Sequestration of Imaging Studies in MIDRC: a Multi-Institutional Data Commons
MEDICAL IMAGING 2022: IMAGE PERCEPTION, OBSERVER PERFORMANCE, AND TECHNOLOGY ASSESSMENT(2022)
摘要
The Medical Imaging and Data Resource Center (MIDRC) is a multi-institutional effort to accelerate medical imaging machine intelligence research and create a publicly available image repository/commons as well as a sequestered database for performance evaluation and benchmarking of algorithms. After de-identification, approximately 80% of the medical images and associated meta-data will become part of the open repository and 20% will be sequestered and kept separate from the open commons. To ensure that both the public, open dataset and the sequestered dataset are representative of the population available, demographic characteristics across the two datasets must be balanced. Our method uses multidimensional stratified sampling where several demographic variables of interest are sequentially used to separate the data into individual strata, each representing a unique combination of variables. Within each stratum, patients are randomly assigned to the open set (80%) or the sequestered set (20%). Thus, for p variables of interest, the balance of the p dimensional distribution of variable combinations can be controlled. This algorithm was used on an example COVID-19 dataset containing image exams of 4662 patients using the variables of race, age, sex at birth, and ethnicity, each containing 8, 8, 2, and 4 categories, respectively. After stratification of this dataset into the two subsets, resulting distributions of each variable matched the distribution from the original dataset with a maximum percent difference from its original fraction of 0.4%. These results demonstrate that the implemented process of multi-dimensional sequential stratified sampling can partition a large database while maintaining balance across several variables.
更多查看译文
关键词
Machine learning,stratified sampling,sequestration,COVID-19,image database,algorithm performance,MIDRC
AI 理解论文
溯源树
样例
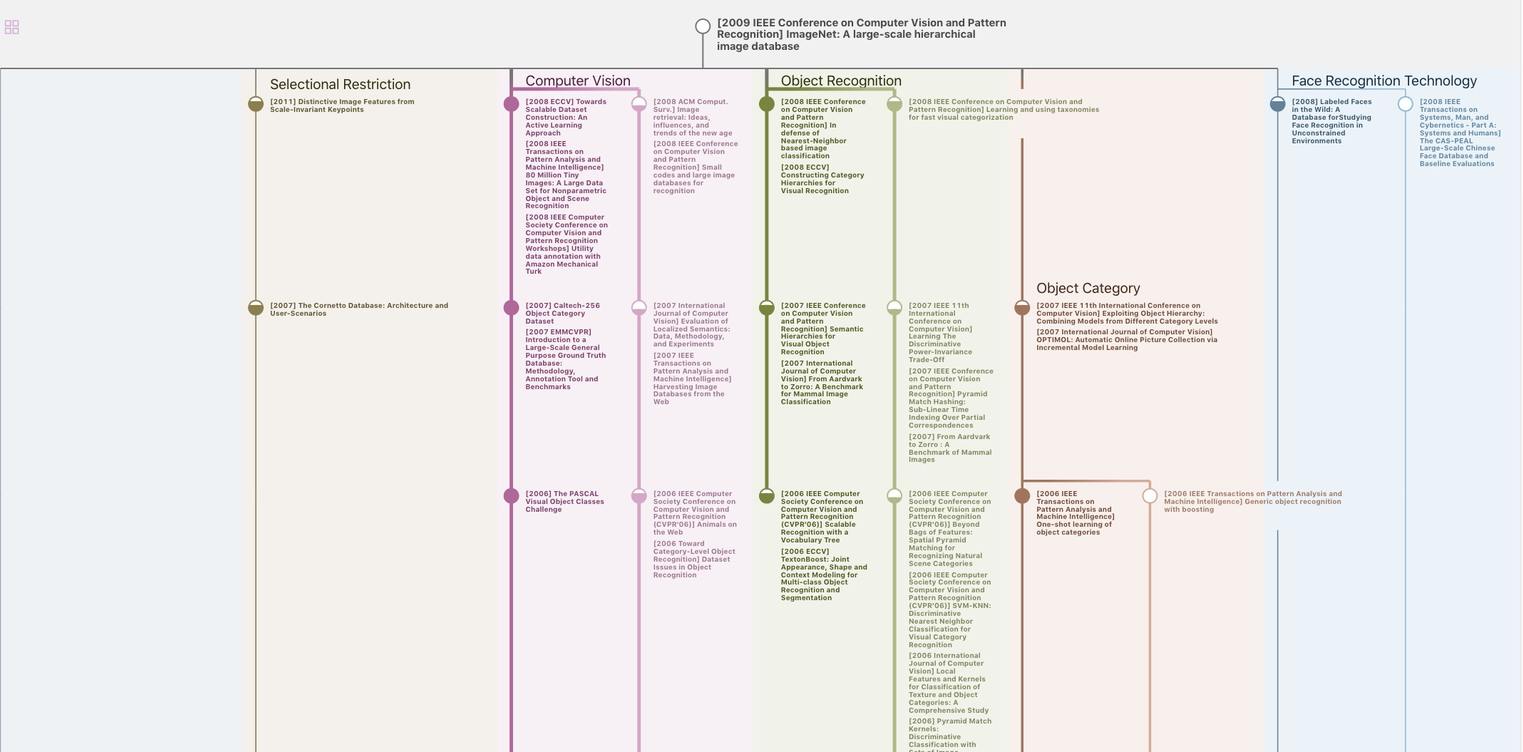
生成溯源树,研究论文发展脉络
Chat Paper
正在生成论文摘要