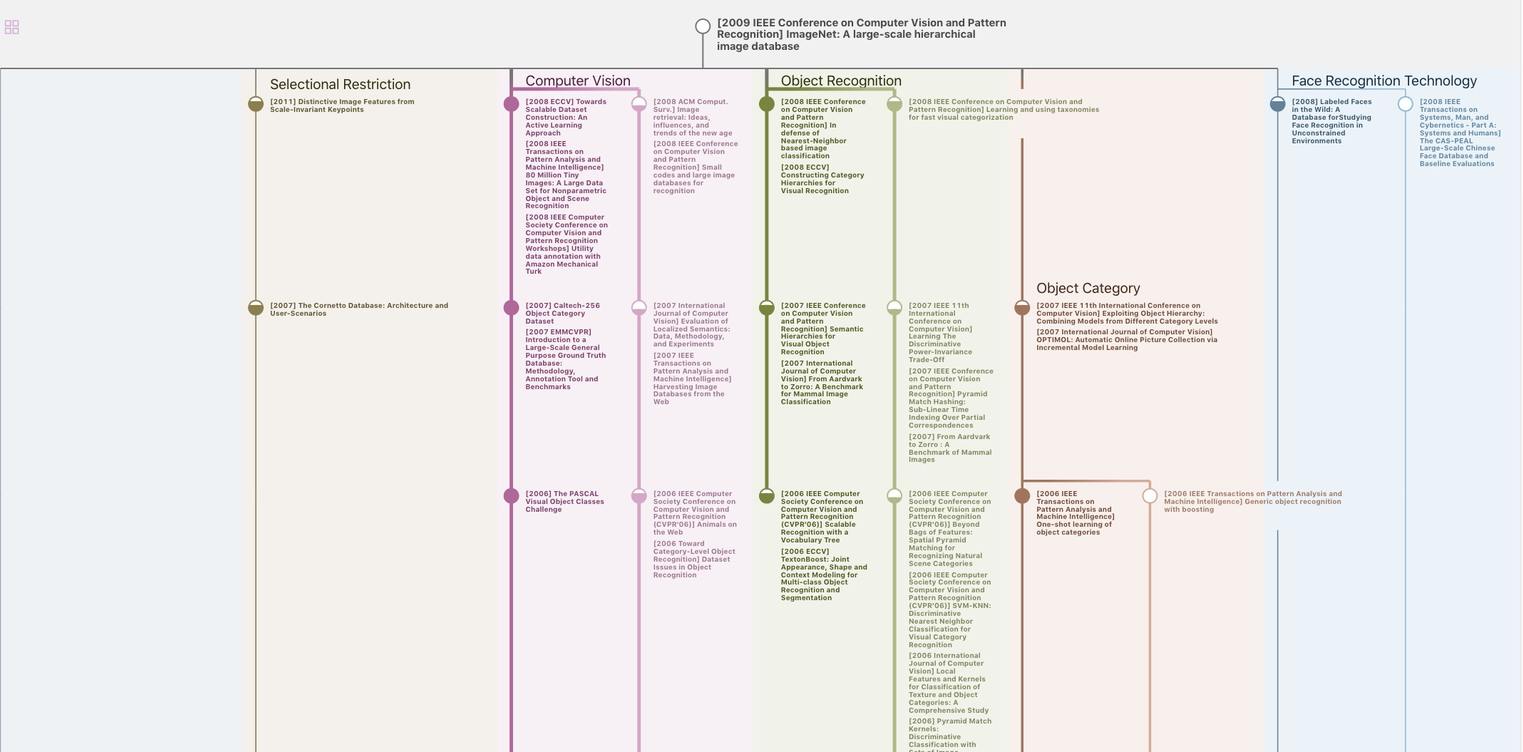
生成溯源树,研究论文发展脉络
Chat Paper
正在生成论文摘要