Slitless Field Spectroscopy for learned Simultaneous Object Detection and Recognition
ALGORITHMS, TECHNOLOGIES, AND APPLICATIONS FOR MULTISPECTRAL AND HYPERSPECTRAL IMAGING XXVIII(2022)
摘要
Effective space domain awareness (SDA) requires accurate positions and identities of artificial satellites. These measurements-critical to effective decision making in the high risk on orbit environment-are daunting in the deep space geosynchronous (GEO) regime. Here, distance precludes collection of spatially resolved measurements from ground-based telescopes. Neural networks designed for deep space object detection and spectroscopic positive identification have been shown to be effective tools for these mission critical SDA measurements. In this work we demonstrate the potential of slitless field spectroscopy to provide simultaneous object detection and identification of on orbit assets at GEO. Slitless spectrographs expose the reflection physics needed for spectroscopic positive identification without destroying the spatial information used for object detection. Such systems are compact and hardened in comparison to classic spectrographs, and may be deployed to small telescopes. In this work we present a GPU-accelerated simulation environment for the production of realistic synthetic imagery to support generation of large datasets for deep learning. We establish a baseline for simultaneous detection and identification performance by training convolutional neural networks on synthetic datasets created with this tool. This work reduces risk for initial technology development and dataset collection, and provides constraints to the design and development of slitless spectrograph systems for space domain awareness.
更多查看译文
关键词
Spectroscopy, space domain awareness, object detection, recognition
AI 理解论文
溯源树
样例
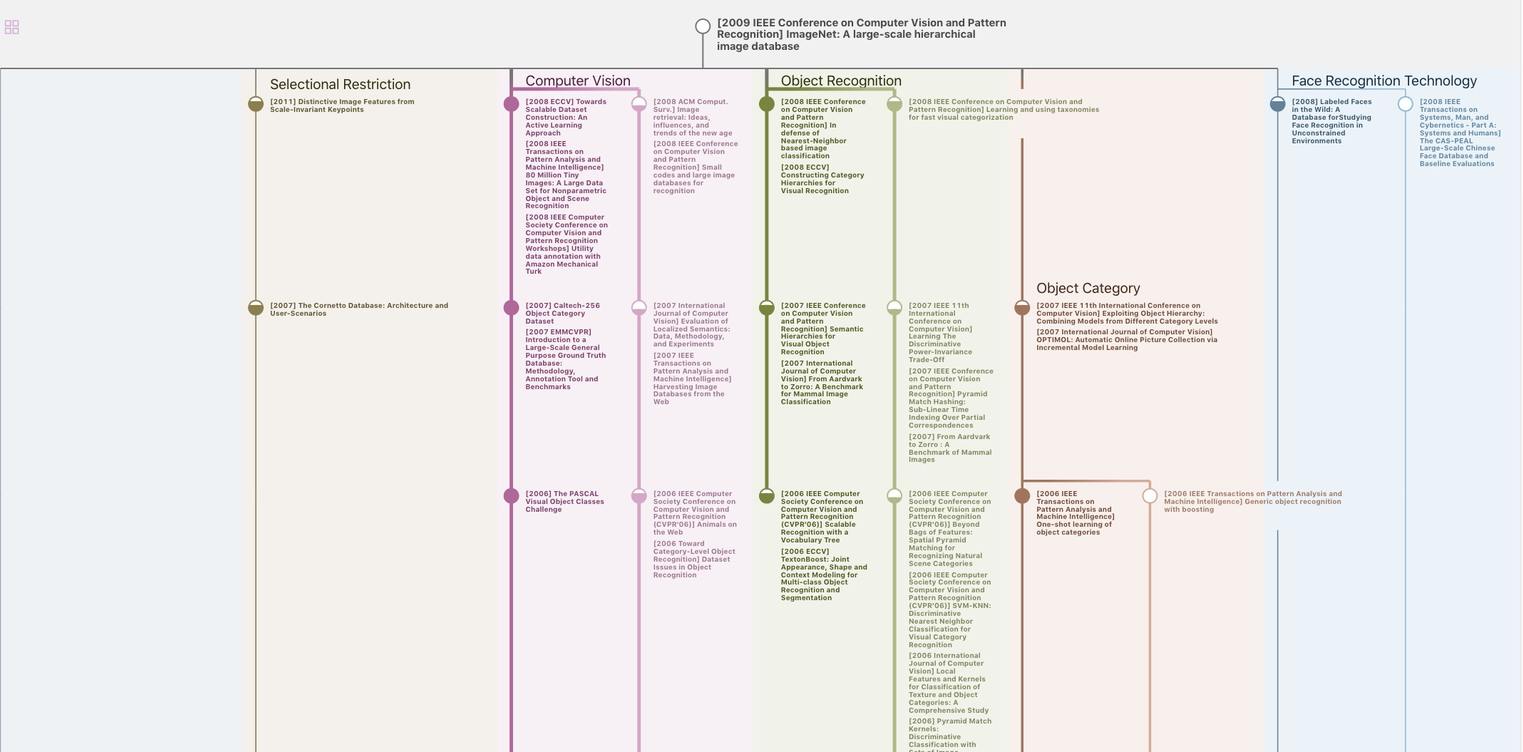
生成溯源树,研究论文发展脉络
Chat Paper
正在生成论文摘要