Multi-task Deep Learning for Segmentation and Landmark Detection in Obstetric Sonography
MEDICAL IMAGING 2022: IMAGE-GUIDED PROCEDURES, ROBOTIC INTERVENTIONS, AND MODELING(2022)
摘要
The utilization of point-of-care ultrasound (POCUS) has been on rise in recent years, followed by a growing need for comprehensive, compact obstetrics analysis software systems. The accurate computerized assessment of obstetric ultrasound (US) is a challenging task due to the noisy nature of US images and presence of complex anatomies. In this work, we propose a multi-branch deep learning architecture to identify multiple anatomies in obstetric sonography through segmentation and landmark detection. The multi-task deep model is trained to segment the uterus and gestational sac regions, and to localize the position of landmark points denoting the crown and rump of the fetus. We conduct experiments with varying sizes of models, presenting a trade-off between accuracy and efficiency. Our larger models reach average Dice scores of 90% and 91% for segmenting uterus and gestational sac, respectively, and have 1.64 mm average length error for the fetus crown-rump length (CRL) measurement. Furthermore, we present choices for smaller model sizes suitable for integration in portable POCUS devices with limited computing capacities. The smallest model with the most efficient run-time has only about 167.3k parameters, where compared to the larger models, the Dice score is reduced by 4.1% and 6.7% for uterus and gestational sac segmentation, respectively, and the CRL length error is increased by 0.42 mm. This is while the smallest model has 98.8% reduced model size and could smoothly run on naive mobile devices.
更多查看译文
关键词
Multi-task Learning,Segmentation,Landmark Detection,Deep Learning,Obstetric Ultrasound
AI 理解论文
溯源树
样例
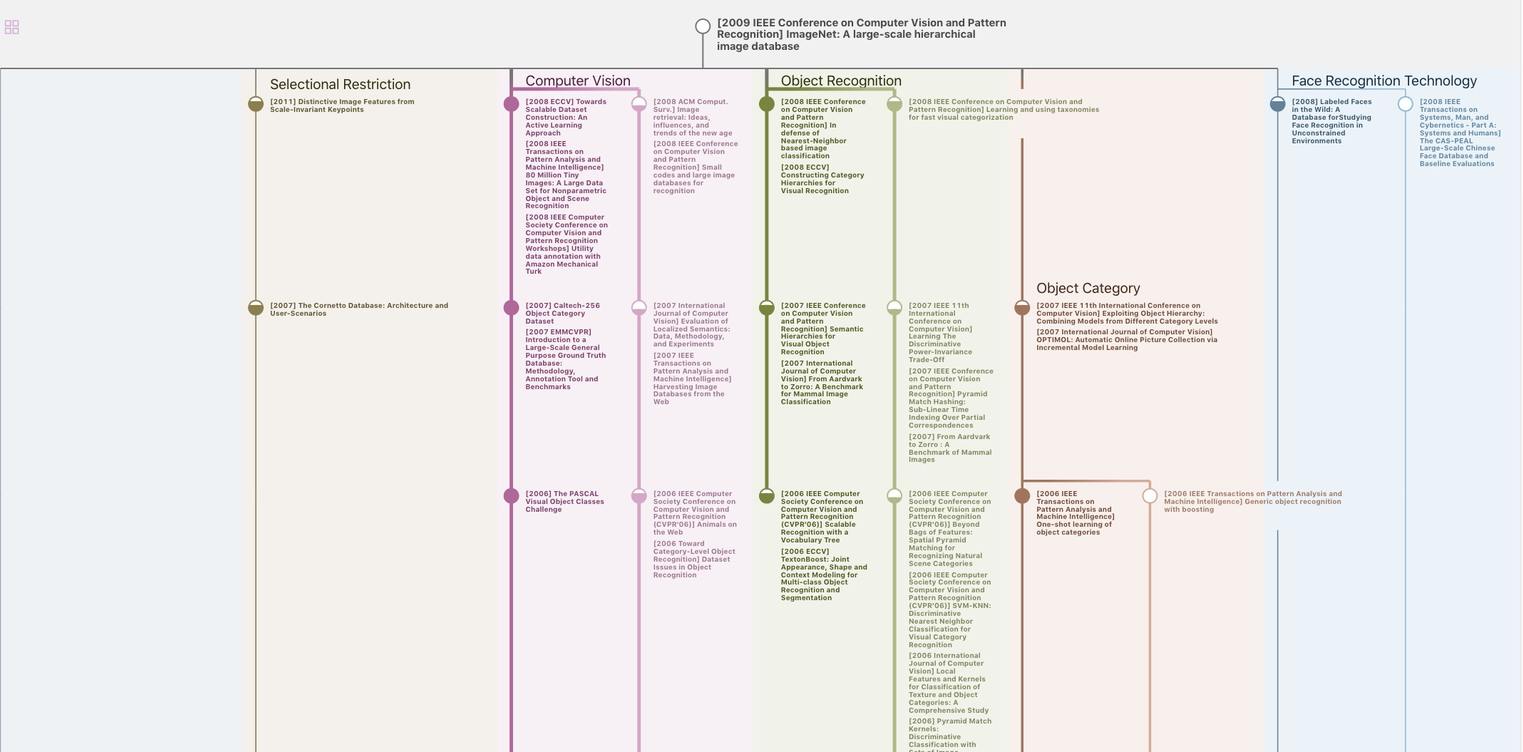
生成溯源树,研究论文发展脉络
Chat Paper
正在生成论文摘要