Attention-Based Two-Stream High-Resolution Networks for Building Damage Assessment from Satellite Imagery
MULTIMODAL IMAGE EXPLOITATION AND LEARNING 2022(2022)
摘要
Satellite imagery provides an efficient means of assessing and effectively planning search and rescue efforts in the aftermath of disasters such as earthquakes, flooding, tsunamis, wildfires, and conflicts. It enables timely visualization of buildings and the human population affected by these disasters and provides humanitarian organizations with crucial information needed to strategize and deliver the much need aid effectively. Recent research on remote sensing combines machine learning methodologies with satellite imagery to automate information extraction, thus reducing turn-around time and manual labor. The existing state-of-the-art approach for building damage assessment relies on an ensemble of different models to obtain independent predictions that are then aggregated to one final output. Other methods rely on a multi-stage model that involves a building localization module and a damage classification module. These methods are either not end-to-end trainable or are impractical for real-time applications. This paper proposes an Attention-based Two-Stream High-Resolution Network (ATS-HRNet), which unifies the building localization and classification problem in an end-to-end trainable manner. The basic residual blocks in HRNet are replaced with attention-based residual blocks to improve the model's performance. Furthermore, a modified cutmix data augmentation technique is introduced for handling class imbalance in satellite imagery. Experiments show that our approach significantly performs better than the baseline and other state-of-the-art methods for building damage classification.
更多查看译文
关键词
building damage assessment, convolutional neural network, deep learning, semi-supervised learning, xBD dataset, attention module, selective oversampling, pixel-aware cutmix
AI 理解论文
溯源树
样例
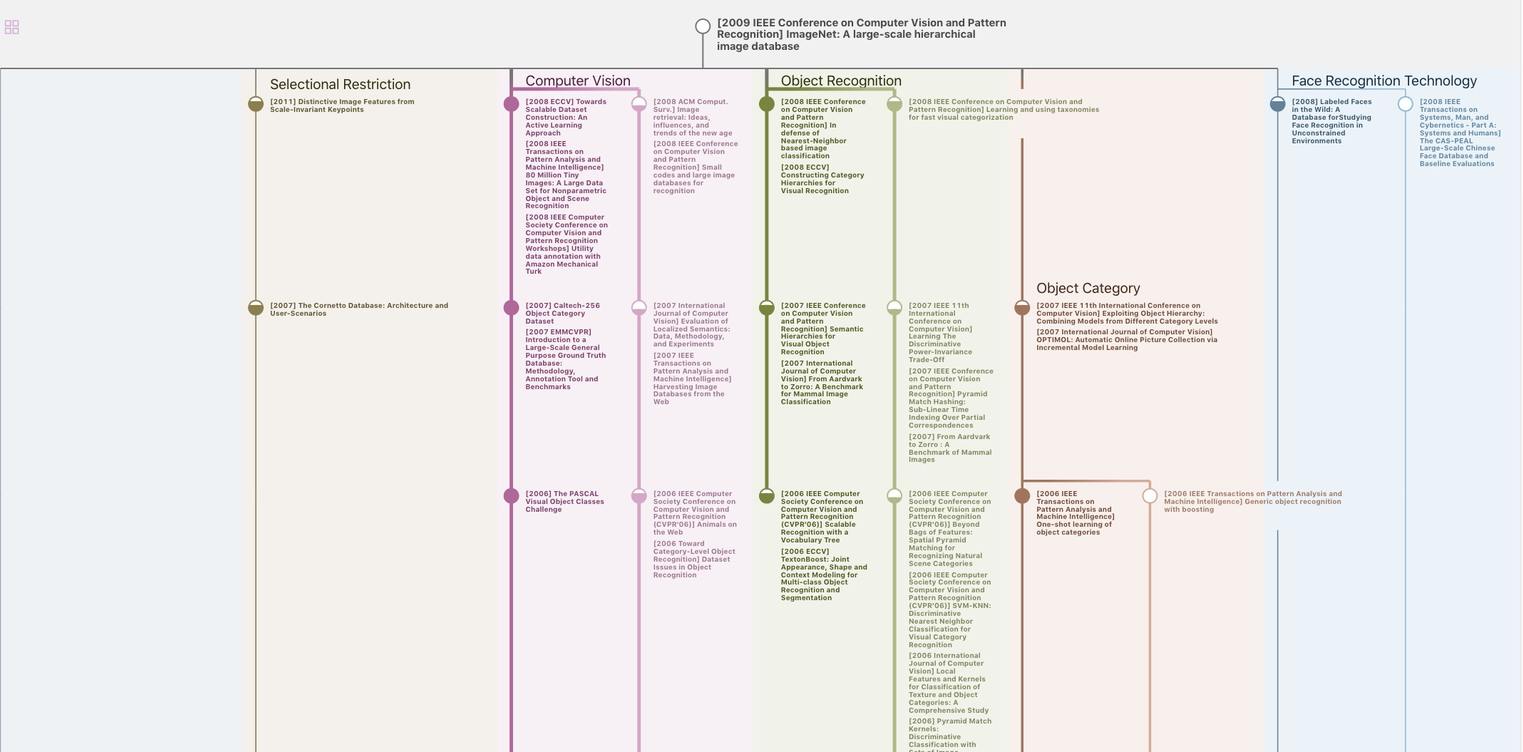
生成溯源树,研究论文发展脉络
Chat Paper
正在生成论文摘要