AiEEG: Personalized Seizure Prediction Through Partially-Reconfigurable Deep Neural Networks
2022 IEEE International Conference on Pervasive Computing and Communications (PerCom)(2022)
摘要
With more than 65M people affected by epilepsy worldwide, early prediction and response to seizure onsets have become more important than ever. Cutting-edge research in implantable medical devices (IMDs) has shown that deep neural networks (DNNs) applied to intracranial electroencephalogram (iEEG) data can predict seizures up to an hour before onset. However, offloading of iEEG data to the edge/cloud is highly prohibitive, due to the sheer size of the generated data. Existing work either focuses on the DNN training phase only, or does not consider the severe energy/space limitations of IMDs. Moreover, the technical aspects of patient personalization, which allows for patient-specific hyper-parameter tuning, still remain unaddressed. In this paper, we propose a platform called AiEEG for in vivo early seizure prediction, whose DNN hardware circuitry can be reconfigured remotely without surgery. We prototype AiEEG on a system on chip (SoC) platform and demonstrate its end-to-end capabilities in seizure prediction with a population of 30 epileptic patients, with iEEG signals coming from a real-world dataset. Extensive experimental results shows that (i) our embedded and personalized DNN has an area under the curve (AUC) averaging at 0.97 and as low as zero false positives per hour (FPH) for over half the patients, an improvement of about 3.5x with respect to a non-personalized prediction method and the best for a dataset of this size when compared to the state-of-the-art; (ii) our AiEEG platform consumes 4.2x less energy than a cloud-based approach, leading to a 4x battery lifetime improvement; (iii) we are able to remotely fine-tune the DNN through partial reconfiguration as needed in about 10s.
更多查看译文
关键词
Ultrasound,Wireless,IoT,Deep Learning,Seizure Prediction
AI 理解论文
溯源树
样例
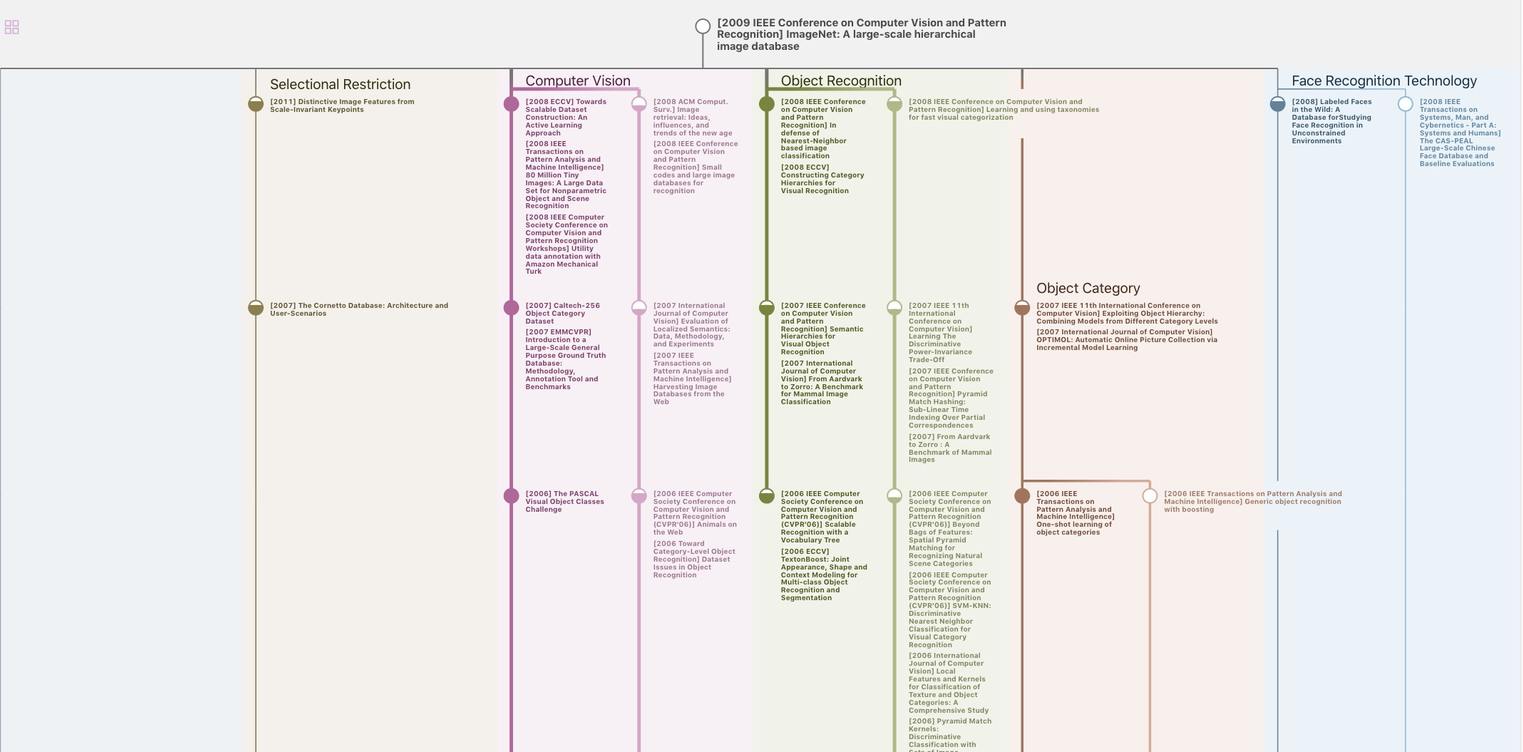
生成溯源树,研究论文发展脉络
Chat Paper
正在生成论文摘要