Brain Multi-parametric MRI Tumor Subregion Segmentation via Hierarchical Substructural Activation Network
MEDICAL IMAGING 2022: BIOMEDICAL APPLICATIONS IN MOLECULAR, STRUCTURAL, AND FUNCTIONAL IMAGING(2022)
摘要
Multiparameter magnetic resonance imaging (mp-MRI) commonly used modality for segmentation of glioma and its subregions, whereas RT therapy is a commonly used treatment modality. Current workflow includes manual segmentation of brain tumor sub-regions, which is a very lengthy and laborious process given that different sets of MR images have to be analyzed for an appropriate diagnosis. This work focuses on implementing and testing feasibility of a new deep learning model for an automatic segmentation of brain tumor sub-regions. Our proposed method, named hierarchical substructural activation network, consists of three main parts: a detection and segmentation module, and a hierarchical convolutional block. While the detection module is employed to detect the view-of-interests (VOIs) of brain tumor, which include all tumor sub-structures, the hierarchical convolutional block is used to derive structural activation map (SAM) to boost the classification accuracy between different structures. This is followed by the semantic segmentation of each substructure within the detected VOI by segmentation module. Brain tumor segmentation challenge (BraTS) 2020 dataset was used for evaluating our proposed framework. We performed five-fold cross validation experiments on 100 BraTS datasets. Three substructures, i.e., necrosis and non-enhancing, edema, enhancing tumor (ET), tumor core (TC), were segmented and compared with manual contours using the Dice similarity coefficient (DSC) and mean surface distance (MSD). In terms of segmentation of necrosis and non-enhancing subregions, edema, ET and TC, our method yielded DSC of 0.69 +/- 0.24, 0.89 +/- 0.09, 0.80 +/- 0.14, and 0.88 +/- 0.11, respectively, and MSD of 2.01 +/- 2.75, 0.63 +/- 0.58, 0.98 +/- 1.10 and 1.74 +/- 1.06 mm, respectively. Preliminary results of this work show promise to both accurately and automatically segment brain tumor subregions by our proposed method, providing motivation for its clinical implementation to improve clinical workflow.
更多查看译文
关键词
MRI, segmentation, deep learning
AI 理解论文
溯源树
样例
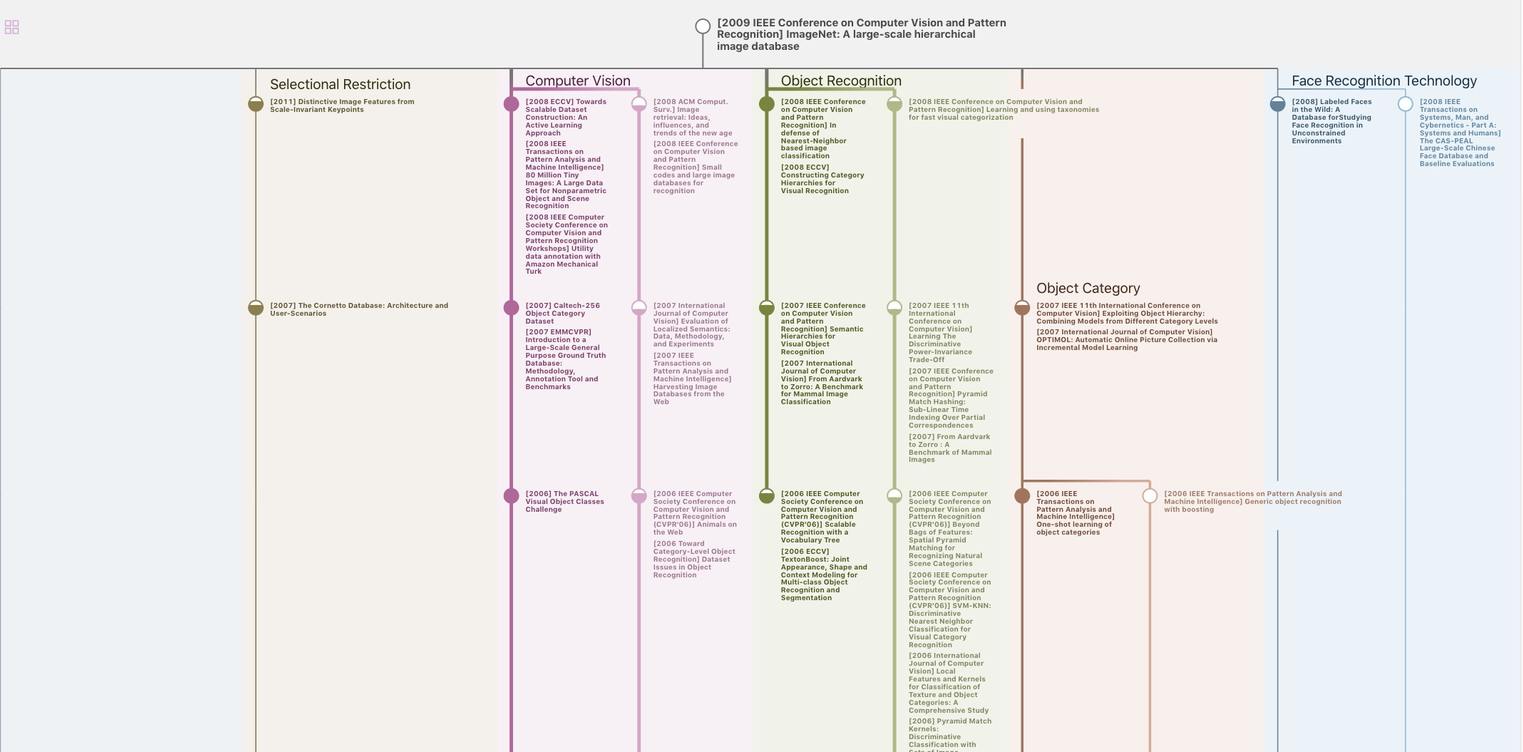
生成溯源树,研究论文发展脉络
Chat Paper
正在生成论文摘要