Development of Deep Learning Pipeline for Direct Observation of Wall Motion Abnormality from 4DCT
MEDICAL IMAGING 2022: BIOMEDICAL APPLICATIONS IN MOLECULAR, STRUCTURAL, AND FUNCTIONAL IMAGING(2022)
摘要
Detection of left ventricular (LV) wall motion abnormalities (WMA) from 4DCT by visual interpretation is challenging. Quantitative assessment requires complex computation on multiple frames with large data sizes. Volume Rendering (VR) of the LV in CT across the cardiac cycle can enable the evaluation of 3D wall motion with significantly reduced data size. We propose a deep-learning (DL) framework to automate WMA detection in volume-rendered videos of clinical 4DCT studies. For 253 cardiac 4DCT studies, 6 VR videos depicting the LV were automatically generated corresponding to views rotated every 60 degrees around the long axis. Ground-truth WMA classification was performed for each video of the LV views by evaluating the extent of impaired regional shortening that was visible in that view. For DL prediction, videos were first processed by a pre-trained CNN "Inception V3" to extract image features. Then, extracted features from multiple frames were concatenated into a matrix and then input into a long short-term memory for the binary classification of WMA presence in the video. Studies were classified as abnormal if >= 2 out of 6 videos were abnormal. Studies were split chronologically so that the first 174 patients were used in 5-fold cross-validation and the final 79 studies were used in testing. VR significantly compressed data size (similar to 800-fold). DL classification of WMA had high (>=89%) per-video and perstudy accuracy, sensitivity, and specificity for both cross-validation and testing cohorts. This novel method may offer a simple and accurate way to screen CT cases for WMA from highly compressed data.
更多查看译文
关键词
X-ray computed tomography, Cardiac imaging, LV wall motion abnormality, Volume Rendering, Deep Learning
AI 理解论文
溯源树
样例
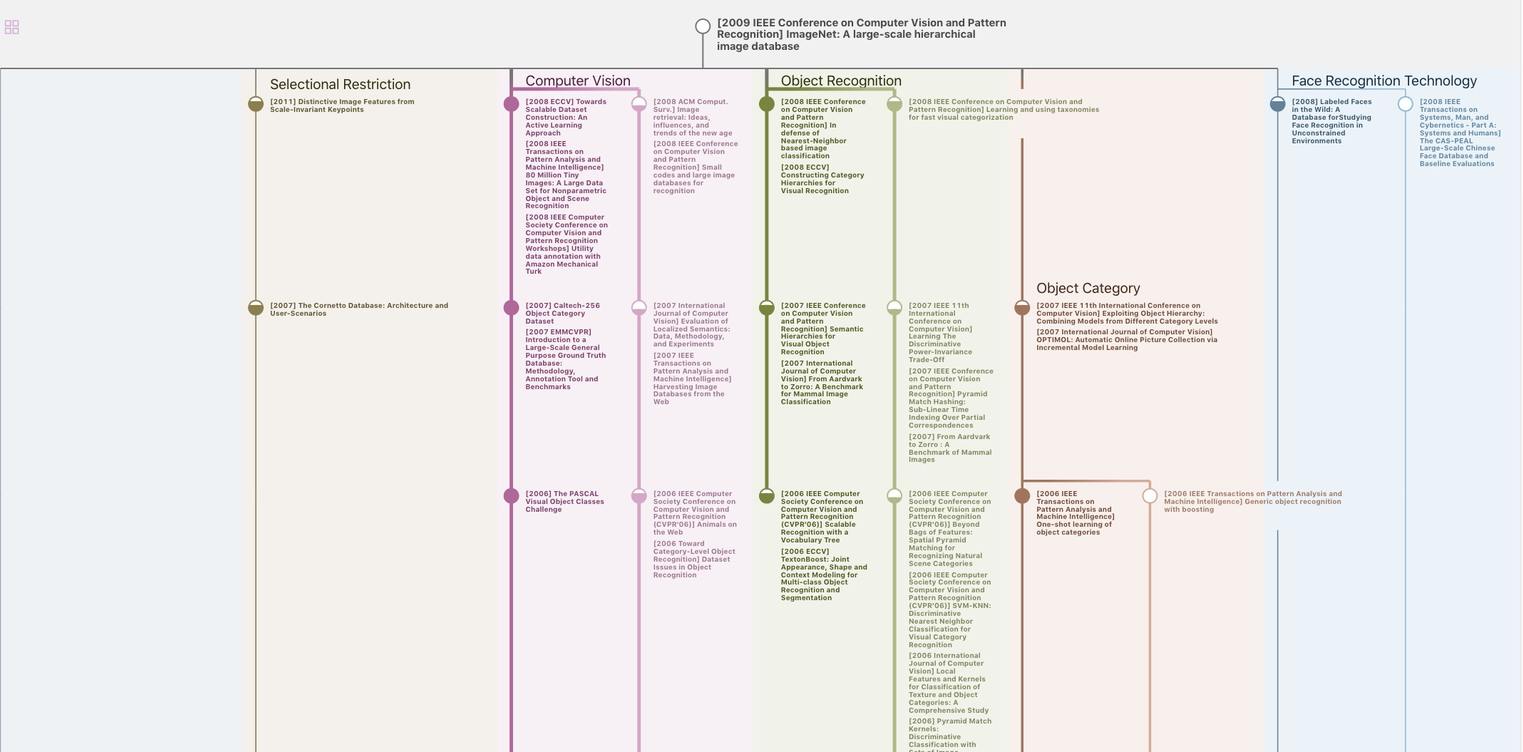
生成溯源树,研究论文发展脉络
Chat Paper
正在生成论文摘要