Fully Convolutional Network for Breast Lesion Segmentation in Ultrasound Image: Towards False Positive Reduction
MULTIMODAL IMAGE EXPLOITATION AND LEARNING 2022(2022)
摘要
Ultrasound scan (US) imagery is an important tool for radiologists to make a fast and reliable diagnosis decision about breast lesion status (benign or malignant). Accurate and automatic segmentation of breast lesion is critical for annotating the lesion characteristics such as margin smoothness and regularity in support of the diagnosis decision. Fully convolutional network (FCN) is one of the commonly used deep learning neural network methods for semantic segmentation. This paper is concerned with effective adaptation of the FCN solutions for segmenting breast lesions from 2D ultrasound images. The paper aims to first evaluate the existing FCN solution for solving the problem at hand and compare its performance with another popular method using U-Net. The paper then highlights one key issue with the FCN, i.e. false positive pixels near the boundary of a lesion and false positive pixels forming false lesions. The paper then investigates several methods in reducing such false positive pixels, including the use of data augmentation in training the classification model and use of loss functions in training the models. Experimental results using several data sets collected from various sources show that our adapted FCN method outperforms U-Net-based solutions in general and the false positive reduction methods we attempted have reduced the false positive pixels in both regions close to lesion boundary and separate from true lesion regions.
更多查看译文
关键词
Breast Lesion, Ultrasound Image, Semantic Segmentation, FCN, False Positive Reduction, Data Augmentation, Loss Function
AI 理解论文
溯源树
样例
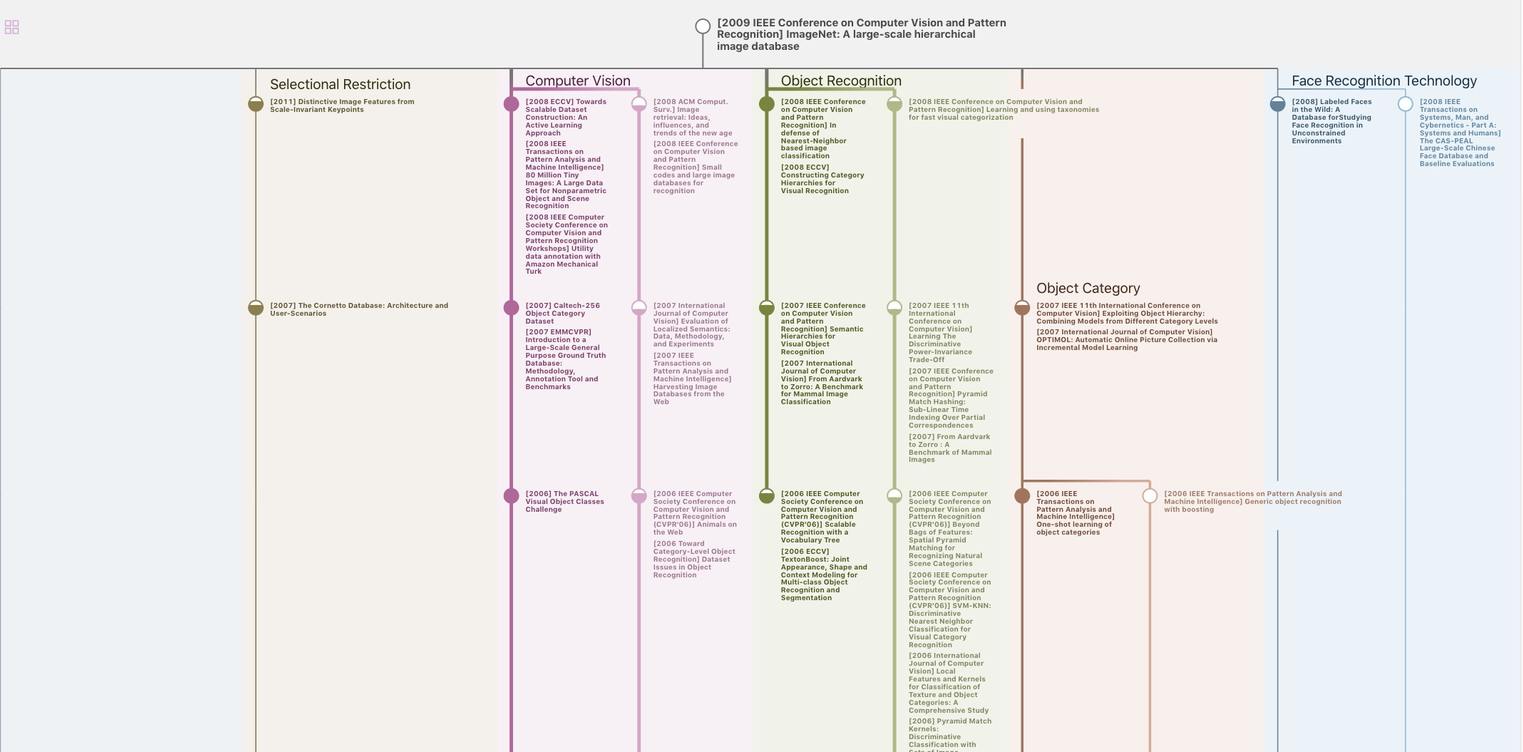
生成溯源树,研究论文发展脉络
Chat Paper
正在生成论文摘要