Gaze-FTNet: A feature transverse architecture for predicting gaze attention
MULTIMODAL IMAGE EXPLOITATION AND LEARNING 2022(2022)
摘要
The dynamics of gaze coordination in natural contexts are affected by various properties of the task, the agent, the environment, and their interaction. Artificial Intelligence (AI) lays the foundation for detection, classification, segmentation, and scene analysis. Much of AI in everyday use is dedicated to predicting people's behavior. However, a purely data-driven approach cannot solve development problems alone. Therefore, it is imperative that decision-makers also consider another AI approach-causal AI, which can help identify the precise relationships of cause and effect. This article presents a novel Gaze Feature Transverse Network (Gaze-FTNet) that generates close-to-human gaze attention. The proposed end-to-end trainable approach leverages a feature transverse network (FTNet) to model long-term dependencies for optimal saliency map prediction. Moreover, several modern backbone architectures are explored, tested, and analyzed. Synthetically predicting human attention from monocular RGB images will benefit several domains, particularly human-vehicle interaction, autonomous driving, and augmented reality.
更多查看译文
关键词
gaze, saliency, vision, convolution, eye-tracking, fixation, metaverse, augmented reality
AI 理解论文
溯源树
样例
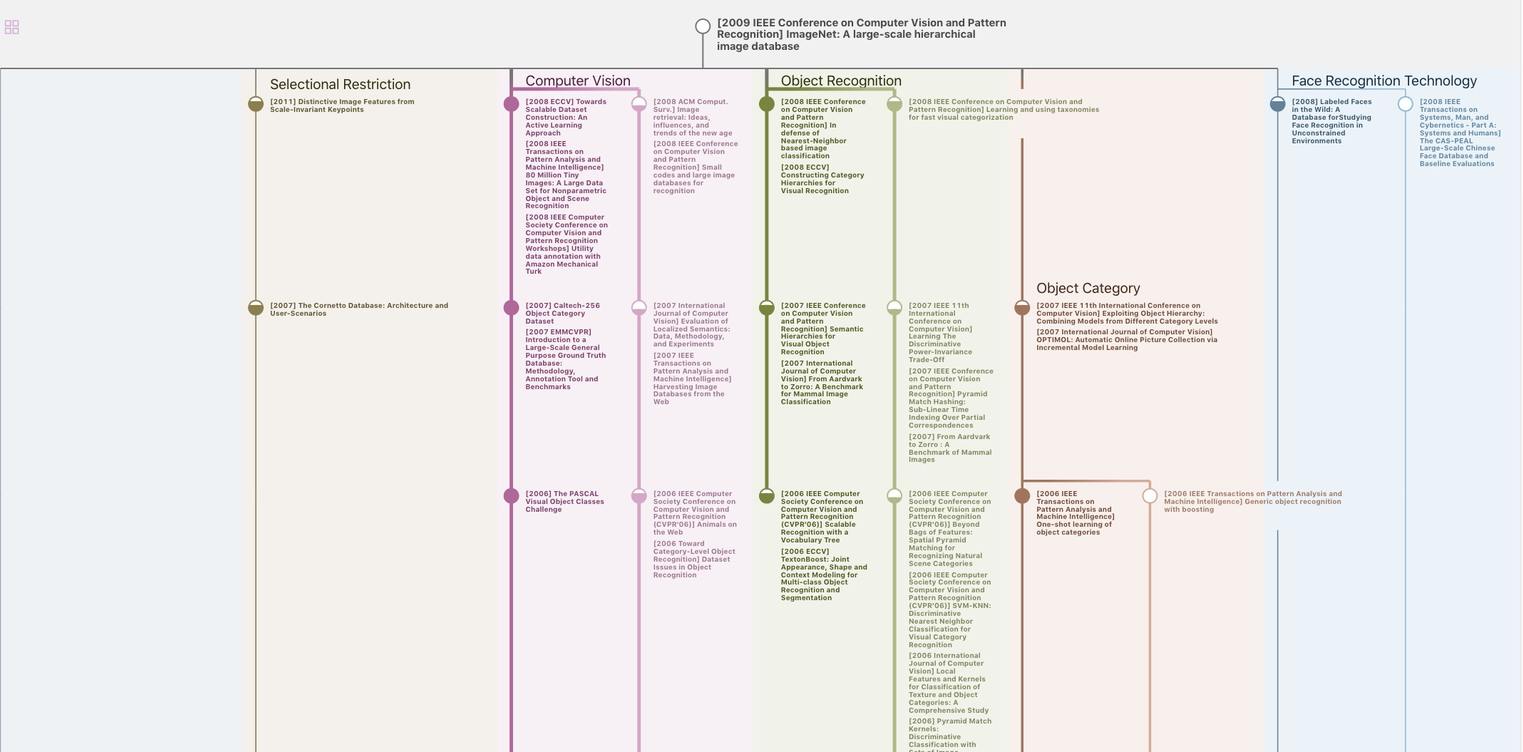
生成溯源树,研究论文发展脉络
Chat Paper
正在生成论文摘要