Breast Mass Segmentation Based on U-Net++ and Adversarial Learning Network
LASER & OPTOELECTRONICS PROGRESS(2022)
Abstract
In this paper, an accurate and reliable breast lesion segmentation algorithm is examined to extract tumor regions from mammographic images for the diagnosis of breast diseases. Additionally, a framework incorporating an adversarial network, which is mainly composed of a segmentation network and a discriminant network, is used for the enhancement of the high-order consistency of the segmentation results. Here, an improved U-Net++ network is used as the segmentation network to generate a breast mass segmentation map (a mask), while the discriminant network is used to discriminate between the generated mask and the real mask to further enhance the performance of the segmentation network. The performance of the proposed method is verified on the public dataset (CBIS-DDSM). The experimental results show that the specificity, sensitivity, accuracy, and Dice coefficient of the proposed method are 99. 7%, 90. 4%, 98%, and 91%, respectively, which are higher than that of the classical algorithms. The deep learning algorithm combined with the improved model (U-Net++) and generated countermeasure network can improve the segmentation performance of breast mass in molybdenum target images.
MoreTranslated text
Key words
breast mass segmentation, deep learning, U-Net++ network, adversarial learning
AI Read Science
Must-Reading Tree
Example
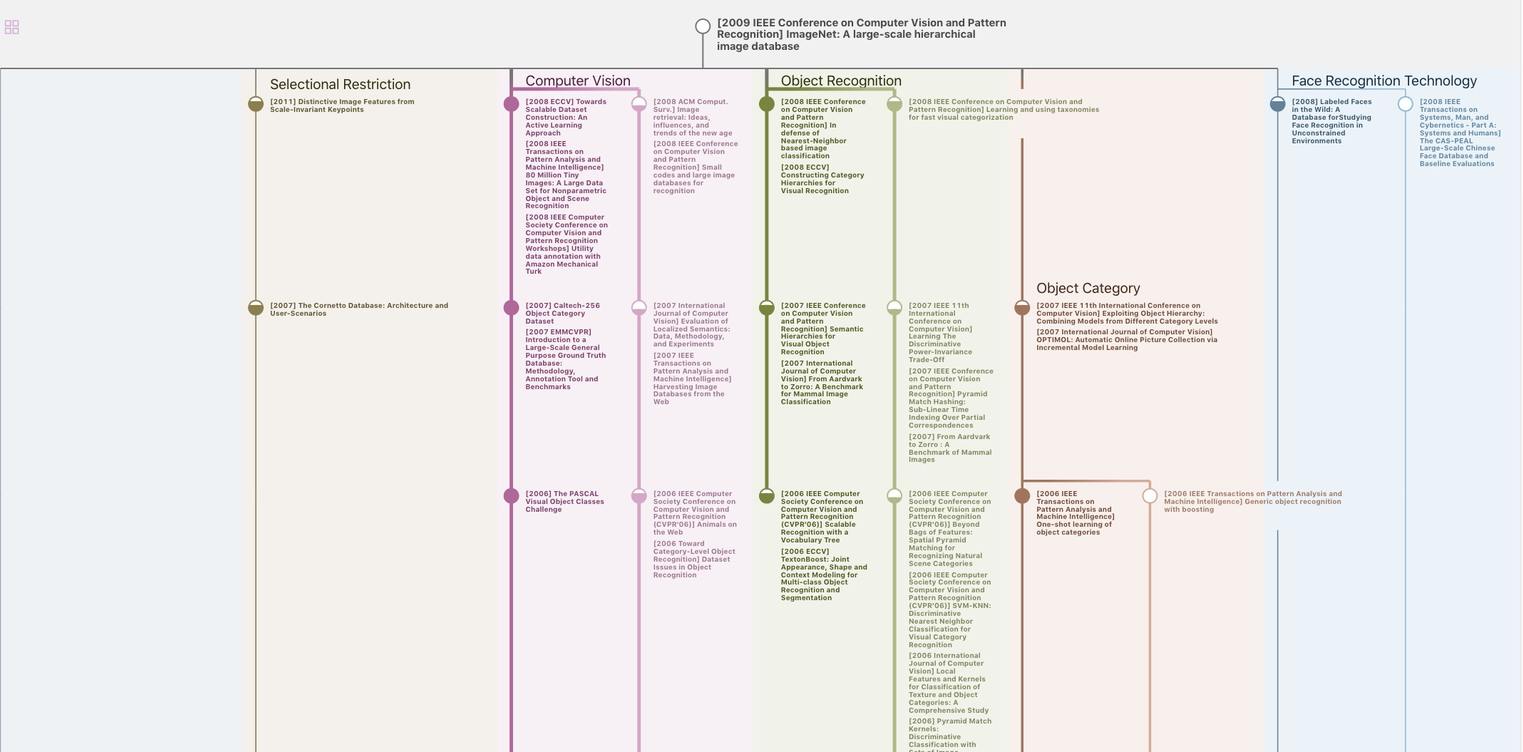
Generate MRT to find the research sequence of this paper
Chat Paper
Summary is being generated by the instructions you defined